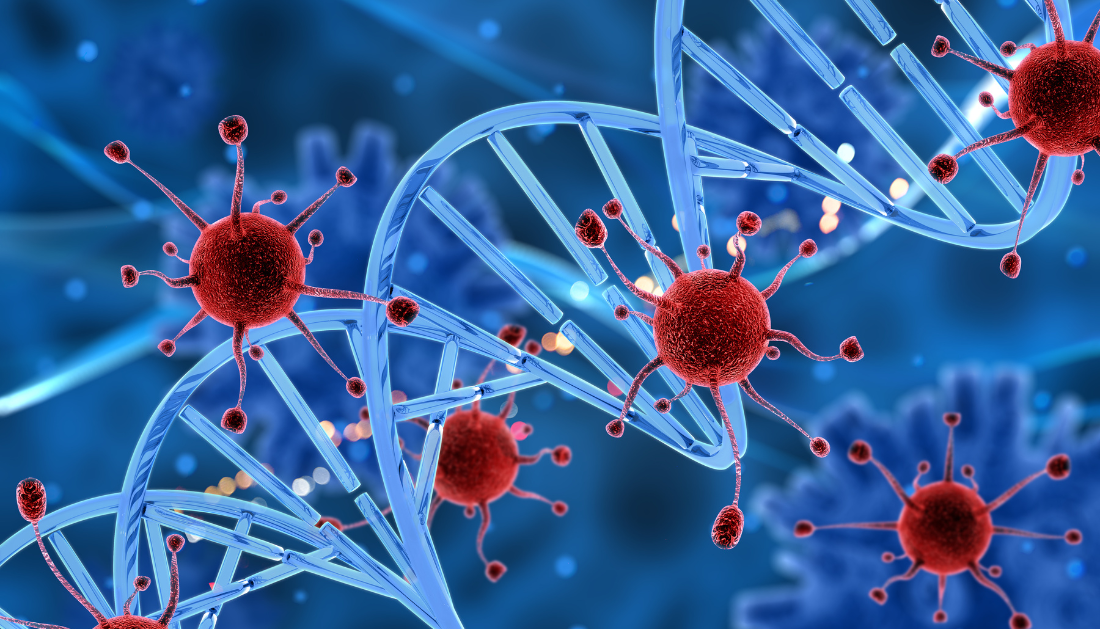
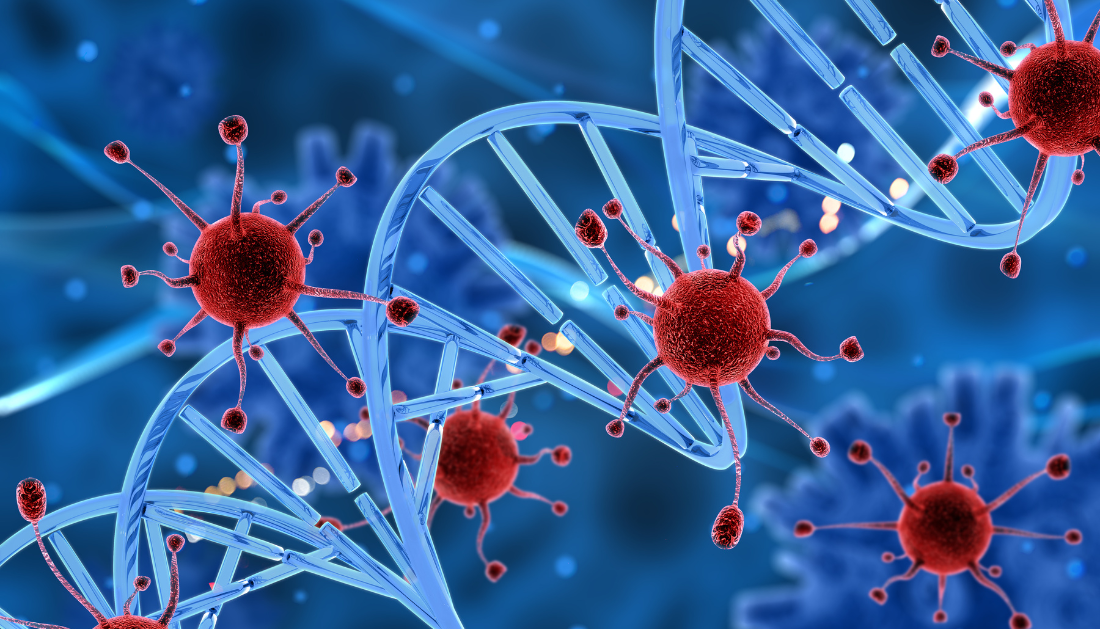
Doctors may be able to assess if immunotherapy medications help treat a patient’s cancer with the aid of the machine-learning model. Researchers at Memorial Sloan Kettering Cancer Center in New York and the National Cancer Institute’s (NCI) Center for Cancer Research led the study, which was published June 3, 2024, in Nature Cancer. The National Institutes of Health includes NCI.
