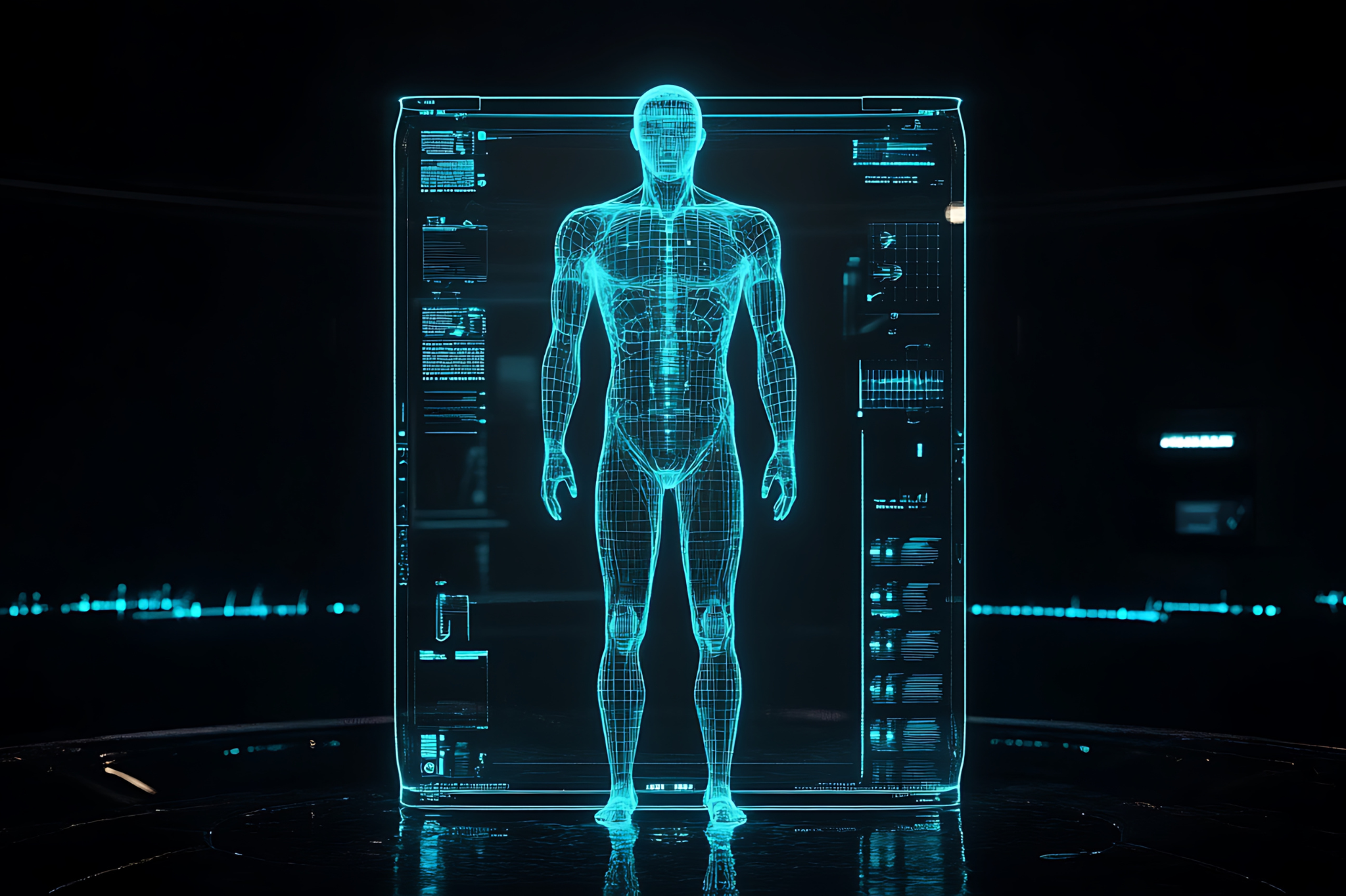
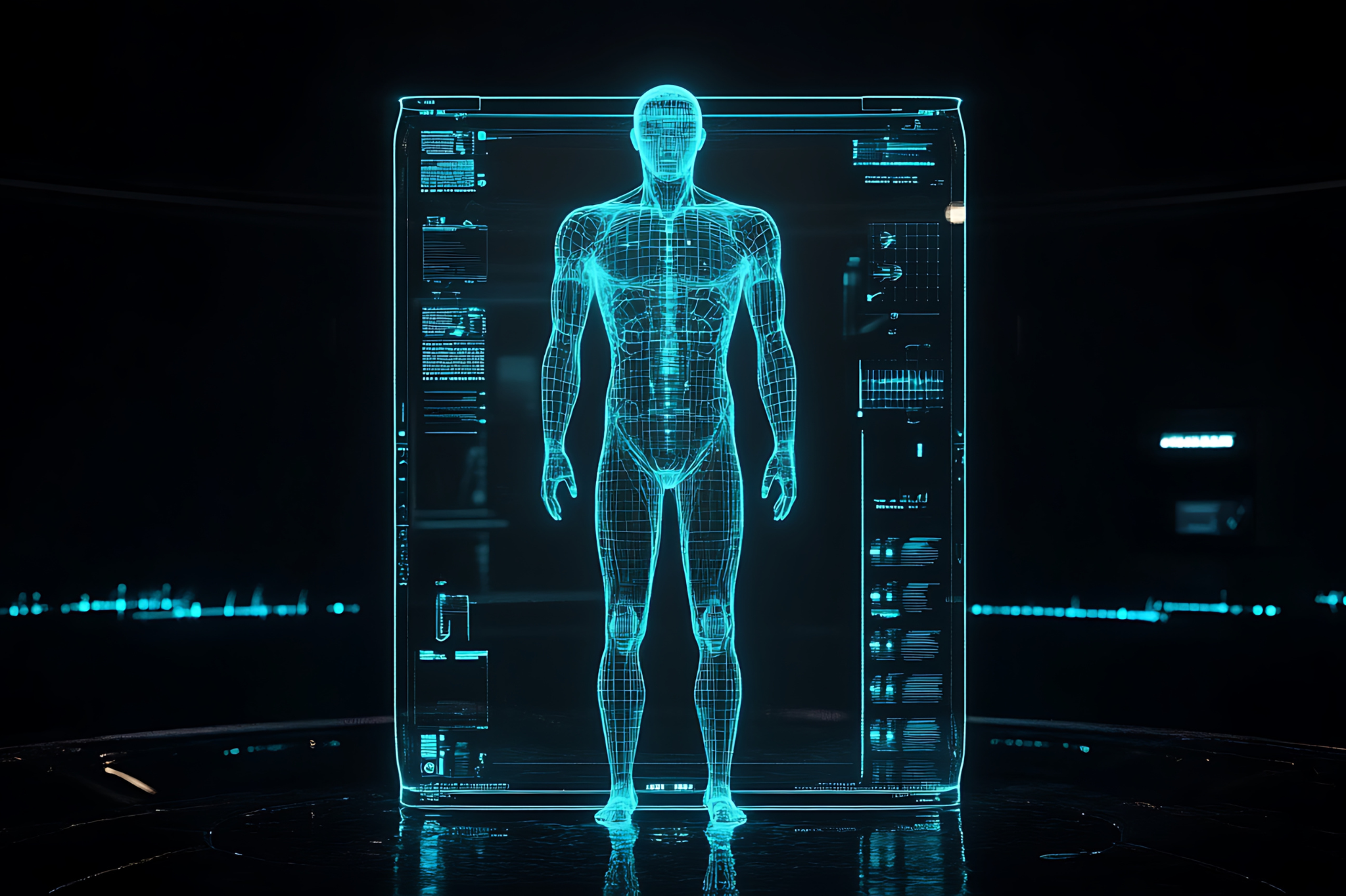
A unique 3D body shape technique promises accessible and reliable body composition forecasts, with the potential to alter how we track health over time and identify hazards.
In a recent study published in the journal npj Digital Medicine, researchers devised a novel method for predicting body composition in three-dimensional (3D) geometries. Body composition is associated with the likelihood of developing chronic diseases. It can be evaluated by computed tomography (CT), dual-energy X-ray absorptiometry (DXA), and magnetic resonance imaging. However, due to ethical and practical limits, these techniques are not widely used in epidemiological studies or clinical practice, and they are not freely accessible to the general public.
Traditional anthropometrics such as waist-hip ratio, body mass index (BMI), and waist-hip circumferences are used to estimate body composition. Nonetheless, these approaches do not distinguish between lean and fat mass, are insufficiently accurate/convenient for long-term use, and frequently necessitate skilled staff and in-person visits. Thus, simple, accessible, and inexpensive techniques are required to reliably analyze body composition.
About the study
In this work, researchers created a new approach for predicting body composition based on 3D body geometry. They received DXA scans, metabolic health indicators, and paired anthropometry data from the Fenland research, which began in 2005. The Fenland research had 12,435 participants in Phase I and 7,795 in Phase II. The current study includes 11,359 Phase I participants and 6,102 from Phase II.
