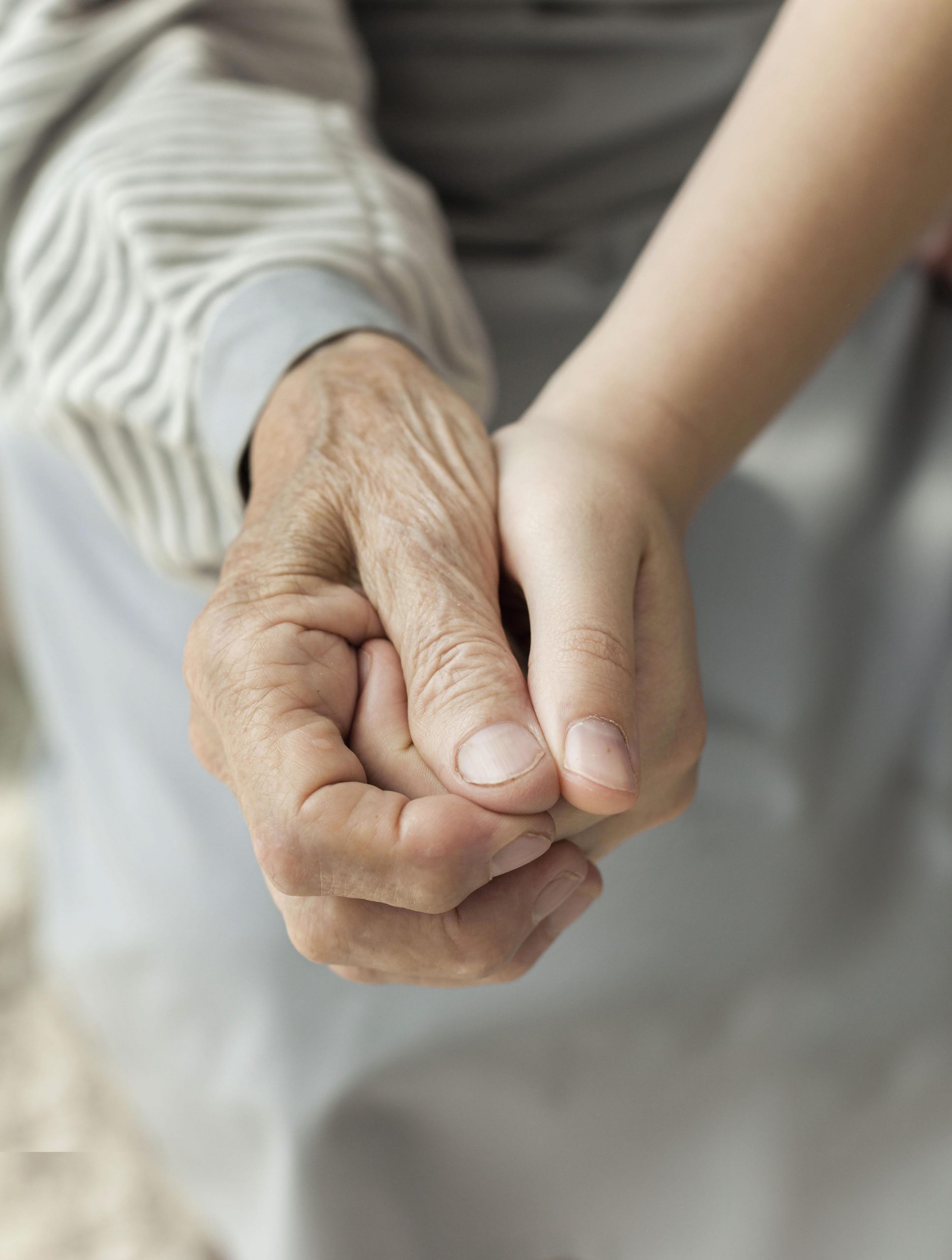
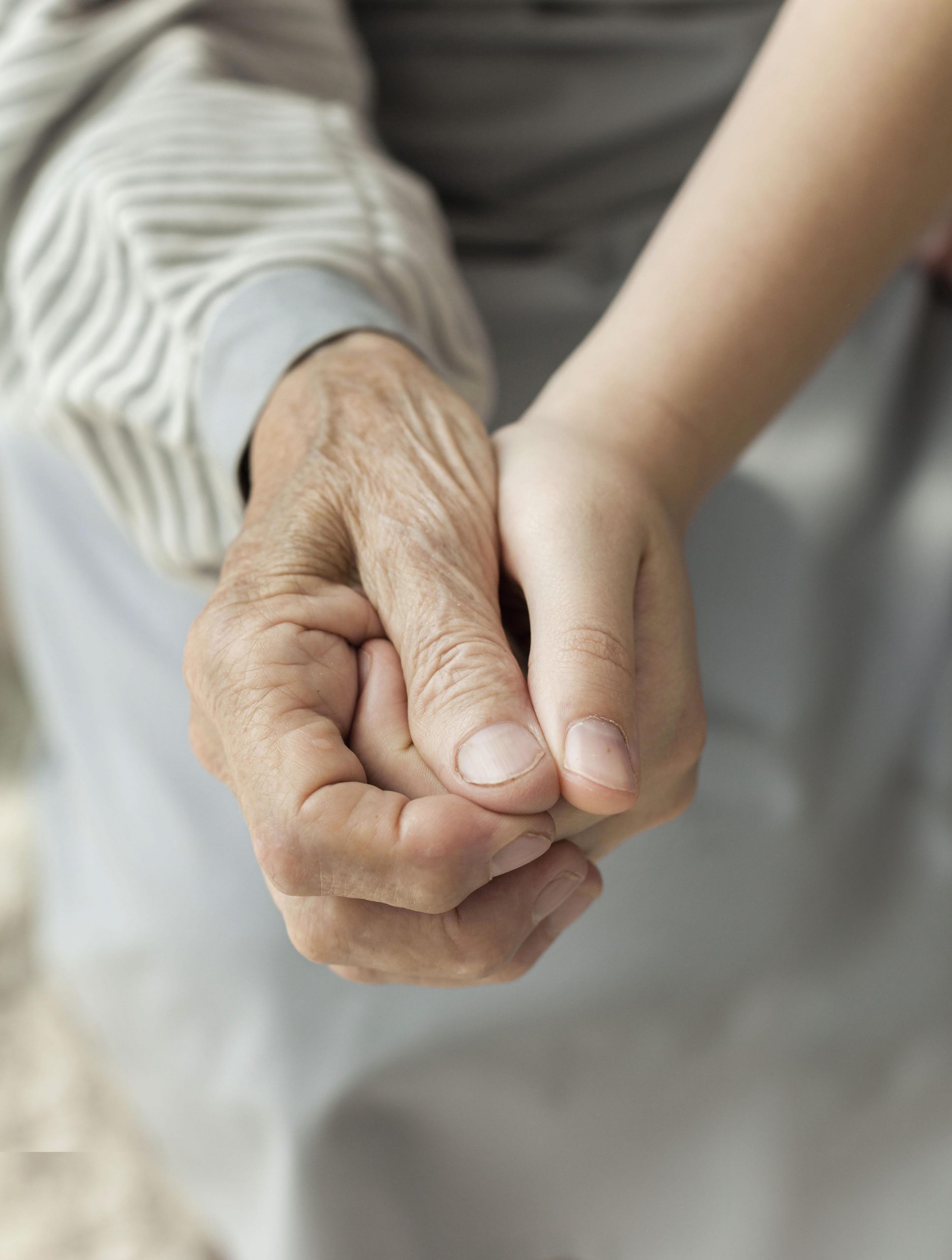
A method created by researchers from UNSW Sydney and Boston University shows early promise for diagnosing Parkinson’s disease years before the first symptoms arise.
The study, which was just published in the journal ACS Central Science, detailed the researchers’ use of neural networks to examine biomarkers in patients’ bodily fluids. The Spanish European Prospective Investigation into Cancer and Nutrition (EPIC) collected blood samples from healthy individuals, which the researchers from UNSW School of Chemistry studied. The team trained their machine learning algorithm over datasets with significant information about metabolites—the chemical compounds that the body produces while breaking down food, pharmaceuticals, or chemicals—with a focus on 39 people who got Parkinson’s up to 15 years later.
The researchers was able to uncover particular combinations of metabolites that could prevent or possibly be early warning signs for Parkinson’s after contrasting these metabolites to those of 39 matched control individuals from the same trial who did not go on to develop Parkinson’s.
CRANK-MS, which stands for Classification and Ranking Analysis using Neural network produces Knowledge from Mass Spectrometry, is a machine learning method that was created by UNSW researcher Diana Zhang and Associate Professor W. Alexander Donald.
