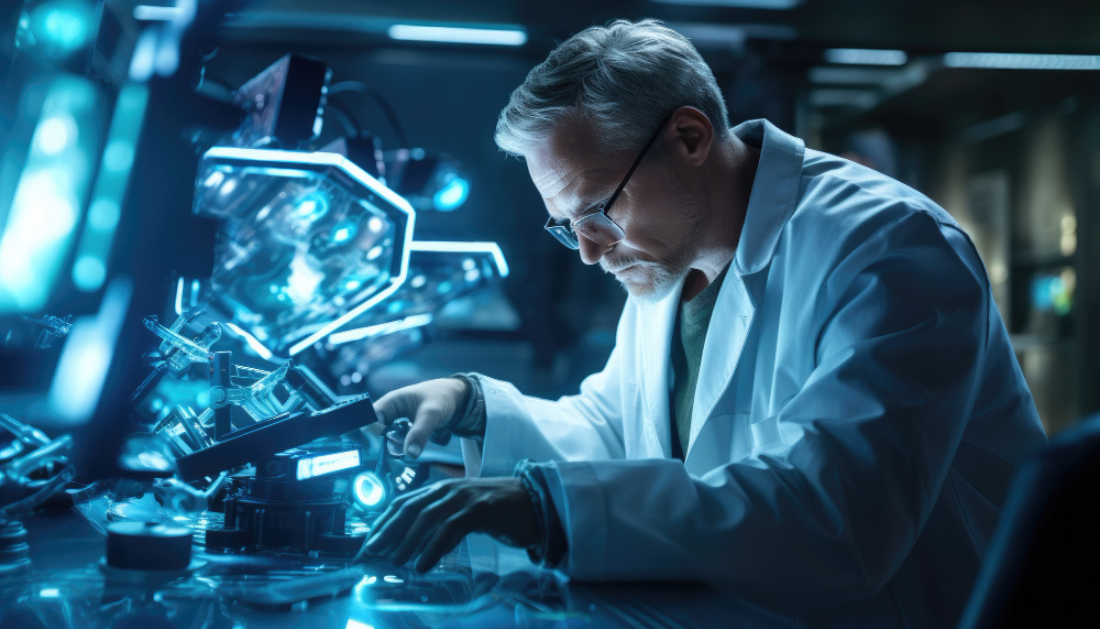
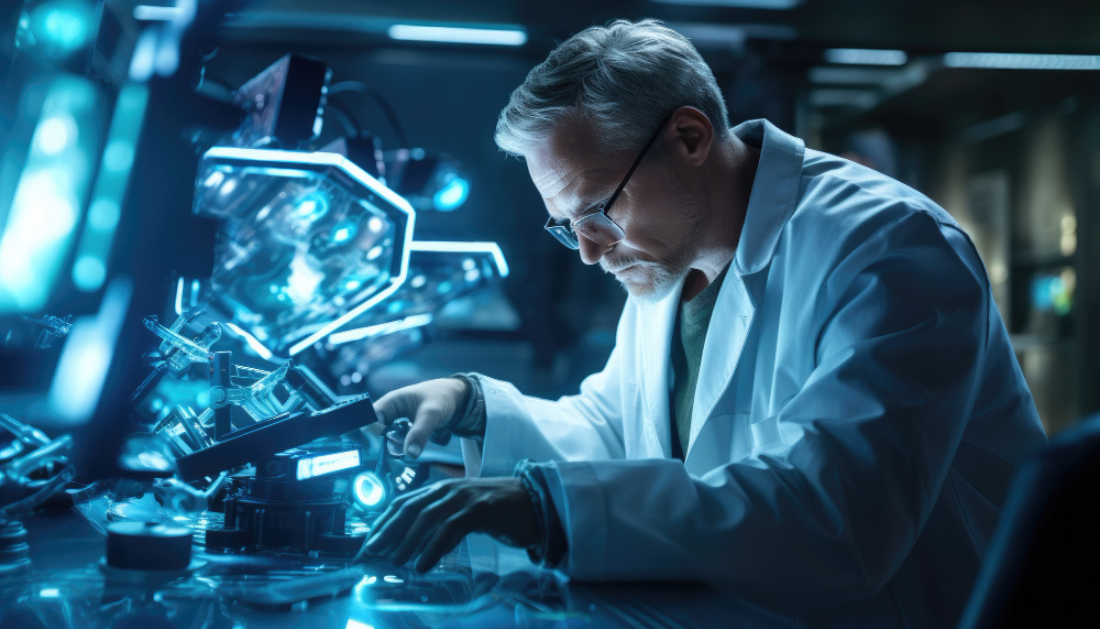
In a recent publication featured in the esteemed journal Cancer, scholarly articles explore the merits and constraints of AI precision medicine methodologies in the realm of oncology research and therapy.
