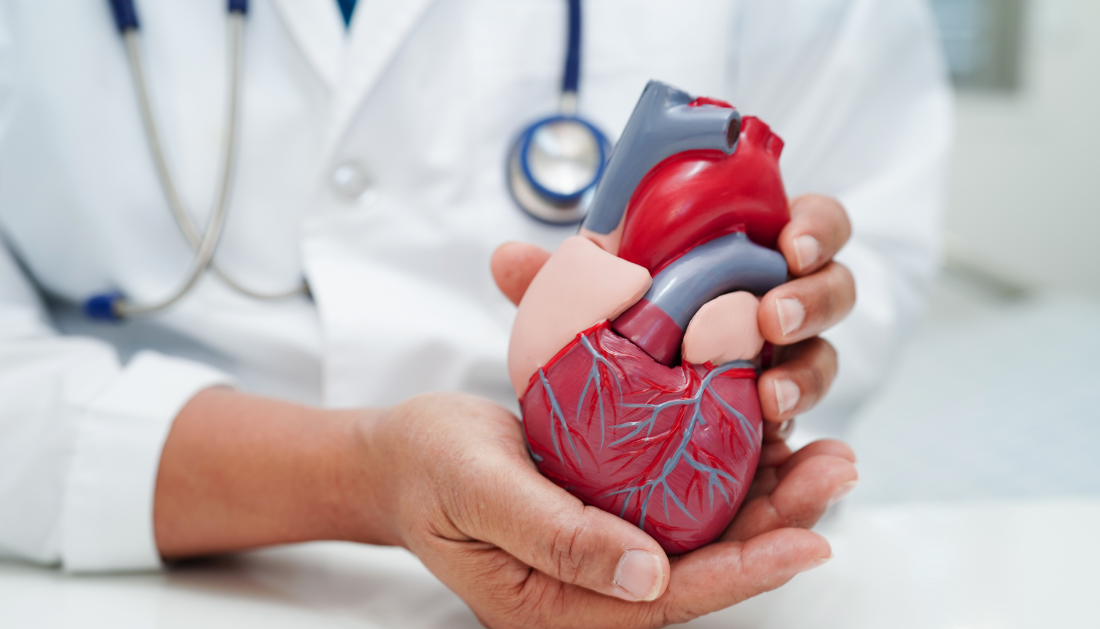
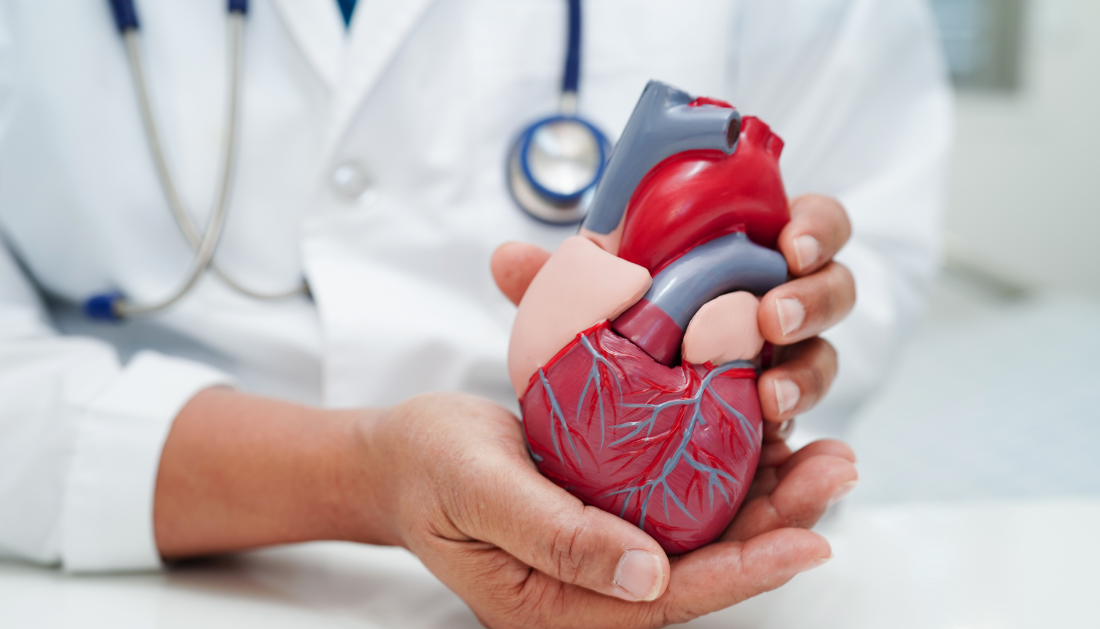
In a recent study published in the journal The Lancet, researchers created and validated a novel artificial intelligence (AI)-enhanced electrocardiography (ECG) model that can use patients’ medical histories and imaging results to accurately predict mortality and cardiovascular disease (CVD) risk.
While not the first attempt to apply AI in disease and mortality prediction, this implementation overcomes the limitations of earlier models in terms of timing, biological plausibility, and explainability, allowing it to create predictions that can enable actionable insights in clinical practice.
