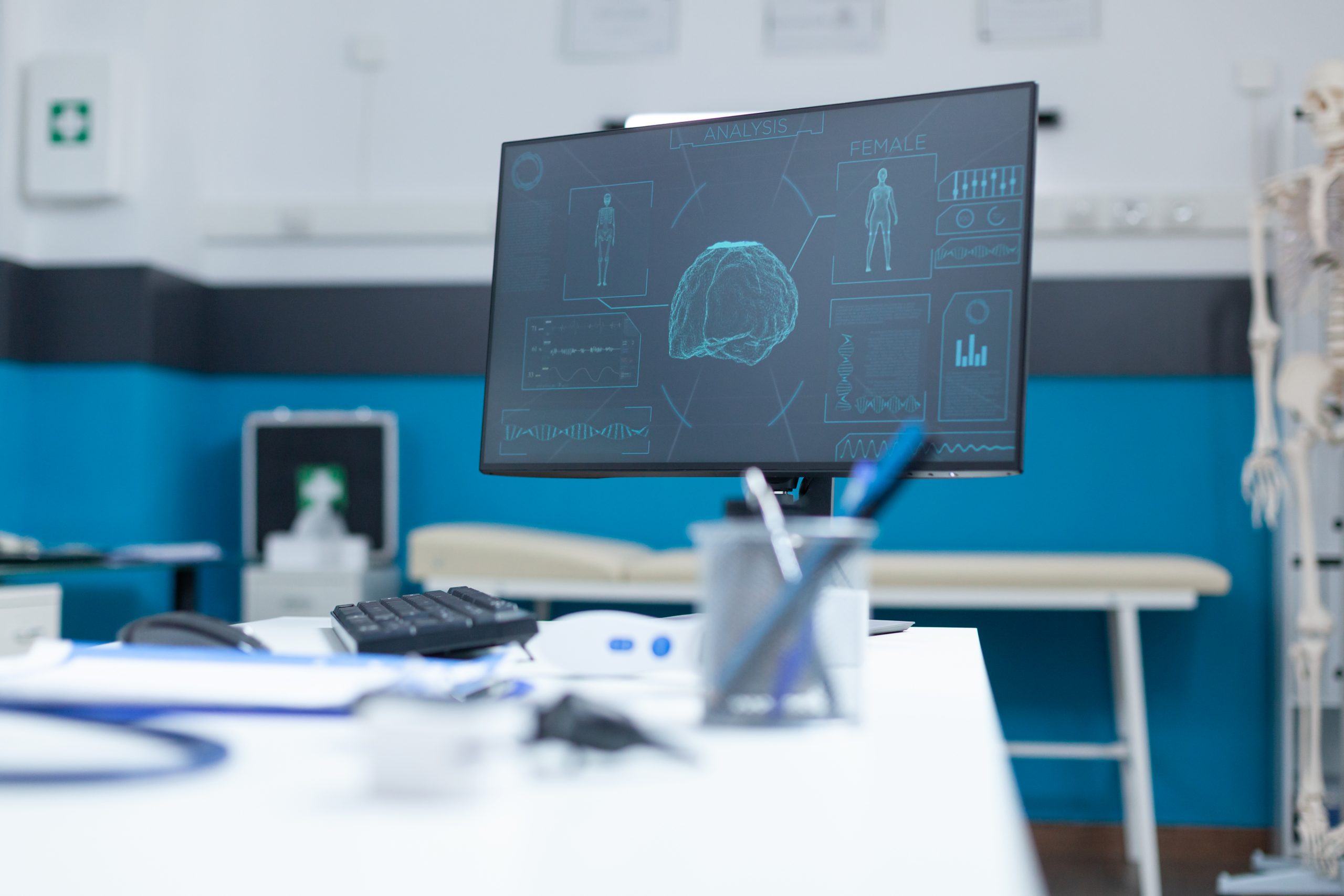
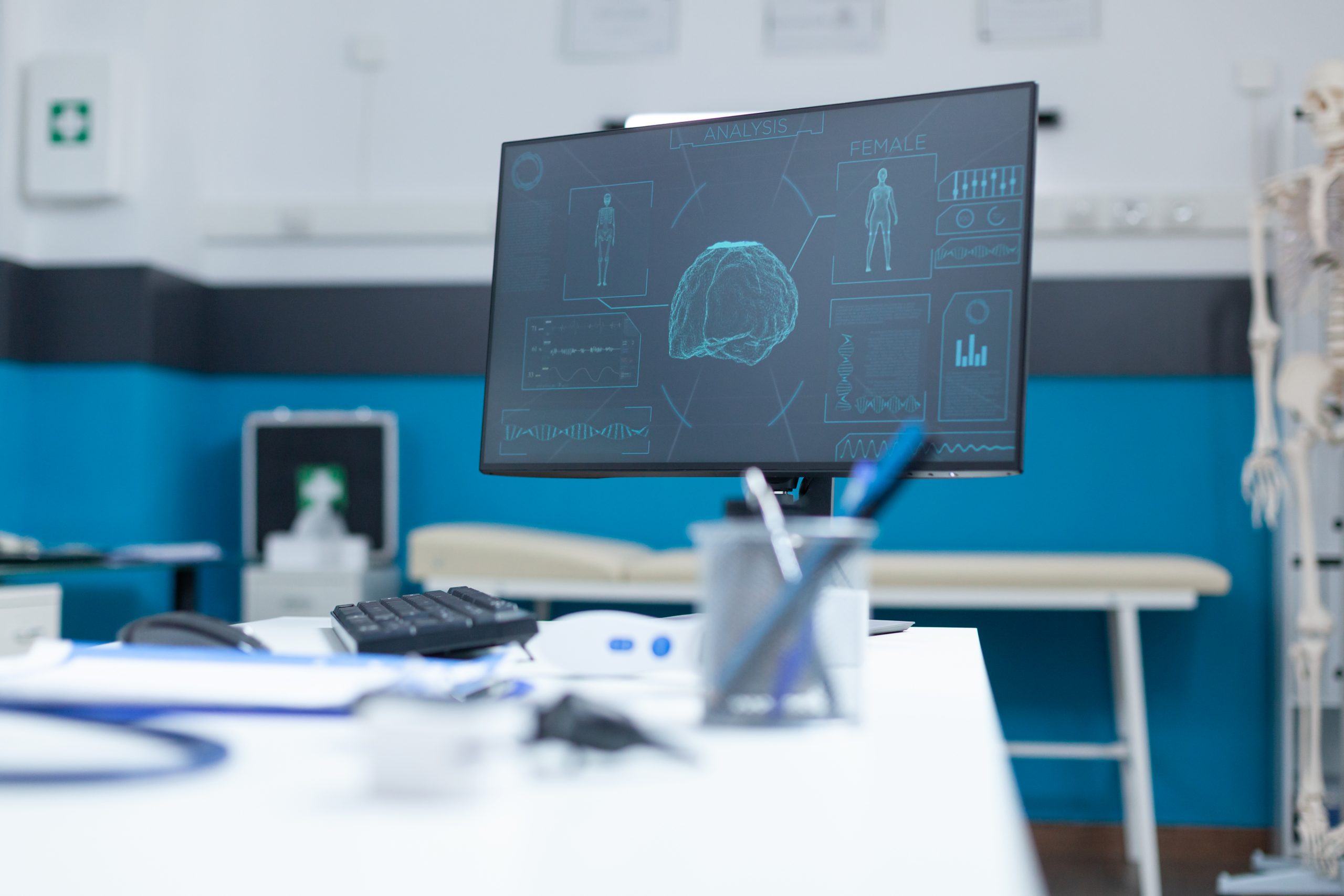
According to the US Centers for Disease Control and Prevention, hypertension is a possible death sentence for about half of all Americans, accounting for nearly 700,000 deaths in 2021. It also raises the chances of having a stroke or developing persistent heart failure. However, while it is generally simple to prevent or control if detected early—eat healthily, move more, and drink less—it can be difficult to manage. Although physicians have a plethora of potential hypertension drugs to choose from, each has pros and cons that make selecting the most effective one difficult: Beta-blockers reduce heart rate but can cause asthma; ACE inhibitors relax blood vessels but can produce a hacking cough. A new artificial intelligence tool may now assist doctors in better matching the appropriate drugs to the appropriate patients.
