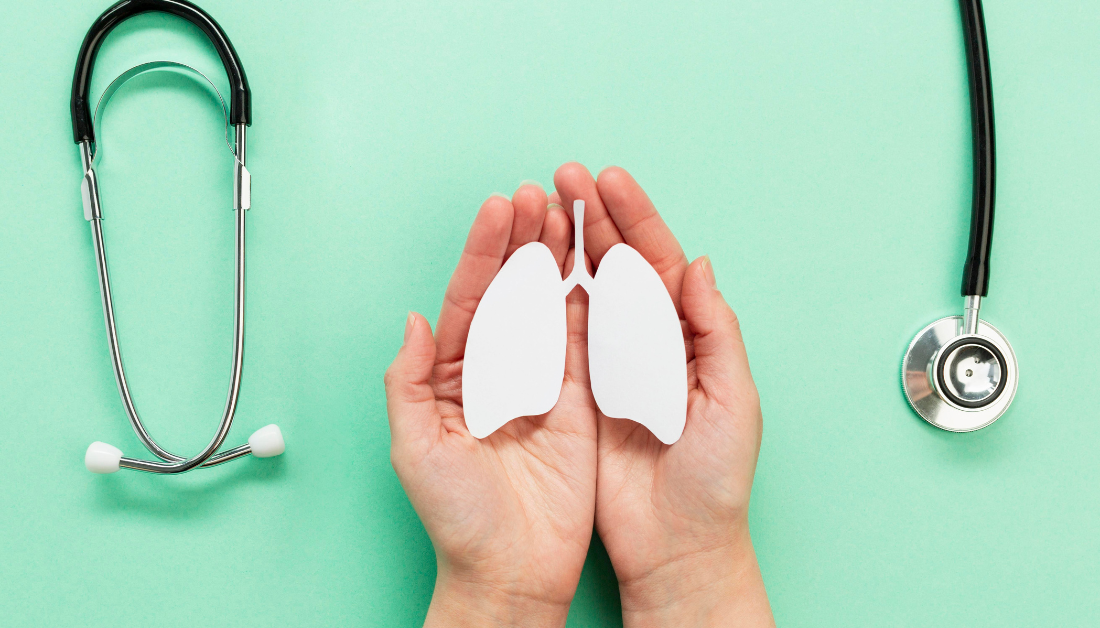
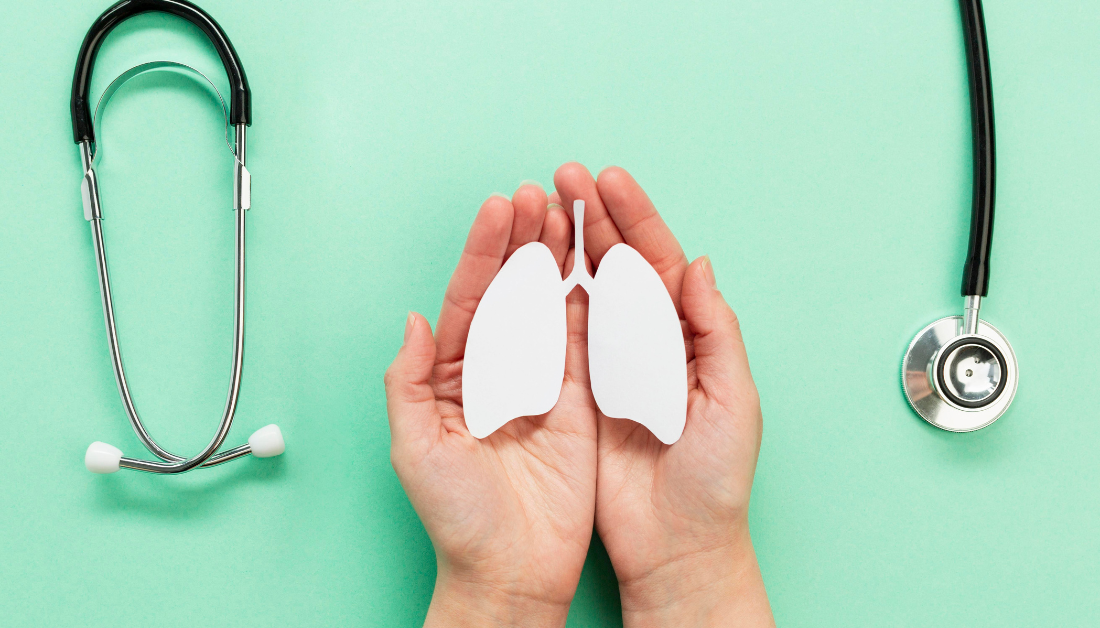
Researchers conducted a meta-analysis in a recent study published in The Lancet Digital Health to assess the quality and efficacy of deep learning and machine learning models for long-term chronic obstructive pulmonary disease (COPD) prognosis. The models were also compared to earlier predictive regression models.
Background
COPD is a leading cause of death worldwide, and the costs of treating it are expected to climb. To save money and improve healthcare delivery efficiency, deep learning and machine learning models are increasingly being utilized to forecast long-term progression in COPD patients.