The study found that the novel model (known as ‘AIRE’) can reliably predict all-cause mortality, ventricular arrhythmia, atherosclerotic CVD, and heart failure risk.
It outperformed traditional AI models in terms of short- and long-term risk estimations, giving physicians with insights for short-term, single-point diagnostic predictions while also recommending long-term, progressive therapies for the patient’s remaining pharmaceutical support.
Background
Electrocardiograms (ECGs) are non-invasive graphical assessments of cardiovascular electrical activity. The procedure includes placing external electrodes strategically on patients’ chests, arms, and legs to provide clinicians with visual representations of heart electrical signals and rhythms.
ECGs have been used in cardiovascular examinations for over a century, and their methodology has remained mostly unaltered.
Recent developments in computer processing capabilities, as well as the introduction of next-generation predictive machine learning (ML) models, have generated interest in the research community.
Since 2020, a few studies have attempted to use ECG-data-trained artificial intelligence (AI) models to predict patients’ CVD and mortality risk, highlighting model performance: in almost every implementation of AI in disease/mortality risk prediction, AI models achieve diagnostic and predictive performance comparable to, or exceeding, human expert predictions.
Thus, AI models have the potential to reduce patient burdens on clinicians (geographically defined number of individuals per number of doctors), notably in rural and impoverished areas, while also increasing diagnostic speed and lowering patient costs.
Unfortunately, while being clinically validated for safety and efficacy, AI-enhanced ECG models are rarely used in real-world ECG applications.
“Existing mortality prediction models are limited by predicting survival at one or a small number of set timepoints and do not provide information on specific actionable pathways. A high-risk prediction is unhelpful to a clinician if there is no accompanying information on how to improve the survival trajectory of their patient. Thus, making AI-ECG predictions more actionable requires considering time-to-event predictions and specific predictions for diseases with established preventive and disease-modifying treatments.”
From a research standpoint, while accurate, early AI implementations provided insufficient explanations of model performance (a computational ‘black box’) and biological plausibility, prompting physicians to be skeptical of model predictions.
About the Study
In the present study, researchers construct, train, and verify eight innovative AI-ECG risk estimation (AIRE) models (together referred to as the ‘AIRE platform’) aiming at predicting mortality risk (all-cause and cardiovascular) without the restrictions of earlier AI implementations.
Data for the study were collected from five geographically varied sources that received clinical care with minimal overlap. These include the Beth Israel Deaconess Medical Center (BIDMC) cohort (secondary patient care dataset), the São Paulo-Minas Gerais Tropical Medicine Research Center (SaMi-Trop) cohort (chronic Chagas cardiomyopathy dataset), the Longitudinal Study of Adult Health (ELSA-Brasil) cohort (public servants), and the United Kingdom (UK) BioBank (UKB) cohort (voluntary). The Clinical Outcomes in Digital Electrocardiography (CODE) cohort was also used to refine model performance.
The BIDMC cohort was used to construct an AI model. The dataset was randomly divided into three groups: training (50%), validation (10%), and internal testing (40%).
Residual block-based convolutional neural network topologies enabled researchers to use a discrete-time survival method, resulting in patient-specific survival curves that account for both participant mortality and censorship (inability to follow-up).
The CODE cohort data-associated model improvements used 75% of the dataset for model parameter fine-tuning, 5% for generalized (external) validation, and 20% for internal primary care validation.
Using similar methodologies, five more models were developed, focused on CV death (AIRE-CV death), non-CV mortality (AIRE-NCV death), atherosclerotic cardiovascular disease (AIRE-ASCVD), ventricular arrhythmia (AIRE-VA), and heart failure (AIRE-HF).
Statistical analyses were utilized to evaluate model performance, notably in comparison to human expert opinions and the Stanford Estimator of ECG Risk (SEER). Cox models (adjusted for demographics, clinical data, and imaging characteristics) and Kaplan-Meier curves were used to calculate differential model accuracy. PheWAS and GWAS were used to discover significant cardiac and metabolic markers, explaining biological plausibility.
Study Findings
more recommended stories
Role of Leptin Signaling in the DMH for Metabolic Regulation
A groundbreaking study from the Pennington.
COVID-19 Vaccines May Lower the Risk of Long COVID by 27%
A recent rapid review suggests that.
3D-Printed Hydrogel for Meniscus Tear Treatment
Meniscus tears are one of the.
Machine Learning Predicts Early Mortality in IBD Patients
A groundbreaking study published in the.
Endometriosis Treatment Advances: Latest Research and Therapy
Recent endometriosis treatment advances are reshaping.
Lung Cancer Screening Gaps Persist Despite Updated Guidelines
A recent study led by researchers.
Altered Knee Movement After ACL Surgery May Trigger Early Osteoarthritis
A recent study published in the.
BRP Peptide for Weight Loss: A Natural Alternative to Ozempic?
The rising obesity epidemic has fueled.
Toxic Soil and Water Linked to Global Heart Disease Crisis
A groundbreaking review published in Atherosclerosis.
Women’s Health: 195 New Genetic Risks Revealed
In a groundbreaking study published in.
Leave a Comment