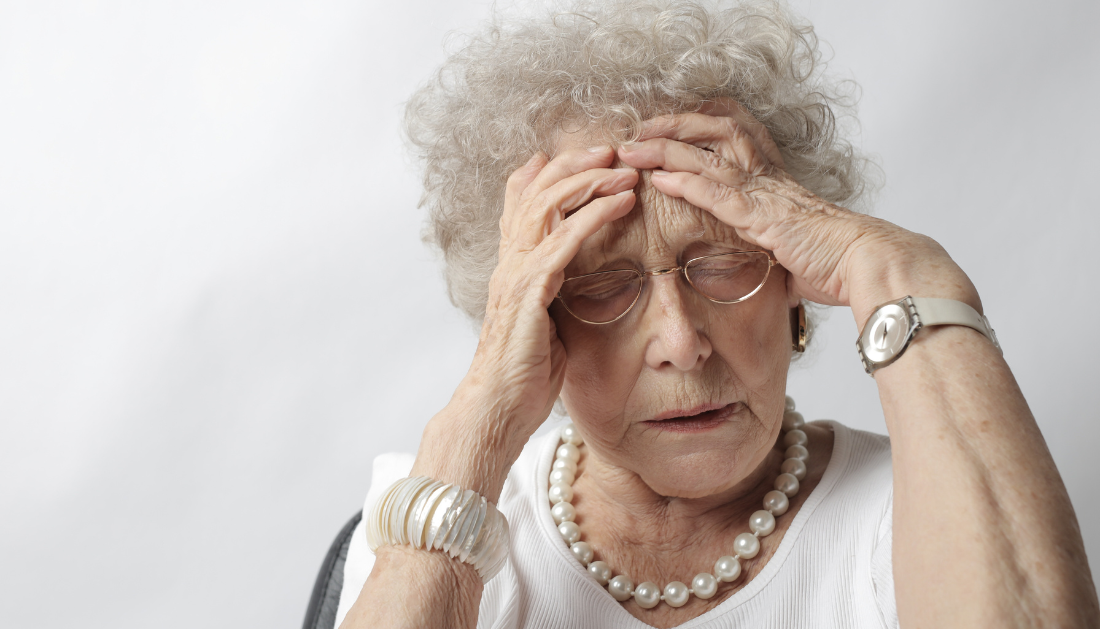
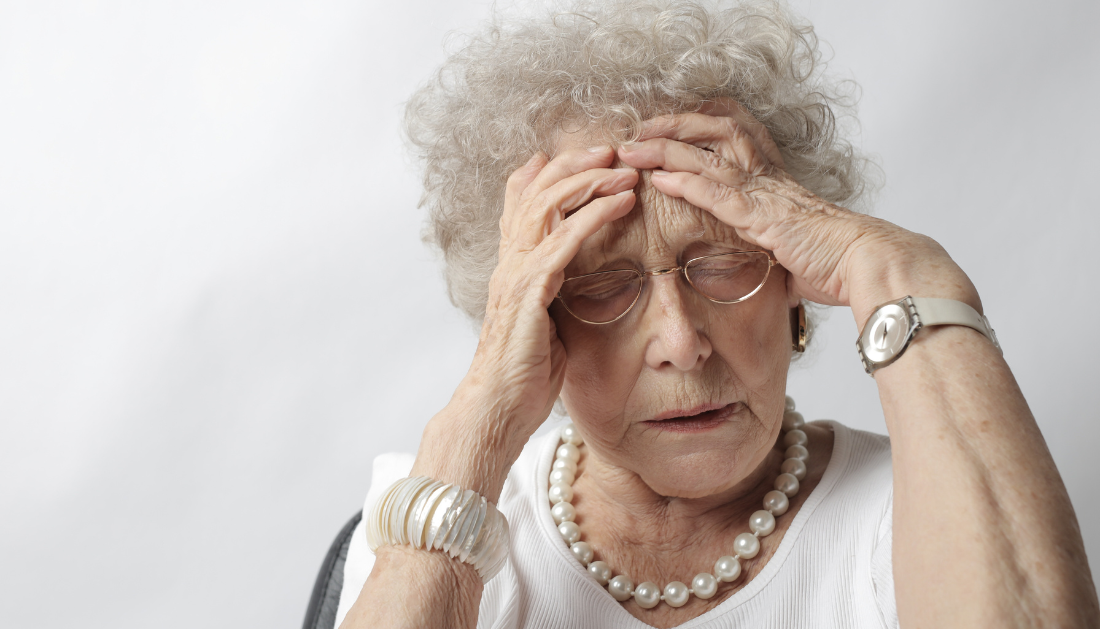
Columbia University Mailman School of Public Health researchers have created a novel computational pipeline for identifying protein biomarkers linked to complex disorders such as Alzheimer’s disease (AD). This novel method examines indicators that might cause 3D structural changes in proteins, providing vital insights into disease mechanisms and identifying potential treatment targets. The discoveries, published in Cell Genomics, have the potential to advance early detection and treatment options for Alzheimer’s disease, which has long resisted successful therapeutics.
