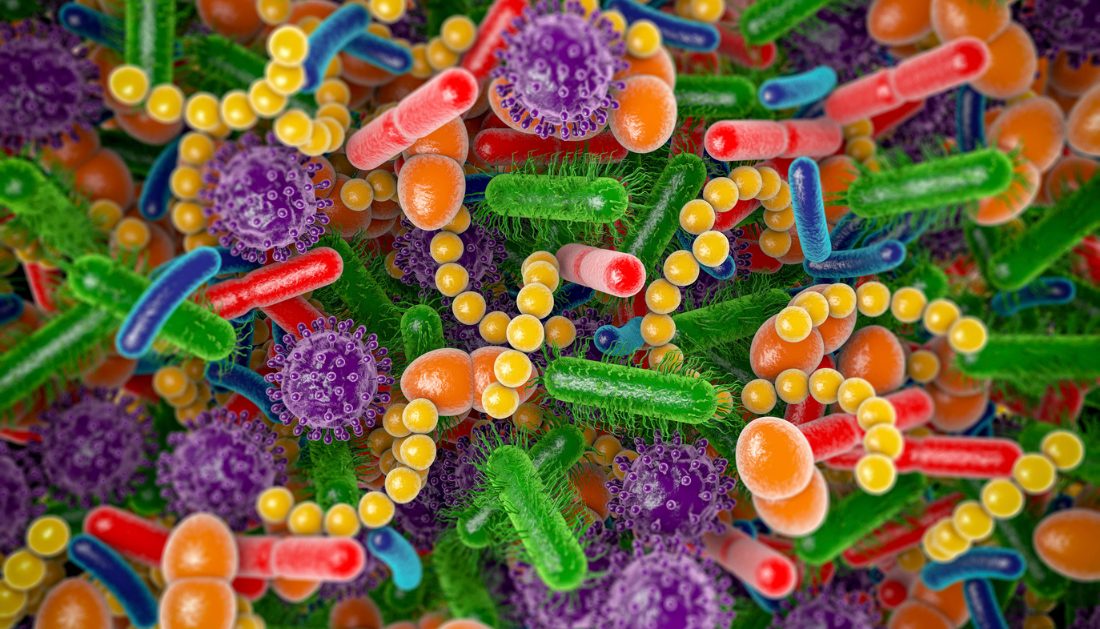
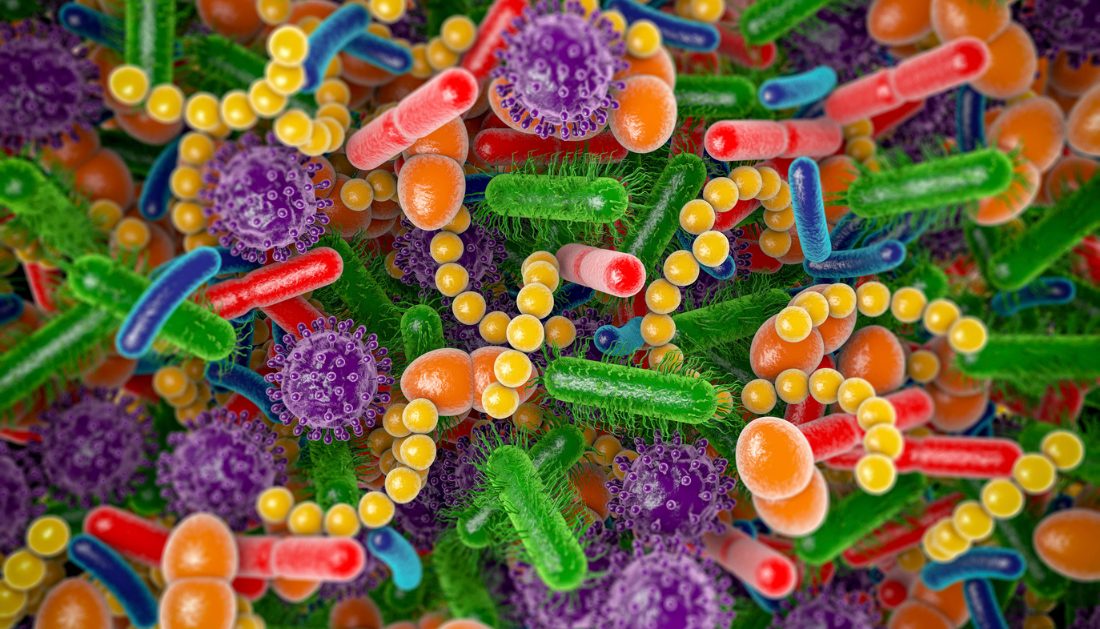
In a recent pre-print study published on bioRxiv*, a team of researchers investigated the predictive role of gut microbiome composition during acute Severe Acute Respiratory Syndrome Coronavirus 2 (SARS-CoV-2) infection in the development of Long Coronavirus Disease (LC), as well as its relationship with clinical variables and symptom clusters.
Background
LC affects 10-30% of non-hospitalized patients infected with SARS-CoV-2, resulting in severe morbidity, job loss, and a $3.7 trillion economic burden in the US.
Symptoms include cardiovascular, gastrointestinal, cognitive, and neurological difficulties, similar to myalgic encephalomyelitis and other post-infectious disorders. Proposed explanations include immunological dysregulation, neuroinflammation, viral persistence, and coagulation problems, with new evidence linking the gut microbiota to LC pathogenesis.
Current research focuses on hospitalized patients, which limits generalizability to milder situations. More study is needed to investigate microbiome-driven variables in outpatient populations, allowing for tailored diagnosis and therapeutics for LC’s diverse and complex presentation.
