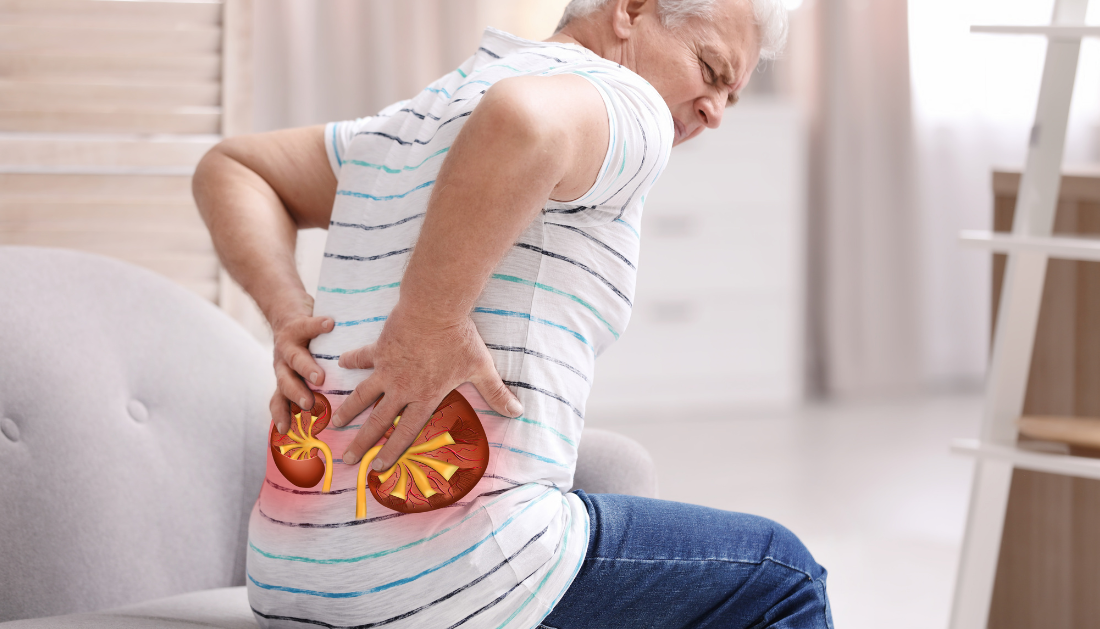
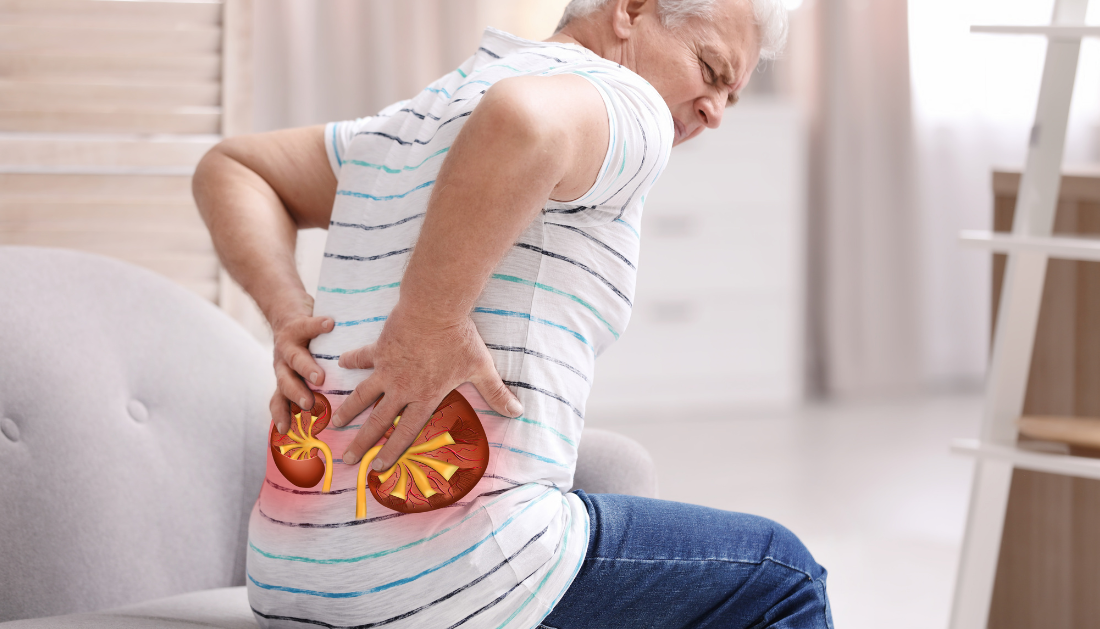
Hospital-acquired acute kidney injury (HA-AKI) is a common complication in hospitalized patients that can progress to chronic renal disease. It is related with longer hospital stays, higher health-care expenses, and increased death. Given these harmful repercussions, avoiding HA-AKI may improve hospitalized patient outcomes. Anticipating the beginning of HA-AKI is challenging due to the vast number of contributing factors.
