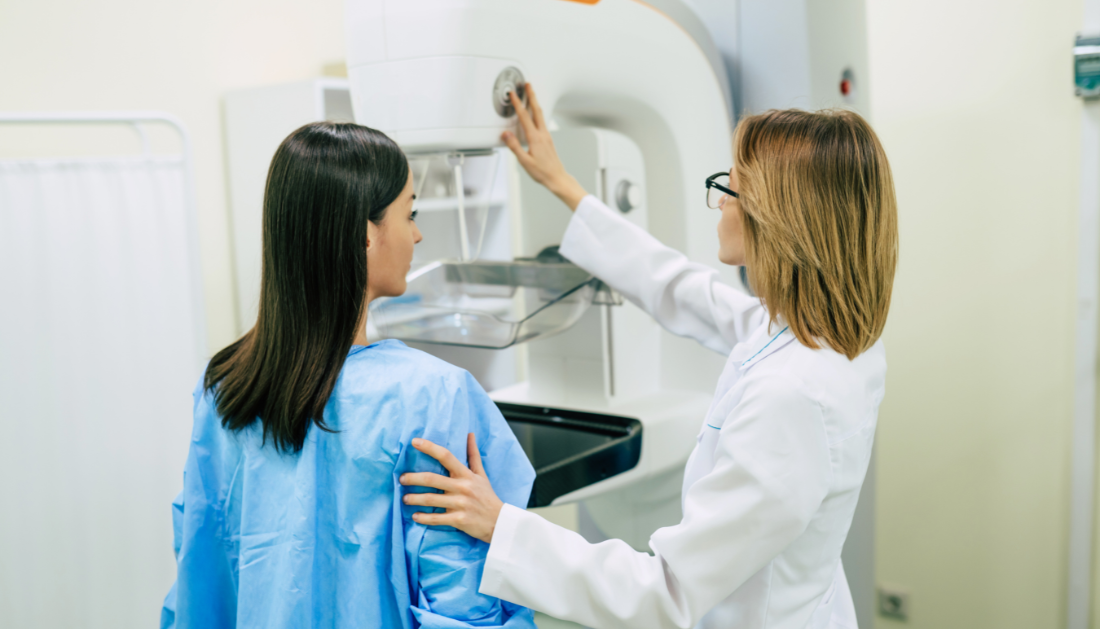
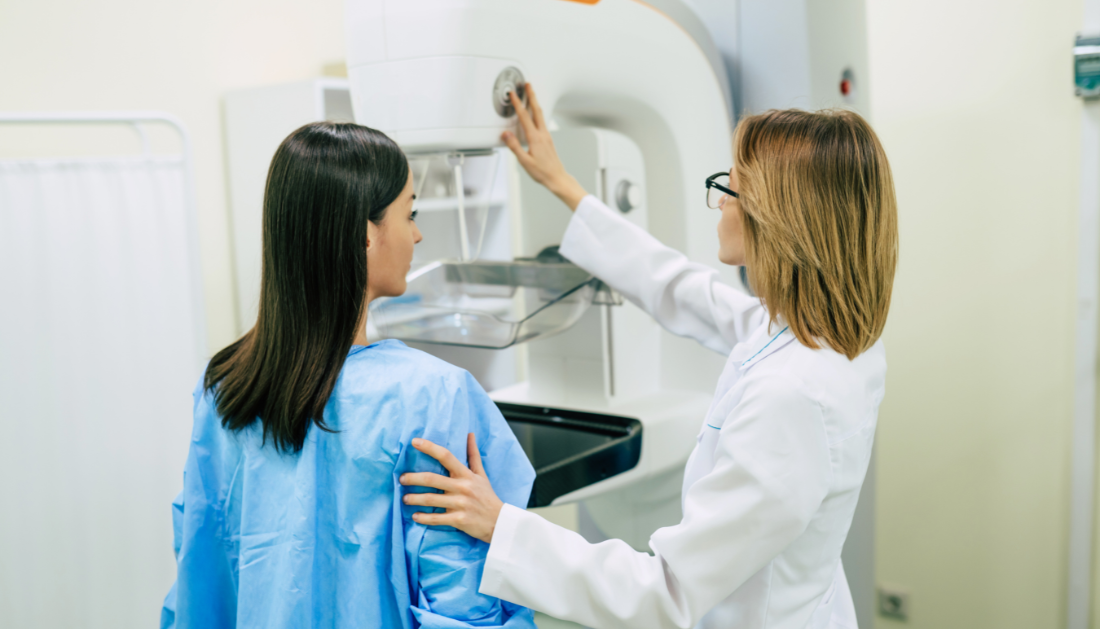
In a new article published in Trends in Cancer, researchers summarized how artificial intelligence (AI) enhances mammography-based risk prediction for breast cancer.
