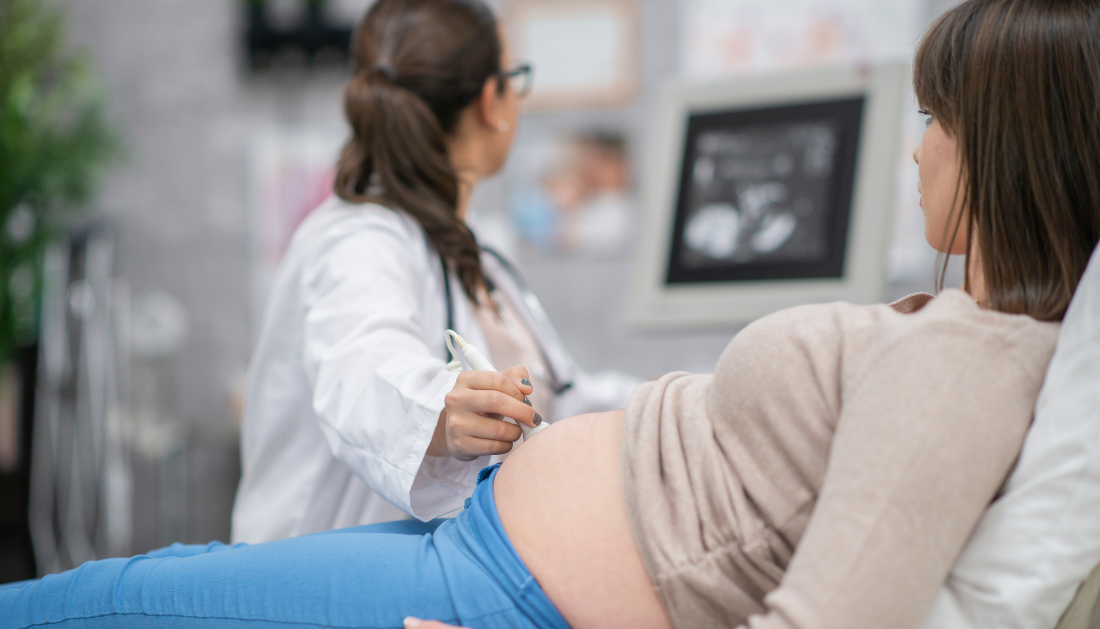
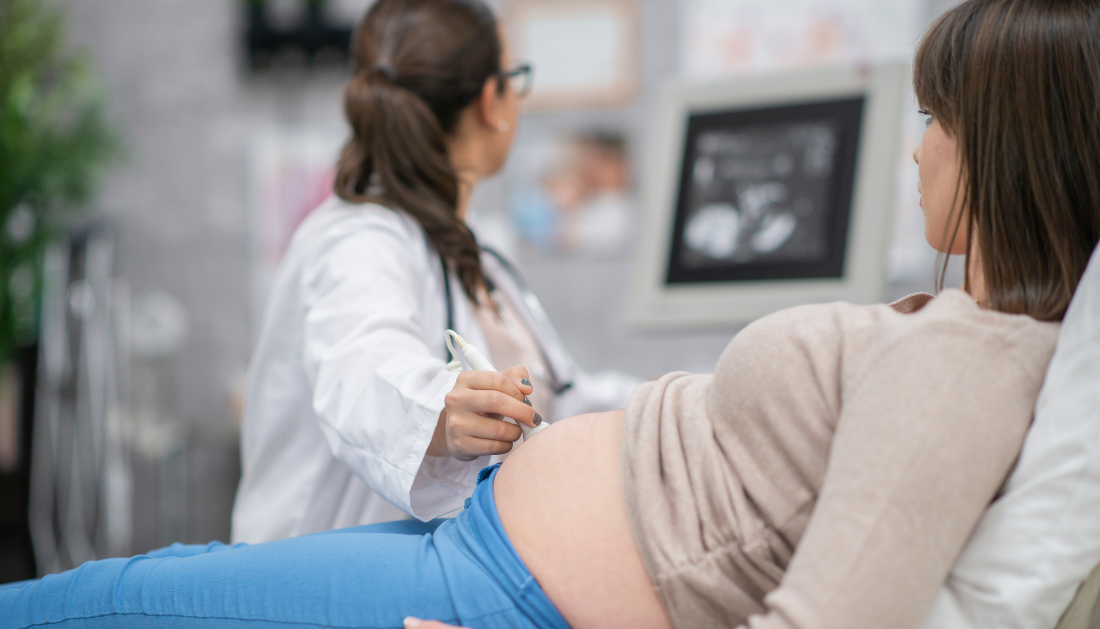
A groundbreaking AI-driven study has identified previously unknown risk factors linked to stillbirth and severe newborn complications, offering a new level of personalized pregnancy risk assessment. The research, published in BMC Pregnancy and Childbirth, analyzed nearly 10,000 pregnancies and uncovered unexpected correlations between maternal conditions, fetal characteristics, and adverse outcomes.
