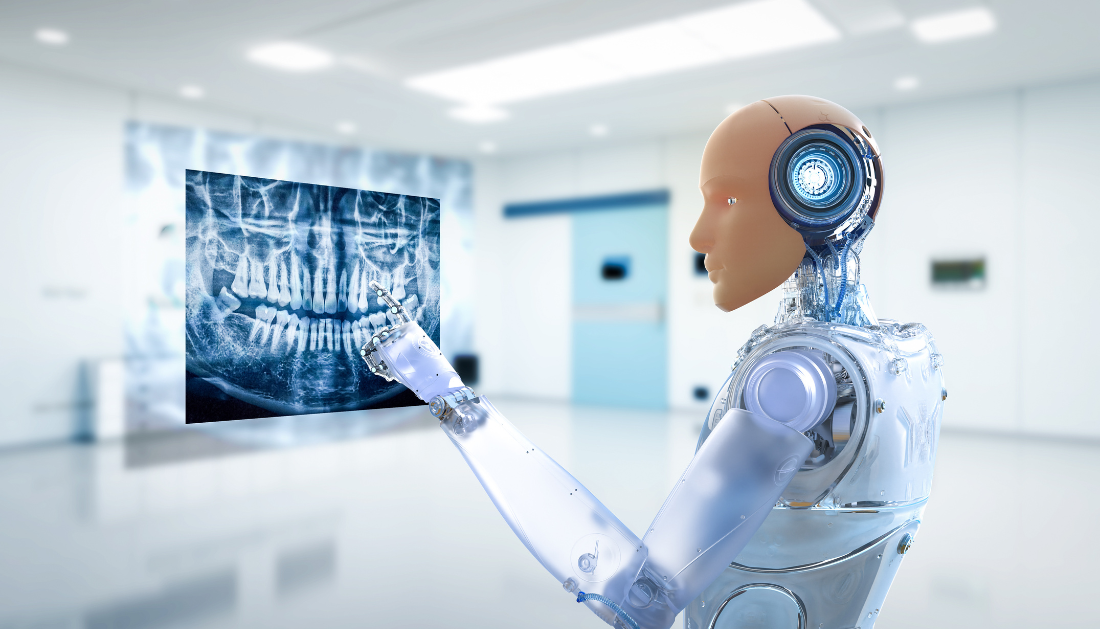
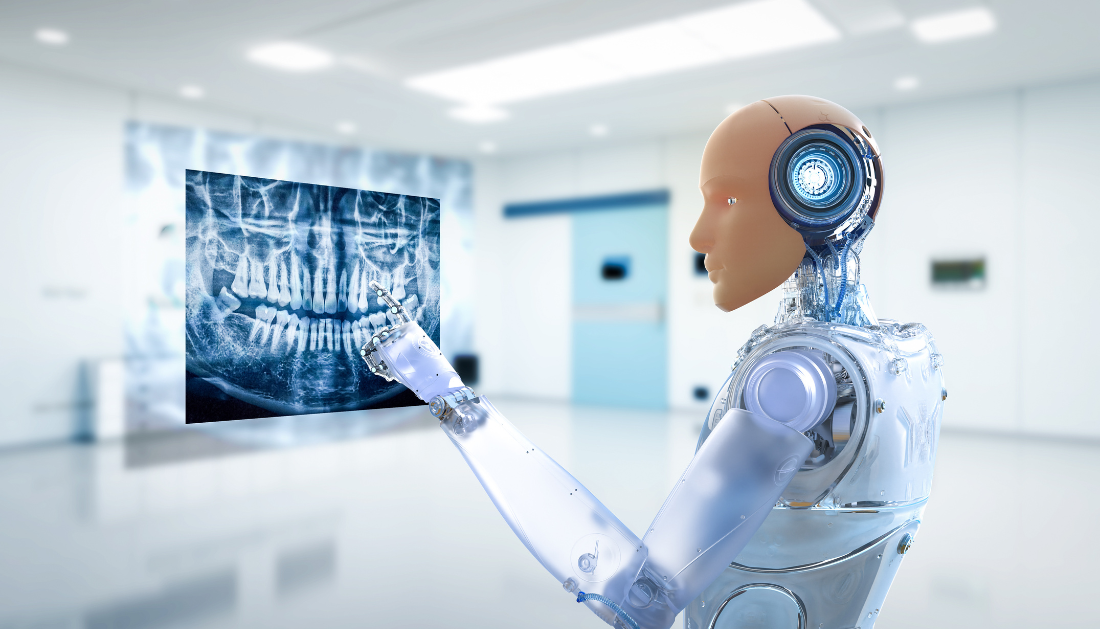
A groundbreaking study from Harvard Medical School and Stanford University, published in Nature Medicine, reveals that while AI doctors excel in standardized medical exams, they often struggle in real-world clinical conversations. The research introduces CRAFT-MD (Conversational Reasoning Assessment Framework for Testing in Medicine), a new evaluation tool designed to measure how well AI doctors or medical tools handle real-life patient interactions.