Introduction – Mammography Density
Since the publication of a landmark research in 1976, many physicians and scientists have been interested in the idea of using mammograms to predict future breast cancer diagnoses.
As a result, breast cancer risk might be calculated only based on the parenchymal pattern seen on mammography. These patterns, now known as mammographic or breast density, describe the appearance of dark and bright areas on a mammography.
Breast tissue that appears dark (on a mammography) is considered radiologically non-dense, whereas white is considered dense. The dense portions are primarily composed of stroma and epithelium, also known as fibroglandular tissues, whereas the non-dense areas are primarily composed of adipose tissue.
Individuals who have a higher mammographic density for their age and body mass index are more likely to develop breast cancer.
Furthermore, such individuals have an additional issue known as the masking effect, which occurs as mammographic density increases, making breast cancer detection more difficult.
In a substantial improvement over mammography-based risk prediction, AI approaches can find mammographic characteristics that are distinct parenchymal patterns and stronger risk predictors. The current study examined developments in AI-based breast cancer risk prediction.
AI-generated mammography features
Studies examining the links between AI-generated mammographic characteristics and breast cancer risk are observational, and the measured results do not always suggest causality. Notably, AI techniques may identify locations with a high risk score on mammograms.
However, because these areas do not show worrisome in standard radiography readings, they may be difficult to identify as radiological findings.
As a result, AI-generated features remain a “black box,” with limited reproducibility beyond the training and testing sample sets. Furthermore, it is crucial to understand the pathobiology linked with mammographic characteristics and the mechanisms that link them to breast cancer.
This will also be critical in determining their relevance to breast cancer risk and measures to reduce the risk.
A recent experiment found 36 breast tumors in 559 magnetic resonance imaging (MRI)-based screens of females who had a negative mammogram and higher AI-based risk scores. These data imply that some mammographic characteristics are indicative of early cancer.
Nonetheless, the identity of mammographic characteristics is unknown. As a result, research must focus on creating novel ways to identifying and characterizing the pathobiology of mammographic characteristics.
Implications for screening and risk mitigation strategies
Mammographic density has sparked widespread interest due to its incorporation in risk prediction algorithms, masking effect, and potential as a biomarker. This interest will grow with the introduction of mammographic features.
Furthermore, this study offers potential to identify persons at the highest risk of breast cancer and distinguish them from those who are at danger of developing breast cancer but are overlooked due to the masking effect.
Individuals with high-risk mammographic characteristics may benefit from more regular screenings and risk-reducing medications. In other words, women with a low risk of breast cancer could receive longer intervals between screenings in the next five years.
Additionally, an individual with increased mammographic density but no high-risk mammographic traits may benefit from supplementary imaging.
Mammographic features also provide for risk-reduction methods. Anti-estrogens, such as tamoxifen, may reduce the risk of breast cancer by decreasing mammographic density.
However, it is unclear whether anti-estrogens or other medications can alter mammographic characteristics. Furthermore, any risk-reducing drug will require evidence from randomized clinical trials showing it reduces risk through its influence on mammographic characteristics.
Conclusion
AI research has uncovered novel features that might explain a large proportion of short-term associations between breast cancer risk and mammographic density. However, this does not imply that mammographic density is no longer a risk factor.
Nevertheless, stronger risk factors can be detected on a mammogram that better pinpoint changes in parenchymal patterns preceding breast cancer detection.
Further research is needed to explore the causal links between mammographic features, mammographic density, and breast cancer risk and inform risk-reducing strategies.
For more information: Ingman WV, Britt KL, Stone J, Nguyen TL, Hopper JL, Thompson EW. (2024) Artificial intelligence improves mammography-based breast cancer risk prediction. Trends in Cancer.
doi: 10.1016/j.trecan.2024.10.007. https://www.cell.com/trends/cancer/fulltext/S2405-8033(24)00226-7
more recommended stories
Harmful Chemicals in Children’s Mattresses
A recent study has brought to.
TMS for Post-Stroke Aphasia Shows Remarkable Gains
A new clinical study led by.
CT Scan Overuse May Cause 1 in 20 New U.S. Cancers
A recent study published in JAMA.
Lactation Metabolism: Brain-Hormone Link Uncovered
Understanding how the body adjusts to.
Osteoarthritis Genetics Study Uncovers New Treatment Hope
Osteoarthritis- the world’s leading cause of.
Quantum Technology in Cancer Surgery: New Probe Aims to Improve Outcomes
A groundbreaking project from the University.
Antibody Breakthrough in Whooping Cough Vaccine
Whooping cough vaccine development is entering.
Bean-Based Gum Offers New Approach to Combat Influenza and Herpes
In an era where infectious diseases.
Shingles Vaccine May Cut Dementia Risk by 20%
A new study shows that the.
Scientists Unveil Next-Gen Eye-Tracking with Unmatched Precision
Eye-tracking technology has long been a.
Leave a Comment