The data-driven model, co-developed by Boston University data scientists and physicians, aims to give clinicians real-time hypertension treatment recommendations based on patient-specific characteristics, including demographics, vital signs, past medical history, and clinical test records. The model, described in a recent study published in BMC Medical Informatics and Decision Making, has the potential to help reduce systolic blood pressure—measured when the heart is beating rather than resting—more effectively than the current standard of care. The program’s commitment to transparency, according to the researchers, could also assist boost physicians’ trust in AI-generated outcomes.
“This is a new machine learning algorithm leveraging information in electronic health records and showcasing the power of AI in healthcare,” says Ioannis Paschalidis, a BU College of Engineering distinguished professor and director of the Rafik B. Hariri Institute for Computing and Computational Science & Engineering. “Our data-driven model is not just predicting an outcome, it is suggesting the most appropriate medication to use for each patient.”
Currently, a doctor examines the patient’s history, treatment goals, and the advantages and hazards associated with certain medications before deciding which medication to give. Choosing which treatment to administer when there are numerous options—and neither drug is better or worse than the other—can often be a coin toss.
The BU-developed algorithm, on the other hand, develops a unique hypertension prescription based on an individual patient’s profile, providing clinicians with a list of proposed drugs and an associated probability of success. The researchers’ goal was to identify the medication that best reduces systolic blood pressure for each patient based on its effectiveness in a group of individuals with comparable symptoms.
“Our goal is to facilitate a personalization approach for hypertension treatment based on machine learning algorithms,” says Paschalidis, “seeking to maximize the effectiveness of hypertensive medications at the individual level.”
The model was created using deidentified data acquired between 2012 and 2020 from 42,752 hypertensive patients at Boston Medical Center (BMC), BU’s principal teaching hospital. Patients were assigned to affinity groups based on clinically relevant variables such as demographics, previous blood pressure data, and medical history. The model’s effectiveness was compared to the current standard of care, as well as three other algorithms attempting to predict optimal treatment options, during the study. The researchers discovered that it reduced systolic blood pressure by 70.3% more than conventional treatment and performed 7.08% better than the second-best model. The system was clinically validated by analyzing a random sample of 350 cases by hand.
The model also demonstrated the advantages of deprescribing—reducing or discontinuing prescriptions for some individuals who take numerous drugs. According to the research team, because the algorithm suggests various appropriate medicines to physicians, it could provide significant insights when the medical community is divided on the efficacy of one drug vs another, a scenario known as clinical equipoise.
These advanced predictive analytics have the ability to augment a clinician’s decisionmaking and to have a positive impact on the quality of care we deliver, and therefore the outcomes for our patients,” says Rebecca Mishuris, who previously taught at BU and recently became Mass General Brigham’s chief medical information officer. “This is an important first step that shows that these models actually perform better than standard of care, and could help us be better doctors.”
While many acknowledge that machine learning’s ability to manage massive amounts of data and identify patterns and connections could assist healthcare, its adoption has been limited, owing in part to challenges comprehending the results—as well as low levels of trust in artificial intelligence. Machine learning in healthcare has been limited in the past by inadequate or erroneous data, as well as sparse patient histories, which can distort prediction results. A critical feature of this study was ensuring that data was visible and that doctors, particularly those without technical ability, understood how the algorithm operated and why the model offered various therapy options.
more recommended stories
Reelin and Cocaine Addiction: A Breakthrough Study
A groundbreaking study from the University.
Preeclampsia and Stroke Risk: Long-Term Effects
Preeclampsia (PE) – a hypertensive disorder.
Statins and Depression: No Added Benefit
What Are Statins Used For? Statins.
Azithromycin Resistance Rises After Mass Treatment
Mass drug administration (MDA) of azithromycin.
Generative AI in Health Campaigns: A Game-Changer
Mass media campaigns have long been.
Molecular Stress in Aging Neurons Explained
As the population ages, scientists are.
Higher BMI and Hypothyroidism Risk Study
A major longitudinal study from Canada.
Therapeutic Plasma Exchange Reduces Biological Age
Therapeutic plasma exchange (TPE), especially when.
Childhood Cancer Diagnosis Delays Persist
Delays in childhood cancer diagnosis remain.
Hypothalamic Changes in Eating Disorders Explained
Groundbreaking MRI scans reveal microstructural hypothalamic.
Leave a Comment