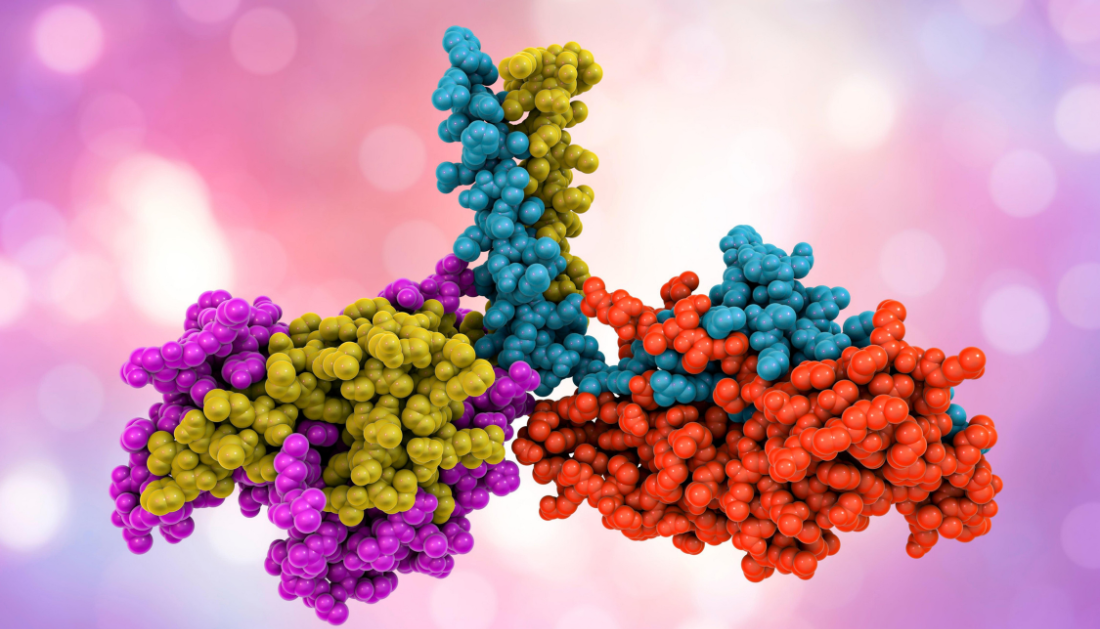
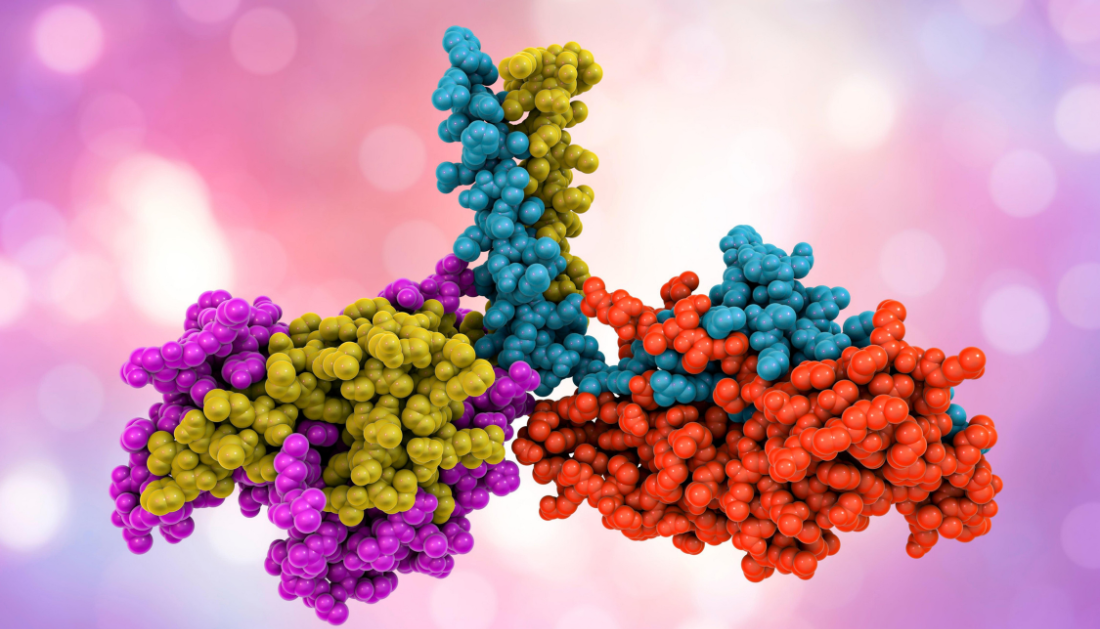
Protein design seeks to develop personalized antibodies for therapeutics, biosensors for diagnostics, and enzymes for chemical processes. An international research team has now devised a way for improving the design of huge novel proteins and generating them with the appropriate features in the laboratory. Their technique entails a novel way of utilizing the capabilities of the AI-based software Alphafold2, for which the Nobel Prize in Chemistry was awarded in 2024.
