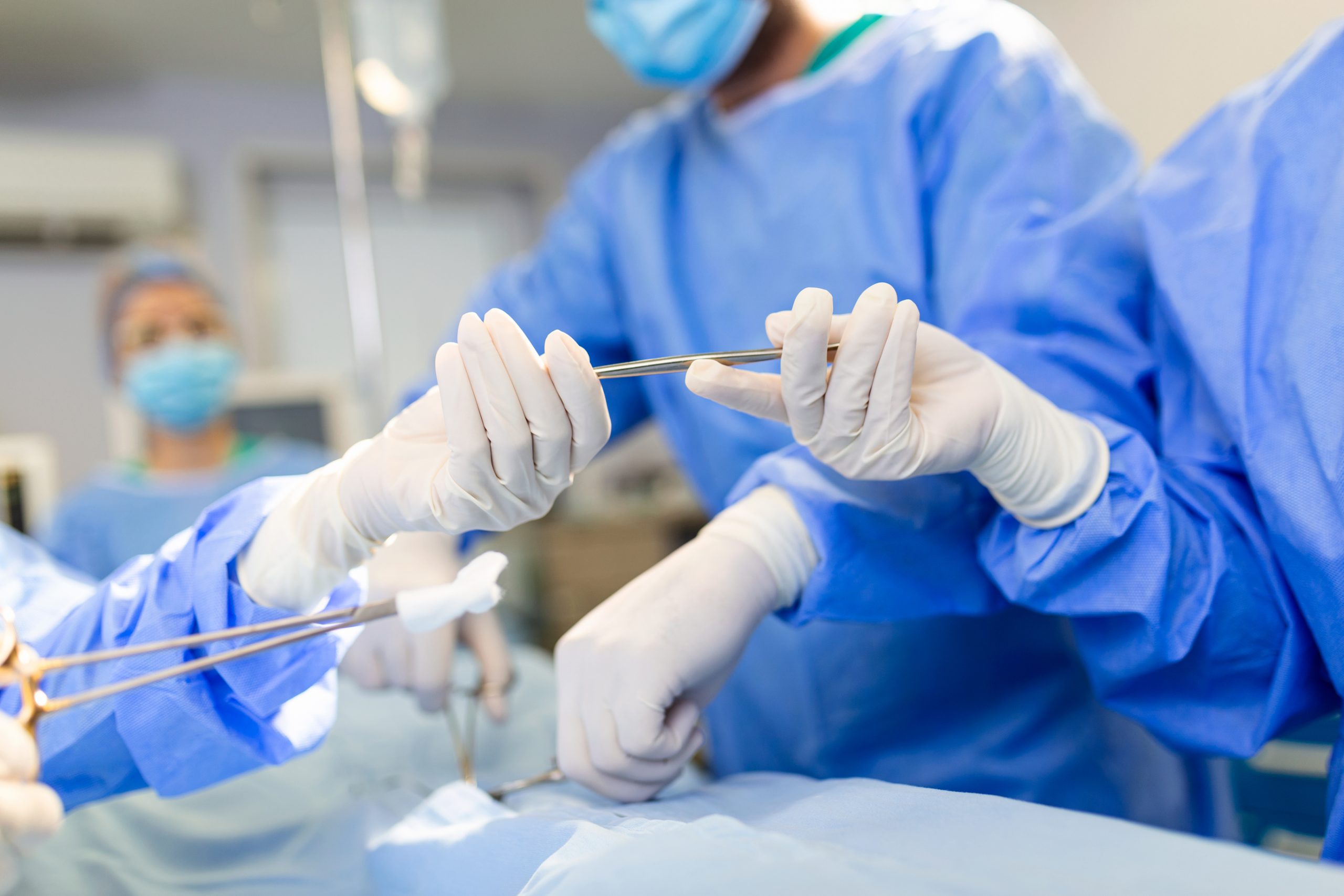
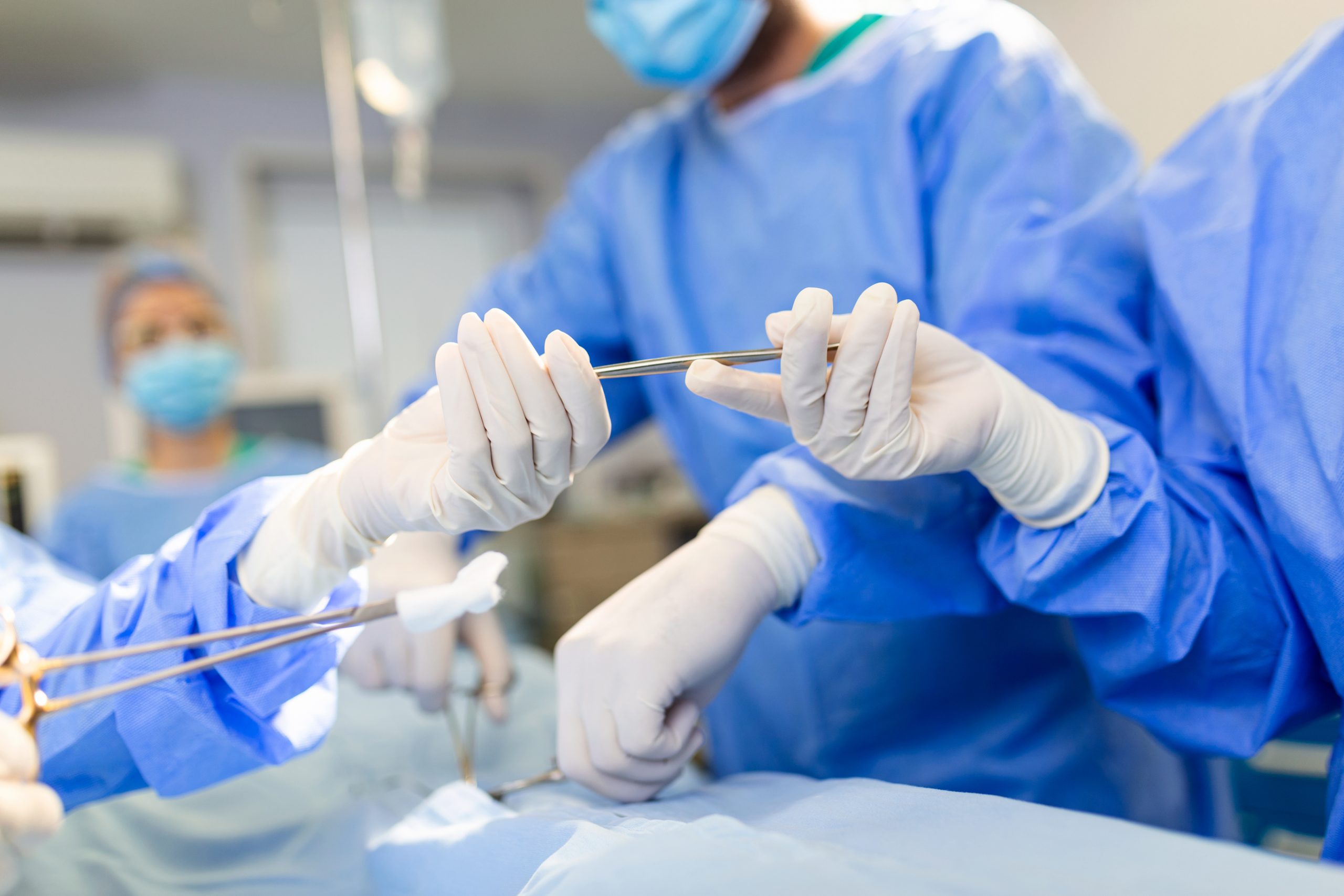
According to a new study published in JAMA Network Open, researchers and clinicians at the University of Pittsburgh and UPMC employed machine learning to design and implement a reliable and flexible model for predicting surgery complications in patients.
Prior to the COVID-19 pandemic, complications 30 days following surgery were the third largest cause of mortality worldwide, killing over 4.2 million people each year. Identifying high-risk individuals for complications before surgery is crucial to saving lives and minimizing health-care expenditures.
“Improving overall health of patients prior to surgery through prehabilitation can go a long way in improving outcomes for high-risk patients,” said Aman Mahajan, M.D., Ph.D., M.B.A., chair of Anesthesiology and Perioperative Medicine, Pitt School of Medicine, and director of UPMC Perioperative and Surgical Services. “However, identifying high-risk patients can be challenging for busy clinicians, who have to integrate the wealth of health data available and frequently perform additional testing and clinical assessments. We wanted to build an easy-to-use model that provides the health care team with an automated and accurate risk assessment quickly using existing data in the electronic health record.”
To develop the model, Mahajan and Oscar Marroquin, M.D., chief health care data and analytics officer at UPMC, and their colleagues trained the algorithm on over 1.25 million surgical patients’ medical records. The model focused on mortality and whether patients experienced a significant brain or cardiac event after surgery, such as a stroke or heart attack. The model was subsequently verified against another 200,000 UPMC surgery patients.
Following validation, the approach was implemented across 20 UPMC hospitals. Every morning, the computer scans the electronic medical record for patients scheduled for surgery and flags those deemed high-risk. This notification enables clinical teams to better coordinate care and implement some prehabilitation in advance of their operation, such as making healthier decisions or referring them to the UPMC Center for Perioperative Care, minimizing their risk of problems. Clinicians can also run the model on demand at any moment.
