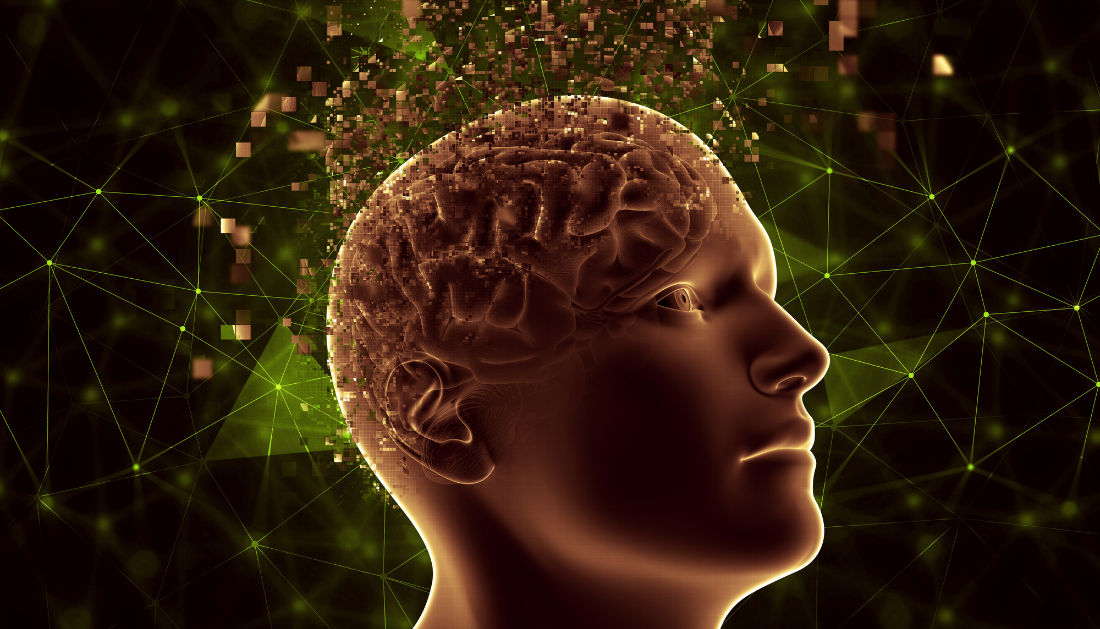
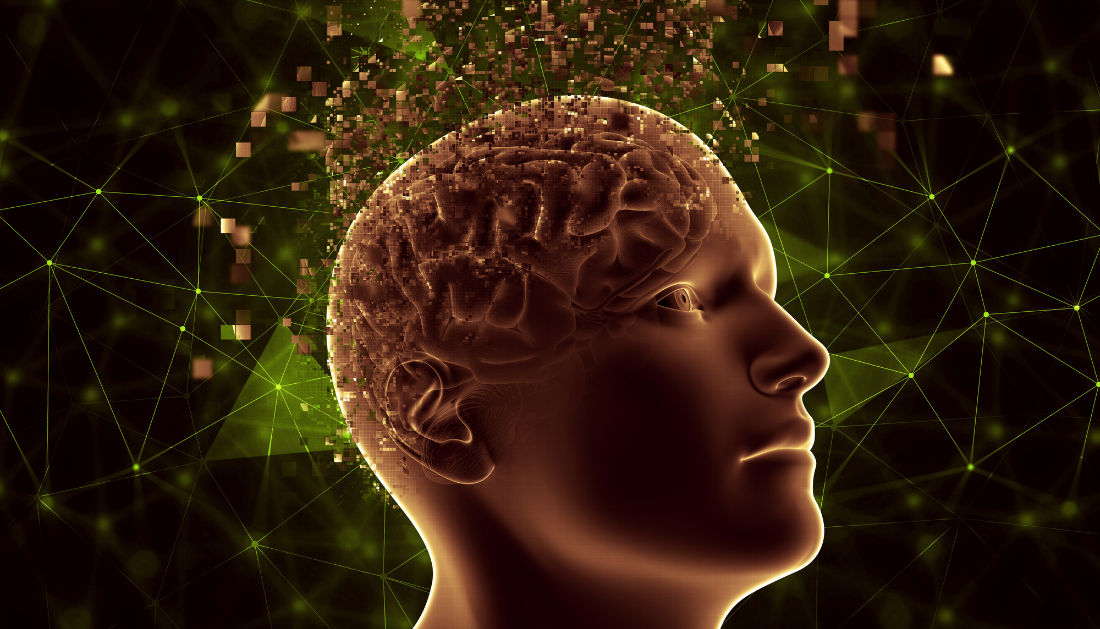
Maryam Shanechi and her team created a new machine learning method that uncovers unexpectedly constant intrinsic brain patterns across various people by separating these patterns from the effects of visual stimuli. The results have been published in the Proceedings of the National Academy of Sciences.