Uncovering Unexpected Pregnancy Risks
The study utilized explainable AI, a model designed to analyze complex datasets while providing transparent, interpretable insights. Researchers from the University of Utah Health Sciences examined data from 9,558 pregnancies, evaluating various maternal and fetal health indicators, including blood pressure, social factors, medical history, and fetal weight. Their goal was to identify new risk patterns that could refine pregnancy monitoring and intervention strategies.
A surprising finding emerged: while female fetuses are generally at lower risk for complications such as stillbirth, those born to mothers with pre-existing diabetes faced a higher risk than males. This contradiction to established medical assumptions highlights the AI model’s potential to reveal risks such as stillbirth that even the most experienced clinicians might overlook.
Varying Risk in Low-Weight Fetuses
One of the study’s most compelling insights involved small fetuses—specifically those in the bottom 10% for weight but not the lowest 3%. While these babies often develop normally, current medical guidelines recommend intensive monitoring for all cases, causing emotional and financial strain for families.
The AI model revealed that risk levels in these cases vary widely, from no higher than an average pregnancy to nearly tenfold the normal risk. Factors such as fetal sex, maternal diabetes, and fetal anomalies like heart defects played key roles in determining outcomes. This finding suggests that current clinical guidelines could be refined to provide more tailored, evidence-based recommendations.
Why AI Matters in Pregnancy Risk Assessment
Pregnancy care requires weighing countless variables, from ultrasound data to maternal health history. While experienced physicians make complex decisions based on intuition and expertise, human factors like bias, fatigue, and inconsistency can affect judgment.
The explainable AI model used in this study provides precise, transparent, and reproducible risk assessments, helping doctors make data-driven decisions that are less prone to error. Unlike traditional “black box” AI models, this system clearly shows how it reaches conclusions, making it easier to address potential biases and refine predictions.
Transforming Maternal and Fetal Care
This AI-based approach has the potential to revolutionize pregnancy risk assessment by identifying individualized risk levels rather than relying on broad guidelines. However, further validation in diverse populations is needed before clinical implementation.
Dr. Nathan Blue, senior author of the study and assistant professor of obstetrics and gynecology at the Spencer Fox Eccles School of Medicine, remains optimistic:
“AI models can estimate risks specific to a person’s unique context—transparently and reproducibly. This could transform maternal and fetal healthcare as we know it.”
As AI continues to advance, its role in personalized pregnancy care could lead to earlier interventions, fewer unnecessary procedures, and better outcomes for both mothers and babies.
More Information: AI-based analysis of fetal growth restriction in a prospective obstetric cohort quantifies compound risks for perinatal morbidity and mortality and identifies previously unrecognized high risk clinical scenarios, BMC Pregnancy and Childbirth (2025). DOI: 10.1186/s12884-024-07095-6
more recommended stories
Caffeine and SIDS: A New Prevention Theory
For the first time in decades,.
Microbial Metabolites Reveal Health Insights
The human body is not just.
Reelin and Cocaine Addiction: A Breakthrough Study
A groundbreaking study from the University.
Preeclampsia and Stroke Risk: Long-Term Effects
Preeclampsia (PE) – a hypertensive disorder.
Statins and Depression: No Added Benefit
What Are Statins Used For? Statins.
Azithromycin Resistance Rises After Mass Treatment
Mass drug administration (MDA) of azithromycin.
Generative AI in Health Campaigns: A Game-Changer
Mass media campaigns have long been.
Molecular Stress in Aging Neurons Explained
As the population ages, scientists are.
Higher BMI and Hypothyroidism Risk Study
A major longitudinal study from Canada.
Therapeutic Plasma Exchange Reduces Biological Age
Therapeutic plasma exchange (TPE), especially when.
Leave a Comment