Background:
The inquiry delves into the diagnostic and prognostic efficacy of artificial intelligence (AI) algorithms, particularly emphasizing the role of AI-driven chatbots (generative AI) in fostering favorable outcomes in cancer treatment over the past decades.
Additionally, it addresses the current obstacles hindering widespread AI integration and proposes regulatory frameworks to enhance the efficacy of these algorithms in the foreseeable future.
Precision Medicine and its Clinical Application in Anti-Cancer Therapies: Referred to colloquially as ‘personalized medicine,’ precision medicine represents a therapeutic paradigm that takes into account a patient’s individual genetic profile, environmental exposures, and lifestyle factors.
In contrast to conventional medical paradigms, which adhere to a ‘one size fits all’ approach, precision medicine offers manifold advantages, particularly in fields like oncology, where patient-specific characteristics (such as tumor profiles) significantly influence treatment outcomes, surpassing those achieved through generic chemotherapy.
Advancements in oncological practices have garnered significant scientific interest, with studies indicating a remarkable 33% reduction in cancer mortality rates over the past three decades.
However, escalating environmental pollutants and suboptimal lifestyle choices have concurrently impeded progress in the field, owing to the escalating variability of carcinogenic agents.
Precision medical interventions, particularly those harnessing artificial intelligence (AI) algorithms, hold promise in overcoming this limitation inherent in conventional, standardized medical approaches. These AI-driven methodologies empower researchers and clinicians to discern previously undetected patterns in patients’ radiological scans through the application of machine learning (ML) and deep learning (DL) technologies.
Regrettably, despite the development and rigorous testing of numerous AI algorithms for the management of cancer care, the integration of these technologies into mainstream medicine remains uncommon.
Prominent barriers to the adoption of AI models in research include their substantial initial implementation expenses, the lack of human interpretability regarding algorithmic outcomes, and the limited human oversight and validation of algorithms post-implementation.
Moreover, research endeavors across various facets and stages of cancer care lack uniformity, with a significantly larger body of literature focused on cancer diagnosis (exceeding 80%) compared to treatment and post-chemotherapy care.
Nevertheless, notwithstanding these challenges, the implementation of AI in oncology has significantly advanced the field, facilitating innovative diagnostic, prognostic, and chat-based information access for clinicians and their patients alike.
The current review delves into these advancements, delineating the merits and demerits of existing AI implementations while also addressing conventional and prospective hurdles in the widespread adoption of AI.
Furthermore, it proposes policy reforms that have the potential to alleviate the global burden of cancer, one of the most lethal and incapacitating chronic illnesses worldwide.
About the Review:
The present review endeavors to contextualize three common applications of precision medicine (particularly AI implementations) in cancer care:
- Cancer classification and diagnosis,
- Cancer prognostication, and
- The utility of AI chatbots and other sophisticated language model (LLM) technologies in streamlining clinical workflows.
Drawing from an extensive analysis of over 40 primary research studies, the review aims to elucidate policy and implementation enhancements that could further enhance reductions in cancer mortality rates in the years ahead.
Diagnosis:
Cancer diagnosis poses a formidable challenge, particularly in early-stage and recurrent cancers, as patients at these stages often appear clinically asymptomatic to human observers.
AI algorithms, especially those employing machine learning (ML) techniques, trained on vast datasets of cancer diagnostic images (including radiology scans, pathology images, and even patient-provided smartphone photographs), demonstrate efficacy in identifying, categorizing, and diagnosing such cancers, particularly when subtle image data features elude human perception.
Even in cases where human oversight is indispensable, AI technologies, including computer-aided detection (CAD) algorithms, variants of deep learning (DL) frameworks, can pinpoint regions of interest (suspicious pixels in cancer diagnostic images), thereby assisting clinicians in their diagnostic assessments.
Surprisingly, in certain instances, AI algorithms have exhibited superior diagnostic precision and efficiency compared to their human counterparts.
“Commonly used AI algorithms for image classification are convolutional neural networks (CNN), deep learning architectures that extract identifying features for each group and use the resulting schema for a new classification task. The algorithm assigns a probability for each output class, and the image is classified into the group assigned the highest probability. The accuracy of the AI tool is measured by comparing the algorithm classifications with clinician classifications, referred to as “ground truth”.”
Leave a Comment