Deep learning learns sophisticated patterns and relationships using hierarchical structures, whereas machine learning learns rules and correlations from data using algorithms. Deep learning is gaining favor as a method of detecting COPD patients who are at risk of developing complications.
About The Study
Researchers compared the predictive effectiveness of deep learning and machine learning models for COPD in this meta-analysis.
From study inception to 6 April 2023, the team searched the Cochrane Library, PubMed, ProQuest, Embase, Web of Science, and Scopus databases for relevant studies published in English that used deep learning or machine learning to estimate patient outcomes 6.0 months after the initial COPD diagnosis. To discover missing studies, hand searches of included study references and systematic reviews were conducted.
The research team comprised experiments with human volunteers aged 18 to 90 years old with a history of COPD for 6.0 months, allowing for several input modalities.
The research established the AUC values for estimating fatalities, exacerbations, and forced expiratory volume decrease in one second (FEV1). The investigations comprised retrospective and prospective cohort studies, cross-sectional studies, case-control studies, and randomized clinical trials.
Prognostic studies for COPD development in patients who did not already have COPD, as well as prognostic studies that did not contain at least six months of follow-up, were eliminated. Two researchers screened the data separately, and discrepancies were resolved through discussion or consultation with another researcher.
The researchers used Cochran’s Q statistic to quantify heterogeneity (I2 value more than 50% indicated significant heterogeneity). They used the PROBAST and TRIPOD checklists to assess bias risks and reporting quality, respectively.
Support vector machines and random forest analysis were touted as more complex algorithms than regression models that might learn to make decisions based on patterns in data.
Deep learning is defined as the application of neural networks with 2.0 hidden layers. Hospitalization was used to represent aggravation. As surrogate markers for FEV1 decline, changes in six-minute walk test performance, dyspnea, or COPD load as indicated by questionnaires or psychophysical assessments were used.
Results
The researchers first discovered 3,620 studies, of which 18 matched the eligibility criteria, with six using deep learning models and 12 using machine learning models, respectively.
When estimating exacerbations, deep learning and machine learning-based models performed no better than pre-existing illness severity scores. Three research compared machine learning-based models and illness severity ratings for mortality estimation, and five external validation tests found that machine learning-based models performed worse than regression modeling.
The most common sources of bias were mishandling missing information, utilizing too small datasets, and failing to document model uncertainty.
The meta-analysis found no statistically significant difference in pooled AUC between machine learning and traditional regression models (pooled AUC values, 0.77 vs. 0.75).
Although seven models examined exacerbation risks, only six provided AUC values (combined AUC, 0.8). The I2 score of 97% suggested that there was high heterogeneity among the included studies.
Eleven models evaluated fatality risks; however, only six produced AUC values with significant heterogeneity (I2 of 60%) (combined AUC, 0.8). Two studies looked at pulmonary function losses but could not be combined.
AUC 0.8 predicted a drop in pulmonary function of >30 mL over five years on a cohort of 42 persons using five-fold cross-validation, and AUC 0.7 predicted an overall decline in lung volume among 4,496 patient using ten fold nested cross validation.
When estimating exacerbations, deep learning and machine learning-based models performed no better than pre-existing illness severity scores. Three research compared machine learning-based models and illness severity ratings for mortality estimation, and five external validation tests found that machine learning-based models performed worse than regression modeling.
The most common sources of bias were mishandling missing information, utilizing too small datasets, and failing to document model uncertainty.
The meta-analysis found no statistically significant difference in pooled AUC between machine learning and traditional regression models (pooled AUC values, 0.77 vs. 0.75).
There is little evidence that deep learning and machine learning models beat pre-existing COPD severity scores for long-term COPD prediction, according to the study findings. To improve the repeatability of study findings, researchers should use the PROBAST and TRIPOD criteria.
Traditional models have limited practical application due to the large number of variables required. However, there are increasing opportunities for deep learning examination of computed tomography (CT) data in clinical practice.
For more information: Luke A Smith et al., (2023) Machine learning and deep learning predictive models for long-term prognosis in patients with chronic obstructive pulmonary disease: a systematic review and meta-analysis, Lancet Digit Health, doi: https://doi.org/10.1016/S2589-7500(23)00177-2.
more recommended stories
Fentanyl Inhalation: Brain Damage Risks
After treating a middle-aged man who.
Enhanced Immune Response in New COVID-19 Vaccines
An improved vaccination offers an enhanced.
Bioresorbable Wireless Sensor for Early Stomach Leakage Detection
In a recent publication in Scientific.
Neurocardiac Connectivity in Depression Treatment
Heart rate deceleration and sadness may.
Antioxidants: Impact on Quality of Life in Acne Vulgaris
A recent study published in the.
Palliative Care Disparities for Opioid Users: Study Findings
In contrast to individuals devoid of.
Brain Pulsations Linked to High BMI
According to a new study from.
Brain Age Estimation: EEG Advancements in Neurology
To estimate brain age using EEG.
Unlocking Ketogenic Diet for Epilepsy Management
Exploring the Therapeutic Potential of Ketogenic.
Senescence in Neurons: Findings
Based on a new study by.
Leave a Comment