Large-language models (LLMs), such as ChatGPT, have shown promise in helping clinicians by triaging patients, collecting medical histories, and offering preliminary diagnoses. However, the study highlights a critical issue: AI models that perform well on structured, multiple-choice medical exams often falter when engaging in unstructured, back-and-forth conversations with patients.
CRAFT-MD evaluates AI performance in realistic patient scenarios by simulating clinical conversations where AI agents act as patients and graders. The assessment focuses on key factors such as information gathering, diagnostic reasoning, and conversational accuracy across 2,000 clinical vignettes spanning 12 medical specialties.
The results showed a clear pattern—AI tools often fail to ask the right follow-up questions, overlook essential details in patient history, and struggle to synthesize scattered information from open-ended exchanges. Their diagnostic accuracy dropped significantly compared to their performance on structured exams.
The research team, led by Pranav Rajpurkar, M.D., emphasized that dynamic doctor-patient interactions require AI to adapt, ask critical questions, and piece together fragmented information—abilities current AI tools struggle to master.
To address these limitations, the study recommends:
- Designing AI models with better conversational reasoning capabilities.
- Using open-ended testing frameworks instead of rigid multiple-choice formats.
- Training AI to process verbal and non-verbal cues, including tone and body language.
- Integrating textual and non-textual data, such as images and lab results, into diagnostics.
The findings advocate for a hybrid evaluation approach, where AI agents complement human experts for more efficient, scalable testing.
As noted by Roxana Daneshjou, M.D., from Stanford University, “CRAFT-MD represents a major step forward, offering a real-world evaluation framework to ensure AI tools meet clinical standards before being deployed in healthcare settings.”
Join this webinar to learn how Artificial intelligence can help psychiatrists in the real world.
More Information: An evaluation framework for clinical use of large language models in patient interaction tasks, Nature Medicine (2024). DOI: 10.1038/s41591-024-03328-5
more recommended stories
lncRNAs in Tumor Microenvironment Drive Cancer Progression
The tumor microenvironment (TME) is a.
Lyme Disease Symptoms: Why They Linger After Treatment
Lyme disease symptoms that persist after.
Body Image in Young Women: Cultural Influences Matter
A new international study led by.
Early Alzheimer’s Found Through Blood Biomarkers
A new study published in Molecular.
Label-Free Motion Analysis Detects Cancer Cells
Label-free motion analysis is emerging as.
Harmful Chemicals in Children’s Mattresses
A recent study has brought to.
TMS for Post-Stroke Aphasia Shows Remarkable Gains
A new clinical study led by.
CT Scan Overuse May Cause 1 in 20 New U.S. Cancers
A recent study published in JAMA.
Lactation Metabolism: Brain-Hormone Link Uncovered
Understanding how the body adjusts to.
Quantum Technology in Cancer Surgery: New Probe Aims to Improve Outcomes
A groundbreaking project from the University.
Leave a Comment