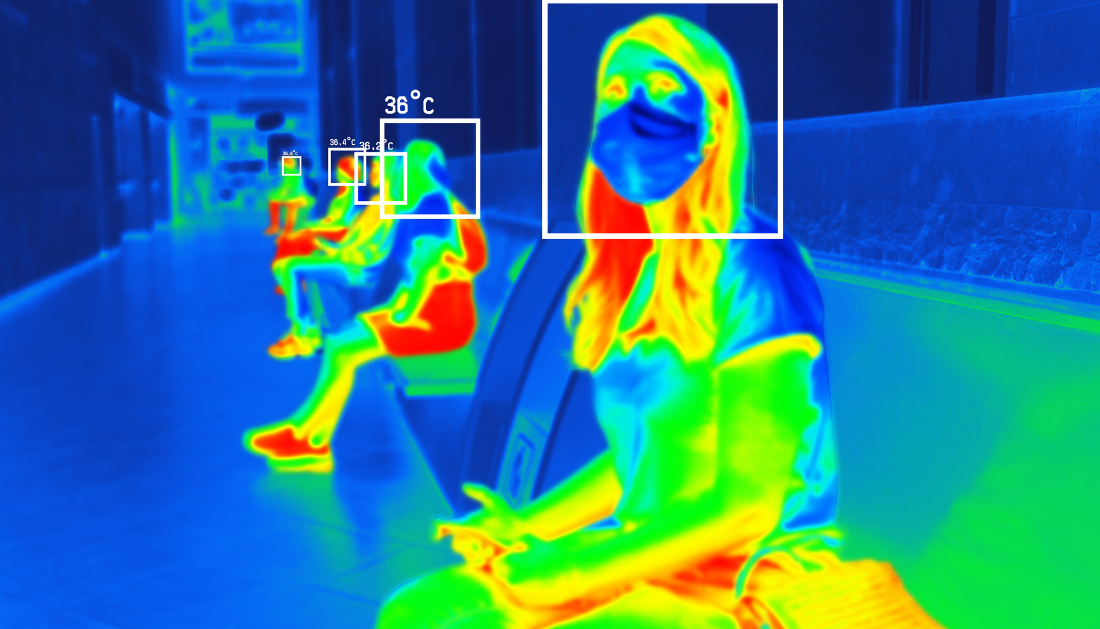
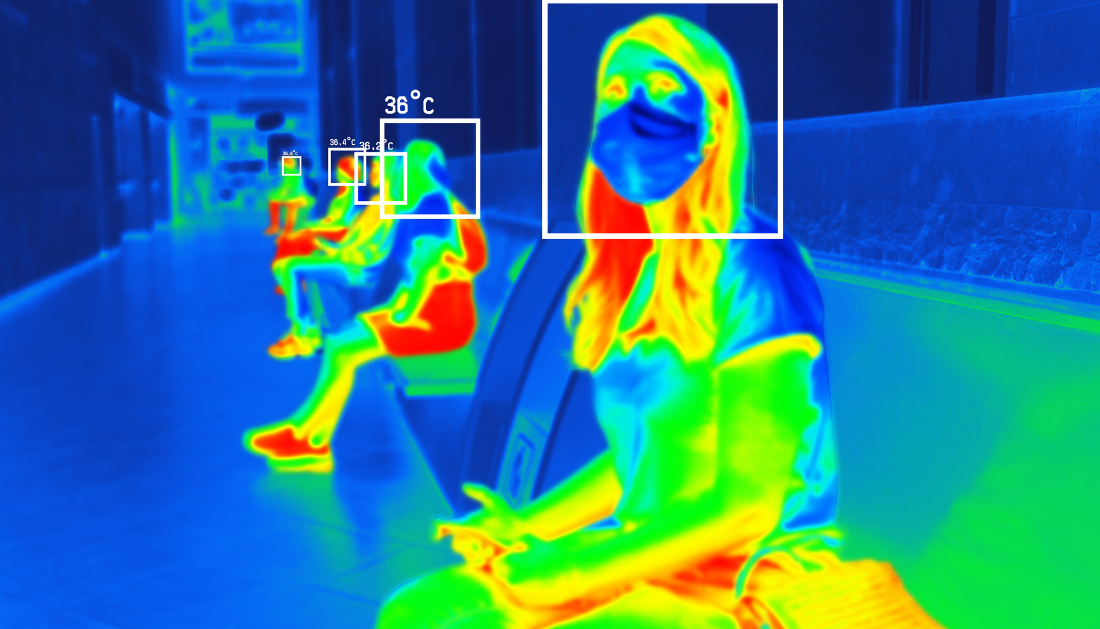
Researchers evaluated the viability of predicting coronary artery disease (CAD) using information from human facial infrared thermography (IRT) in a recent study that was published in the journal BMJ Health & Care Informatics.
A major global burden of death, coronary artery disease (CAD) is one of the primary causes of death. Care and treatment for CAD patients depend on accurate CAD assessment. Currently, in suspected patients, pretest probability tools (PTPs) are utilized to estimate the likelihood of CAD. However, these instruments have low precision, limited generalizability, and subjectivity problems.