Mass General Brigham Digital researchers used a commercial machine learning tool, the Epic Risk of HA-AKI predictive model, and discovered that it was somewhat successful in predicting the risk of HA-AKI in recorded patient data. The study discovered lesser performance than that recorded by Epic Systems Corporation’s internal validation, emphasizing the significance of testing AI models before clinical use.
The Epic model assesses adult inpatient visits for the risk of HA-AKI, which is characterized by specified elevations in serum creatinine. After training the model with data from MGB hospitals, the researchers evaluated it on approximately 40,000 inpatient hospital stays over a five-month period from August 2022 to January 2023. The dataset was broad, with numerous points collected from patient contacts, including patient demographics, comorbidities, primary illnesses, serum creatinine levels, and length of hospital stay. Two analyses were done to assess model performance at the encounter and prediction levels.
The researchers discovered that the instrument was more reliable when screening patients at lower risk of HA-AKI. Although the algorithm accurately predicted which low-risk individuals would not develop HA-AKI, it struggled to predict when higher-risk patients would develop HA-AKI. The results also differed based on the stage of HA-AKI being studied; predictions were more successful for Stage 1 HA-AKI than for more severe instances.
Overall, the authors found that deployment may result in significant false-positive rates and recommended additional research into the tool’s therapeutic impact.
“We found that the Epic predictive model was better at ruling out low-risk patients than identifying high-risk patients,” said lead study author Sayon Dutta, MD, MPH, of Mass General Brigham Digital’s Clinical Informatics team, and an emergency medicine physician at Massachusetts General Hospital. “Identifying HA-AKI risk with predictive models could help support clinical decisions such as by warning providers against ordering nephrotoxic medications, but further study is needed before clinical implementation.”
For more information: Sayon Dutta et al, External Validation of a Commercial Acute Kidney Injury Predictive Model, NEJM AI (2024). DOI: 10.1056/AIoa2300099
more recommended stories
ADHD and Gut Health: The Role of Chili Peppers
The Gut Health-Brain Axis and ADHD:.
HEALEY Platform Accelerates ALS Therapy Research
A New Era of ALS Clinical.
Can Your Genetics Influence Your Income and Health?
A New Perspective on Health and.
Low-Oxygen Therapy in a HypoxyStat Pill? Scientists Say It’s Possible
A New Approach to Oxygen Regulation-HypoxyStat.
Tracking Immune Cells in Blood Predicts Cancer Survival
A new study from University College.
Dream Recall: The Role of Personality, Sleep, and Cognitive Traits
A recent study from the IMT.
Higher BMI Linked to Stronger Memory in Midlife Adults
Does Obesity Boost Brainpower? Study Links.
Antibiotic-Resistant Bacteria Found in Hospital Drains
Dangerous Bacteria Found in Hospital Sink.
Pancreatic Cancer Immune Map May Guide Future Therapies
New Immune Map Reveals Key Insights.
Brain-Like AI Explains Relational Learning
AI Unveils How the Brain Learns.
Leave a Comment