Proteins are essential components of our bodies, serving as building blocks, transport systems, enzymes, and antibodies. Researchers are thus attempting to reproduce them or build so-called de novo proteins, which do not occur naturally. Such engineered proteins are intended to bind to specific viruses or deliver medications, for example. Scientists are increasingly employing machine learning to create them. The Nobel Prize in Chemistry was recently awarded to David Baker, a pioneer in de novo protein creation, as well as Demis Hassabis and John Jumper, developers of the software Alphafold2. This software allows you to predict protein structures on the computer with great accuracy.
An international team led by Hendrik Dietz, Professor of Biomolecular Nanotechnology at the Technical University of Munich (TUM), and Sergey Ovchinnikov, Professor of Biology at MIT, has developed a method for efficient protein design that combines Alphafold2’s accurate structure prediction with a gradient descent approach. It appeared in the journal Science.
Gradient descent is a popular method for model optimization. It can be used in a step-by-step manner to find deviations from the desired target function and change the parameters until the best result is obtained. In protein design, gradient descent can be used to compare the structure of new proteins predicted by AlphaFold2 to the intended protein structure. This enables scientists to improve their newly developed amino acid chain and its subsequent structure. The latter determines the protein’s stability and function and is dependent on delicate energy interactions.
Virtual superposition of the building blocks
The new technology allows for improved creation of big novel proteins and tailoring them to certain qualities, such as precise binding to other proteins. Their design technique is different from prior approaches in various respects.
We have designed the process for new proteins so that we initially ignore the limits of what is physically possible. Usually, only one of the 20 possible building blocks is assumed at each point of the amino acid chain. Instead, we use a variant in which all possibilities are virtually superimposed.”
Christopher Frank, doctoral candidate at the Chair of Biomolecular Nanotechnology and first author of the study
This virtual superposition cannot be directly translated into an actually producible protein. But it allows the protein to be iteratively optimized. “We improve the arrangement of the amino acids in several iterations until the new protein is very close to the desired structure,” says Christopher Frank. This optimized structure is then used to determine the amino acid sequence that can actually be assembled to a protein in the laboratory.
The crucial test: how do the predictions hold up in real life?
The ultimate test for all newly designed proteins: does the actual structure correspond to the predicted construct and the desired function? Using the new method, the team designed more than 100 proteins virtually, produced them in the laboratory and tested them experimentally. „We were able to show that the structures that we designed are very close to the structures that are actually produced,” says Christopher Frank.
Using their new method, they were able to produce proteins consisting of up to 1000 amino acids. “This brings us closer to the size of antibodies, and – just as with antibodies – we can then integrate several desired functions into such a protein,” explains Hendrik Dietz. „These could, for example, be motifs for recognizing and suppressing pathogens.”
For more information: Frank, C., et al. (2024). Scalable protein design using optimization in a relaxed sequence space. Science. doi.org/10.1126/science.adq1741.
more recommended stories
Brain’s Biological Age Emerges as Key Health Risk Indicator
Clinical Significance of Brain Age in.
Children’s Health in the United States is Declining!
Summary: A comprehensive analysis of U.S..
Autoimmune Disorders: ADA2 as a Therapeutic Target
Adenosine deaminase 2 (ADA2) has emerged.
Is Prediabetes Reversible through Exercise?
150 Minutes of Weekly Exercise May.
New Blood Cancer Model Unveils Drug Resistance
New Lab Model Reveals Gene Mutation.
Healthy Habits Slash Diverticulitis Risk in Half: Clinical Insights
Healthy Habits Slash Diverticulitis Risk in.
Caffeine and SIDS: A New Prevention Theory
For the first time in decades,.
Microbial Metabolites Reveal Health Insights
The human body is not just.
Reelin and Cocaine Addiction: A Breakthrough Study
A groundbreaking study from the University.
Preeclampsia and Stroke Risk: Long-Term Effects
Preeclampsia (PE) – a hypertensive disorder.
Leave a Comment