While more sophisticated clinical models incorporating additional laboratory markers and risk factors or additional cardiovascular examinations (e.g., electrocardiography and coronary artery calcium score) may enhance probability estimates, issues with time efficiency, procedural complexity, and limited availability persist.
For the assessment of diseases, IRT, a non-contact surface temperature detection method, has shown promise. By analyzing patterns in skin temperature, it is possible to detect inflammation and irregular blood circulation. Research shows correlations between IRT data and factors relevant to atherosclerotic cardiovascular disease.
Concerning the study
Researchers assessed whether facial IRT temperature data might be used to predict CAD in the current study. Participants in the study were adults receiving either invasive coronary angiography (ICA) or coronary CT angiography (CCTA). Before CCTA or ICA, trained staff collected baseline data and carried out IRT videography.
Additional data, such as blood biochemistry, clinical history, risk factors, and results from the CAD workup, were obtained from electronic medical records. Each participant was given one IRT image, which was treated (grayscale conversion, background cropping, and uniform resizing) prior to analysis. The existence of CAD, which is defined as a coronary lesion stenosis of at least 50%, was the prediction of interest.
The group created an IRT picture model using a cutting-edge deep learning technique. In addition, two models were created for comparison: the PTP model, which served as the clinical baseline and contained the age, sex, and symptom characteristics of the patients, and the hybrid model, which combined IRT and clinical data from the PTP and IRT image models, respectively.
Numerous interpretation analyses were carried out, including as dose-response analysis, CAD surrogate label prediction, saliency map visualization, and occlusion experiments. Additionally, a variety of IRT tabular features were retrieved from the IRT image and categorized into levels relevant to the ROI and the complete face.
Temperature, fractal analysis characteristics, second-order texture, and first-order texture comprised the majority of the retrieved features. These collected features were combined by the XGBoost algorithm, which then assessed their prediction potential for CAD. Only temperature features were used by the researchers to evaluate performance.
Results
Between September 2021 and February 2023, 893 persons undergoing CCTA or ICA underwent screening in total. Of them, 460 individuals with an average age of 58.4 were included; of them, 70% had CAD and 27.4% were female. Compared to people without CAD, CAD subjects were more likely to be male, older, and to have a higher prevalence of risk factors. The IRT image model outperformed the PTP model by a significant margin.
Nonetheless, there was no discernible difference in the performance of the hybrid and IRT image models. The best prediction performance was achieved by using all extracted features or only temperature features, which was consistent with the IRT picture model. The overall left-right temperature differential had the greatest effect on the whole-face, whereas the average temperature of the left jaw had the most effect on the ROI-specific level.
When occluding distinct ROIs, the IRT picture model showed varying degrees of performance degradation. The most notable effect was caused by the blockage of the area between the upper and lower lips. In addition, the IRT image model demonstrated good predictive performance for surrogate labels linked with CAD, including inflammation, body mass index, smoking, hyperlipidemia, and glycated hemoglobin.
In conclusion
The study demonstrated that human facial IRT temperature data might be used to predict CAD. The IRT image model outperformed the PTP model that was advised by guidelines, demonstrating its potential for CAD evaluation. Moreover, adding clinical data to the IRT image model did not result in any further improvements, indicating that the important CAD-related information was already included in the retrieved facial IRT data.
Furthermore, interpretable IRT tabular features were used to validate the predictive value of the IRT model, and the results were largely consistent with the IRT image model. These human-interpretable IRT features also shed light on elements like distribution non-uniformity and facial temperature symmetry that are crucial for CAD prognosis. Validation requires more research with bigger sample sizes and a wider range of demographics.
For more information: Prediction of coronary artery disease based on facial temperature information captured by non-contact infrared thermography, BMJ Health & Care Informatics, https://doi.org/10.1136/bmjhci-2023-100942
more recommended stories
Scientists Unveil Next-Gen Eye-Tracking with Unmatched Precision
Eye-tracking technology has long been a.
New Study Questions Fluid Restriction in Heart Failure Management
A groundbreaking study presented at the.
Role of Leptin Signaling in the DMH for Metabolic Regulation
A groundbreaking study from the Pennington.
COVID-19 Vaccines May Lower the Risk of Long COVID by 27%
A recent rapid review suggests that.
3D-Printed Hydrogel for Meniscus Tear Treatment
Meniscus tears are one of the.
Machine Learning Predicts Early Mortality in IBD Patients
A groundbreaking study published in the.
Endometriosis Treatment Advances: Latest Research and Therapy
Recent endometriosis treatment advances are reshaping.
Lung Cancer Screening Gaps Persist Despite Updated Guidelines
A recent study led by researchers.
Altered Knee Movement After ACL Surgery May Trigger Early Osteoarthritis
A recent study published in the.
BRP Peptide for Weight Loss: A Natural Alternative to Ozempic?
The rising obesity epidemic has fueled.
Leave a Comment