When we conduct common movements, like reaching for a book, our brain must process information, which is often in the form of visual input—for example, seeing where the book is. Our brain must then interpret this information internally in order to coordinate the action of our muscles and complete the movement.
But how do millions of neurons in our brain carry out such a task? Answering this question necessitates investigating the neurons’ aggregate activity patterns while disentangling the effect of input from the neurons’ intrinsic (or internal) activities, whether movement-related or not.
Shanechi, her Ph.D. student Parsa Vahidi, and a research associate in her lab, Omid Sani, developed a new machine-learning algorithm that mimics brain activity while taking into account movement behavior and sensory input.
“Prior methods for analyzing brain data have either considered neural activity and input but not behavior, or considered neural activity and behavior but not input,” said Shanechi.
“We developed a method that can consider all three signals—neural activity, behavior, and input—when extracting hidden brain patterns. This allowed us to not only disentangle input-related and intrinsic neural patterns, but also separate out which intrinsic patterns were related to movement behavior and which were not.”
Shanechi and her team applied this strategy to three publicly available datasets in which three different participants performed one of two unique movement tasks, such as moving a cursor on a computer screen over a grid or consecutively to random points.
“When using methods that did not consider all three signals, the patterns found in neural activity of these three subjects looked different,” Vahidi said in a statement. However, when the team applied the novel approach to all three signals, a strikingly consistent hidden pattern emerged from all three subjects’ brain activity that was important to movement. This closeness persisted despite the fact that the three subjects’ tasks were not the same.
“In addition to revealing this new consistent pattern, the method also improved the prediction of neural activity and behavior compared to when all three signals were not considered during machine learning, as in prior work,” said Sani. “The new method enables researchers to more accurately model neural and behavioral data by accounting for various measured inputs to the brain, such as sensory inputs as in this work, electrical or optogenetic stimulation, or even input from different brain areas.”
This strategy and the pattern found can help us comprehend how our brains conduct motions in response to information from the outside environment. Furthermore, by modeling the effect of input and distinguishing intrinsic patterns that are behaviorally relevant, this method can aid in the development of future brain-computer interfaces that regulate abnormal brain patterns in disorders such as major depression by optimizing external inputs such as deep brain stimulation therapy.
“We are excited about how this algorithm could facilitate both scientific discoveries and the development of future neurotechnologies for millions of patients with neurological or neuropsychiatric disorders,” said Shanechi.
More information: Parsa Vahidi et al, Modeling and dissociation of intrinsic and input-driven neural population dynamics underlying behavior, Proceedings of the National Academy of Sciences (2024). DOI: 10.1073/pnas.2212887121
more recommended stories
Label-Free Motion Analysis Detects Cancer Cells
Label-free motion analysis is emerging as.
Harmful Chemicals in Children’s Mattresses
A recent study has brought to.
TMS for Post-Stroke Aphasia Shows Remarkable Gains
A new clinical study led by.
CT Scan Overuse May Cause 1 in 20 New U.S. Cancers
A recent study published in JAMA.
Lactation Metabolism: Brain-Hormone Link Uncovered
Understanding how the body adjusts to.
Quantum Technology in Cancer Surgery: New Probe Aims to Improve Outcomes
A groundbreaking project from the University.
Bean-Based Gum Offers New Approach to Combat Influenza and Herpes
In an era where infectious diseases.
Shingles Vaccine May Cut Dementia Risk by 20%
A new study shows that the.
New Study Questions Fluid Restriction in Heart Failure Management
A groundbreaking study presented at the.
Role of Leptin Signaling in the DMH for Metabolic Regulation
A groundbreaking study from the Pennington.
Leave a Comment