The Food and Drug Administration has currently approved the use of two predictive biomarkers to help identify patients who might benefit from immune checkpoint inhibitor medication. Tumor mutational load, or the quantity of mutations in cancer cells’ DNA, is the first. The other is PD-L1, a protein in tumor cells that suppresses the immune system and is targeted by various immune checkpoint inhibitors.
These biomarkers do not, however, always correctly indicate how the body will react to immune checkpoint inhibitors. Although response can be accurately predicted by recent machine-learning algorithms that incorporate molecular sequencing data, this type of data is costly to acquire and is not frequently collected.
A distinct type of machine-learning model is described in the new study, which bases its predictions on five clinical features routinely obtained from patients: blood albumin level, age, cancer type, history of systemic therapy, and blood neutrophil-to-lymphocyte ratio, which is a measure of inflammation.
The tumor mutational burden, as determined by sequencing panels, is also taken into account by the model. Data from several different data sets, comprising 2,881 individuals treated with immune checkpoint inhibitors spanning 18 solid tumor types, were used to build and assess the model.
The model correctly predicted the total survival time as well as the time until the disease recurred for each patient, the time until the disease recurred for each patient and the anoint inhibitor. Interestingly, according to the researchers, the approach could also identify patients who could still benefit from immunotherapy despite having a low tumor mutational burden.
Larger prospective trials are required, according to the researchers, to fully assess the AI model in clinical settings. The Logistic Regression-Based Immunotherapy-Response Score (LORIS), an AI model, is now accessible to the general public. The program uses information on the six variables mentioned above to assess the probability that a patient would respond to immune checkpoint inhibitors.
Luc G. T. Morris, M.D., of Memorial Sloan Kettering Cancer Center, and Eytan Ruppin, M.D., Ph.D., of NCI’s Center for Cancer Research co-led the study. Tiangen Chang, Ph.D., and Yingying Cao, Ph.D., from Dr. Ruppin’s group at the NCI’s Center for Cancer Research, led the effort.
For more information: LORIS robustly predicts patient outcomes with immune checkpoint blockade therapy using common clinical, pathologic, and genomic features, nature cancer, DOI: 10.1038/s43018-024-00772-7
more recommended stories
COPD Mucus Plugs Accelerate Lung Decline
Chronic obstructive pulmonary disease (COPD) remains.
Lipid Accumulation Product Predicts Bone Loss
A new study reveals that lipid.
AI-Designed COVID Vaccine Antigens
As SARS-CoV-2 continues to evolve, existing.
Multi-Cancer Early Detection Test Saves Lives
A groundbreaking multi-cancer early detection test.
Breath Sensor for Chronic Kidney Disease Detection
In a groundbreaking development, researchers have.
Vagus Nerve Stimulation for PTSD Relief
A pioneering clinical study from The.
Gut Microbiota Diet Score and Infertility: Surprising Link Revealed
Can what you eat really impact.
Lung Cancer Outcomes Linked to PSG Genes in Women
Researchers at Memorial Sloan Kettering Cancer.
Osteoarthritis Genetic Targets Identified in Largest Study
In a groundbreaking achievement, researchers have.
lncRNAs in Tumor Microenvironment Drive Cancer Progression
The tumor microenvironment (TME) is a.
Leave a Comment