Mahajan and his team compared their approach to the American College of Surgeons’ National Surgical Quality Improvement Program (ACS NSQIP) to acquire a better grasp of how it compared to the industry standard. While the ACS NSQIP is utilized in hospitals across the country, doctors must manually enter patient information and cannot predict if information is missing. Mahajan and his colleagues discovered that their approach outperformed the ACS NSQIP at identifying high-risk patients.
“We designed our model with the health care worker in mind,” Marroquin said. “Since our model is completely automated and can make educated predictions even if some data are missing, it adds almost no additional burden to clinicians while providing them a reliable and useful tool.”
As the model is tweaked and extended further, Mahajan and his team hope to teach the computer to anticipate the possibility of sepsis, respiratory problems, and other complications that frequently detain patients in the hospital following surgery.
more recommended stories
Caffeine and SIDS: A New Prevention Theory
For the first time in decades,.
Microbial Metabolites Reveal Health Insights
The human body is not just.
Reelin and Cocaine Addiction: A Breakthrough Study
A groundbreaking study from the University.
Preeclampsia and Stroke Risk: Long-Term Effects
Preeclampsia (PE) – a hypertensive disorder.
Statins and Depression: No Added Benefit
What Are Statins Used For? Statins.
Azithromycin Resistance Rises After Mass Treatment
Mass drug administration (MDA) of azithromycin.
Generative AI in Health Campaigns: A Game-Changer
Mass media campaigns have long been.
Molecular Stress in Aging Neurons Explained
As the population ages, scientists are.
Higher BMI and Hypothyroidism Risk Study
A major longitudinal study from Canada.
Therapeutic Plasma Exchange Reduces Biological Age
Therapeutic plasma exchange (TPE), especially when.
Leave a Comment