About the study
The Mayo Clinic Institutional Review Board approved the study, which enrolled persons aged 18 and older who had SARS-CoV-2 testing at Mayo Clinic facilities in Minnesota, Florida, and Arizona between October 2020 and September 2021. Participants were discovered using electronic health record (EHR) evaluations that were filtered for SARS-CoV-2 testing schedules.
Eligible individuals were contacted via email, and their informed consent was obtained. Of the 1,061 people initially recruited, 242 were eliminated due to insufficient data, faulty sequencing, or other difficulties. The final group consisted of 799 participants (380 SARS-CoV-2 positive and 419 SARS-CoV-2 negative), who provided 947 stool samples.
Stool samples were taken at two intervals: weeks 0-2 and weeks 3-5 following testing. Samples were transported in frozen gel packs via overnight courier and stored at −80°C for further analysis. Qiagen kits were used to extract microbial deoxyribonucleic acid (DNA), and metagenomic sequencing was performed with a target of 8 million reads per sample.
The Human Microbiome Project Unified Metabolic Analysis Network (HUMAnN3) was used to do functional profiling, while Kraken2 was used for taxonomic profiling.
Calprotectin levels in the stool were assessed using an enzyme-linked immunosorbent assay (ELISA), and SARS-CoV-2 RNA was identified using reverse transcription-quantitative polymerase chain reaction (RT-qPCR).
Clinical data, such as demographics, comorbidities, medications, and symptom persistence, were retrieved from electronic health records.
Machine learning models including microbiome and clinical data were used to predict LC and identify symptom clusters, revealing important information about the condition’s variability.
Study results
The study examined 947 stool samples taken from 799 patients, including 380 SARS-CoV-2 positives and 419 negative controls. 80 individuals in the SARS-CoV-2-positive group developed LC during a one-year follow-up period.
Participants were divided into three groups for analysis: LC, non-LC (SARS-CoV-2 positive without LC), and SARS-CoV-2 negative. Baseline parameters revealed substantial disparities between these groups. LC individuals were primarily female and had more baseline comorbidities than non-LC participants.
The SARS-CoV-2-negative group was older and more likely to have had antibiotics and vaccinations. These variables were controlled for in future studies.
During acute infection, gut microbiome diversity varied dramatically across groups. SARS-CoV-2-positive participants (LC and non-LC) had decreased alpha diversity compared to SARS-CoV-2-negative persons.
Beta diversity studies indicated different microbial compositions amongst the groups, with LC patients having distinct microbiome profiles during acute illness.
Certain bacterial taxa, such as Faecalimonas and Blautia, were more abundant in LC patients, whereas other taxa were more prevalent in non-LC and negative individuals. These data suggest that the gut microbiome makeup during an acute infection may be a predictor of LC.
Temporal examination of gut microbiota alterations during the acute and post-acute stages revealed significant individual heterogeneity but no cohort-level differences, implying that temporal changes do not contribute to LC formation.
However, machine learning algorithms indicated that microbiome data collected during acute infection, when paired with clinical factors, accurately predicted LC. Microbial predictors, including species from the Lachnospiraceae family, had a substantial impact on model performance.
Symptom study found that LC comprises a variety of clinical manifestations. Fatigue was the most common symptom, followed by dyspnea and a cough.
Cluster analysis revealed four distinct LC subphenotypes based on symptom co-occurrence: gastrointestinal and sensory, musculoskeletal and neuropsychiatric, cardiopulmonary, and fatigue-only.
Each cluster displayed distinct microbial relationships, with the gastrointestinal and sensory clusters exhibiting the most dramatic microbial changes. Notably, organisms from the Lachnospiraceae and Erysipelotrichaceae families were overrepresented in this cluster.
Conclusions
To summarize, this study found that SARS-CoV-2-positive individuals who developed LC had unique gut microbiota patterns during acute infection. While previous study has linked the gut microbiome to COVID-19 results, few studies have looked at its prognostic value for LC, particularly in outpatient groups.
Using machine learning methods such as artificial neural networks and logistic regression, this study discovered that microbiome data predicted LC more precisely than clinical characteristics including disease severity, gender, and immunization status.
The Lachnospiraceae family, including Eubacterium and Agathobacter, as well as Prevotella spp., made significant contributions. These findings emphasize the gut microbiome’s potential as a diagnostic tool for determining LC risk, allowing for more customized therapies.
For more information: Isin Y. Comba, Ruben A. T. Mars, Lu Yang, et al. (2024) Gut Microbiome Signatures During Acute Infection Predict Long COVID, bioRxiv. doi:https://doi.org/10.1101/2024.12.10.626852. https://www.biorxiv.org/content/10.1101/2024.12.10.626852v1.full
more recommended stories
PFAS Exposure Linked to Increased Type 2 Diabetes Risk
Investigating the Metabolic Impact of Forever.
Can Routine Vaccines Help Combat Antibiotic Resistance?
A growing body of evidence now.
T-bet and the Genetic Control of Memory B Cell Differentiation
In a major advancement in immunology,.
Brain’s Biological Age Emerges as Key Health Risk Indicator
Clinical Significance of Brain Age in.
Children’s Health in the United States is Declining!
Summary: A comprehensive analysis of U.S..
Emotional Processing for Chronic Pain Relief
A groundbreaking clinical trial led by.
Egg White Proteins for Hypertension
Egg white proteins for hypertension have.
Limited Health Care Access During Disasters Delays Colorectal Cancer Diagnoses
Natural disasters like Hurricanes Irma and.
Ethical Issues in Human-AI Relationships
As artificial intelligence becomes increasingly lifelike.
Vegetarian Diets and Healthy Aging: Does Diet Quality Make a Difference?
Vegetarian diets are widely recognized for.
Leave a Comment