The most common method of analyzing metabolomics data is through statistical approaches,” says Zhang.
“So to figure out which metabolites are more significant for the disease versus control groups, researchers usually look at correlations involving specific molecules.”
“But here we take into account that metabolites can have associations with other metabolites—which is where the machine learning comes in. With hundreds to thousands of metabolites, we’ve used computational power to understand what’s going on.”
A/Prof. Donald says that in addition to looking at combinations of metabolites, the researchers used an unedited list of data.
“Typically, researchers using machine learning to examine correlations between metabolites and disease reduce the number of chemical features first, before they feed it into the algorithm,” he says.
“But here we feed all the information into CRANK-MS without any data reduction right at the start. And from that, we can get the model prediction and identify which metabolites are driving the prediction the most, all in one step. It means that if there are metabolites which may potentially have been missed using conventional approaches, we can now pick those up.”
Parkinson’s disease is now identified through the observation of physical symptoms like a resting hand tremor. For non-genetic cases of it, there is no blood or laboratory testing available for diagnosis. However, decades before the motor symptoms appear, atypical symptoms including insomnia and apathy can appear in persons with Parkinson’s disease. Therefore, CRANK-MS could be used to rule in or rule out the risk of acquiring Parkinson’s in the future at the first manifestation of these atypical symptoms.
A/Prof Donald emphasizes that before the instrument might be used consistently, validation studies utilizing significantly bigger cohorts and carried out in numerous locations throughout the world are required. However, in the small sample investigated for this study, the results were encouraging, with CRANK-MS able to analyze molecules found in blood to accurately diagnose Parkinson’s disease in up to 96 percent of cases.
“This study is interesting at multiple levels,” he says.
First, the accuracy is very high for predicting Parkinson’s disease in advance of clinical diagnosis. Second, this machine learning approach enabled us to identify chemical markers that are the most important in accurately predicting who will develop Parkinson’s disease in the future. Third, some of the chemical markers that drive accurate prediction the most have been previously implicated by others to Parkinson’s disease in cell-based assays but not in humans.”
Analyzing the metabolites of research participants who later had Parkinson’s revealed some intriguing results.
For instance, compared to those who did not eventually acquire Parkinson’s disease, those who did had lower quantities of triterpenoids in their blood. Triterpenoids, a well-known neuroprotectant that controls oxidative stress, are frequently present in foods including tomatoes, apples, and olives. If consuming these foods can naturally prevent against getting Parkinson’s disease, that could be the subject of a future study.
The discovery of polyfluorinated alkyl compounds (PFAS), which may be related to exposure to industrial chemicals, in Parkinson’s patients was also worthy of further investigation.
“We have evidence to suggest that it is PFAS, but we need more characterization data to be 100 percent sure,” says A/Prof Donald.
Researchers can use CRANK-MS, a publically accessible tool, to use machine learning for metabolomics data-based disease diagnosis.
“We’ve built the model in such a way that it’s fit for purpose,” says Zhang.
“The application of CRANK-MS to detect Parkinson’s disease is just one example of how AI can improve the way we diagnose and monitor diseases. What’s exciting is that CRANK-MS can be readily applied to other diseases to identify new biomarkers of interest.”
“The tool is user-friendly where on average, results can be generated in less than 10 minutes on a conventional laptop.”
more recommended stories
Human Cell Atlas: Mapping Biology for Precision Medicine
In a recent perspective article published.
Preterm Birth Linked to Higher Mortality Risk
A new study from Wake Forest.
Heart Failure Risk Related to Obesity reduced by Tirzepatide
Tirzepatide, a weight-loss and diabetes medicine,.
Antibiotic Activity Altered by Nanoplastics
Antibiotic adsorption on micro- and nano-plastics.
Cocoa Flavonols: Combat Stress & Boost Vascular Health
Cocoa Flavonols on combatting Stress: Stress.
AI Predicts Triple-Negative Breast Cancer Prognosis
Researchers at Sweden’s Karolinska Institutet explored.
Music Therapy: A Breakthrough in Dementia Care?
‘Severe’ or ‘advanced’ dementia is a.
FasL Inhibitor Asunercept Speeds COVID-19 Recovery
A new clinical trial demonstrates that.
Gut Health and Disease is related to microbial load
When it comes to Gut Health,.
Camel vs Cow vs Goat Milk: Best for Diabetes
In a recent review published in.
Leave a Comment