The team used 80% of the Phase I data to train and develop 3D body shape composition models, with the remainder used for validation. Phase II data served as a test dataset for validation in an older population. Furthermore, 119 healthy adults participated in a smartphone validation research that includes DXA scans, air plethysmography, and a mobile app for picture capture. This sample was used to validate models created from the Fenland study and to evaluate the correctness of 3D shapes generated from smartphone photos. Statistical validation metrics such as Pearson correlation coefficients and root-mean-square error (RMSE) were used to assess the accuracy of these predictions.
A mobile software designed to create a 3D body mesh was used to capture 2D photographs of the front, rear, right-side, and left-side profiles. The researchers applied 3D body meshes to DXA silhouettes with paired anthropometry measurements, and the resulting parameters were utilized to estimate body composition metrics. To fit a 3D mesh, DXA silhouettes were augmented with matched anthropometrics using the skinned multi-person linear (SMPL) model in a two-step process.
The hierarchical kinematic probability distributions (HKPD) approach was utilized to estimate starting position and form. The first guess was then refined using an optimization approach. Optimized SMPL form parameters were used to predict body composition measures. A feed-forward neural network was built for regression, with 10 SMPL shape parameters, height, weight, gender, and BMI as inputs. The network outputs included total lean mass, total fat mass, and so on. Furthermore, the HKPD approach created SMPL avatars by combining multi-view information from smartphone photos. Using these methodologies, a model was created that predicts regional and total body composition parameters. The performance was assessed using root-mean-square error values. Pearson correlation coefficients were used to analyze the relationship between anticipated values and DXA measurements.
Findings
The smartphone validation research participants were younger, slimmer, and lighter than those in the Fenland study. The researchers discovered that the optimized meshes matched the DXA silhouette far better than the initial shape and position predictions. In the Fenland study’s Phase I sample, correlation coefficients between DXA and projected parameters were robust for all lean and fat mass variables. Similarly, correlation coefficients were high for all variables in the Phase II sample.
Furthermore, comparable results were obtained in the external validation sample. Most indicators had Pearson correlation coefficients more than 0.86, indicating a high degree of agreement between expected and DXA values. In addition, a comparison study was conducted on several regressor model inputs. One model with simply height and weight as inputs demonstrated some predictive performance. The addition of waist and hip circumferences improved performance. The final model, which took SMPL, height, and weight as inputs, performed significantly better in estimating body composition measures. The model had a root-mean-square error (RMSE) of less than 3.5% for percentage body fat estimates, indicating its accuracy.
The Fenland study included 5,733 participants in both stages, allowing us to assess the model’s capacity to detect changes in body composition across a 6.7-year period. The model detected changes in several fat mass measures; however, lean mass changes were less well recorded, owing to the fact that lean mass remains relatively constant throughout time.fat
Conclusions
The researchers developed a novel computer vision-based method for fitting a 3D body mesh to a DXA silhouette using associated anthropometric data, resulting in a database of 3D body meshes. These meshes correctly predicted body composition measurements. Furthermore, the model detected longitudinal variations. However, the researchers observed that, while the model was particularly good at identifying changes in fat mass, its capacity to track changes in lean mass was limited due to the stability of lean mass over time.
The scientists also demonstrated how avatars created from smartphone photographs may be used to forecast body composition. Overall, 3D body forms created from 2D photos and applicable inference methods have the potential to be a successful clinical medical imaging solution. The paper acknowledges the dataset’s demographic limitations, which primarily included white European adults, and recommends additional research in different populations for greater applicability.
For more information:Qiao C, Rolfe EDL, Mak E, et al. Prediction of total and regional body composition from 3D body shape. npj Digital Medicine, 2024. DOI: 10.1038/s41746-024-01289-0
more recommended stories
Scientists Unveil Next-Gen Eye-Tracking with Unmatched Precision
Eye-tracking technology has long been a.
New Study Questions Fluid Restriction in Heart Failure Management
A groundbreaking study presented at the.
Role of Leptin Signaling in the DMH for Metabolic Regulation
A groundbreaking study from the Pennington.
COVID-19 Vaccines May Lower the Risk of Long COVID by 27%
A recent rapid review suggests that.
3D-Printed Hydrogel for Meniscus Tear Treatment
Meniscus tears are one of the.
Machine Learning Predicts Early Mortality in IBD Patients
A groundbreaking study published in the.
Endometriosis Treatment Advances: Latest Research and Therapy
Recent endometriosis treatment advances are reshaping.
Lung Cancer Screening Gaps Persist Despite Updated Guidelines
A recent study led by researchers.
Altered Knee Movement After ACL Surgery May Trigger Early Osteoarthritis
A recent study published in the.
BRP Peptide for Weight Loss: A Natural Alternative to Ozempic?
The rising obesity epidemic has fueled.
Leave a Comment