Alzheimer’s disease is defined by amyloid-beta plaques and tau neurofibrillary tangles in the brain, which accumulate decades before symptoms. Current early diagnostics are either resource-intensive or invasive. Moreover, current AD therapies targeting amyloid-beta provide some symptomatic relief and may slow disease progression but fall short of halting it entirely. Our study highlights the urgent need to identify blood-based protein biomarkers that are less invasive and more accessible for early detection of Alzheimer’s disease. Such advancements could unravel the underlying mechanisms of the disease and pave the way for more effective treatments.”
Zhonghua Liu, ScD, assistant professor of Biostatistics at Columbia Mailman School, and senior investigator
A new approach to Alzheimer’s disease
Using data from the UK Biobank, which includes 54,306 participants, and a genome-wide association study (GWAS) with 455,258 subjects (71,880 AD cases and 383,378 controls), the research team identified seven key proteins—TREM2, PILRB, PILRA, EPHA1, CD33, RET, and CD55—that exhibit structural alterations linked to Alzheimer’s risk.
“We discovered that certain FDA-approved drugs already targeting these proteins could potentially be repurposed to treat Alzheimer’s,” Liu added. “Our findings underscore the potential of this pipeline to identify protein biomarkers that can serve as new therapeutic targets, as well as provide opportunities for drug repurposing in the fight against Alzheimer’s.”
The MR-SPI pipeline: Precision in disease prediction
The novel computational pipeline, known as MR-SPI (Mendelian Randomization by Selecting Genetic Instruments and Post-selection Inference), offers numerous significant advantages. Unlike standard approaches, MR-SPI does not necessitate a large number of candidate genetic instruments (e.g., protein quantitative trait loci) to find disease-associated proteins. MR-SPI is an effective method for research with a restricted number of genetic markers available.
“MR-SPI is particularly valuable for elucidating causal relationships in complex diseases like Alzheimer’s, where traditional approaches struggle,” Liu told me. “The integration of MR-SPI with AlphaFold3-;an advanced tool for predicting protein 3D structures-;further enhances its ability to predict 3D structural changes caused by genetic mutations, providing a deeper understanding of the molecular mechanisms driving disease.”
Implications for drug discovery and treatment
The study’s findings indicate that MR-SPI could have far-reaching applications beyond Alzheimer’s disease, providing a robust foundation for identifying protein biomarkers in a variety of complicated disorders. Furthermore, the capacity to predict 3D structural changes in proteins offers up new avenues for drug discovery and repurposing existing therapies.
“By combining MR-SPI with AlphaFold3, we can achieve a comprehensive computational pipeline that not only identifies potential drug targets but also predicts structural changes at the molecular level,” Liu concluded. “This pipeline offers exciting implications for therapeutic development and could pave the way for more effective treatments for Alzheimer’s and other complex diseases.”
“By leveraging large cohorts with biobanks, innovative statistical and computational approaches, and AI-based tools like AlphaFold this work represents a convergence of innovation that will improve our understanding of Alzheimer’s and other complex diseases,” said Gary W. Miller, PhD, Columbia Mailman Vice Dean for Research Strategy and Innovation and professor, Department of Environmental Health Sciences.
For more information: Yao, M., et al. (2024). Deciphering proteins in Alzheimer’s disease: A new Mendelian randomization method integrated with AlphaFold3 for 3D structure prediction. Cell Genomics. doi.org/10.1016/j.xgen.2024.100700.
more recommended stories
Limited Health Care Access During Disasters Delays Colorectal Cancer Diagnoses
Natural disasters like Hurricanes Irma and.
Ethical Issues in Human-AI Relationships
As artificial intelligence becomes increasingly lifelike.
Osteoarthritis Genetics Study Uncovers New Treatment Hope
Osteoarthritis- the world’s leading cause of.
Antibody Breakthrough in Whooping Cough Vaccine
Whooping cough vaccine development is entering.
Vegetarian Diets and Healthy Aging: Does Diet Quality Make a Difference?
Vegetarian diets are widely recognized for.
Mental Health Pros May Miss Bulimia Signs – Here’s Why
A recent study by the University.
Scientists Unveil Next-Gen Eye-Tracking with Unmatched Precision
Eye-tracking technology has long been a.
New Study Questions Fluid Restriction in Heart Failure Management
A groundbreaking study presented at the.
Men5CV: Hope for Ending Africa’s Meningitis Epidemics
A landmark global health study led.
Semen Quality as a Predictor of Long-Term Health
A landmark study published in Human.
Leave a Comment