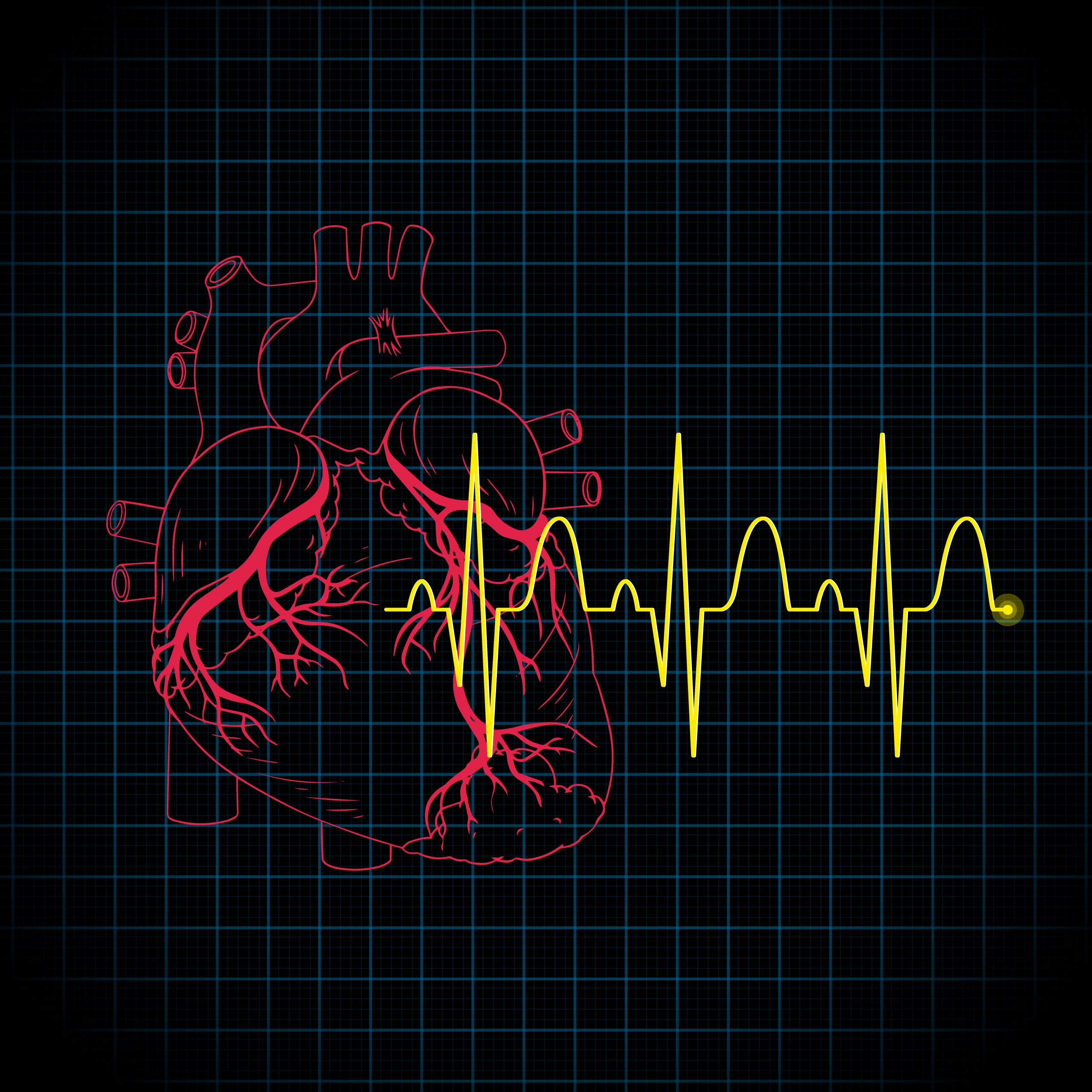
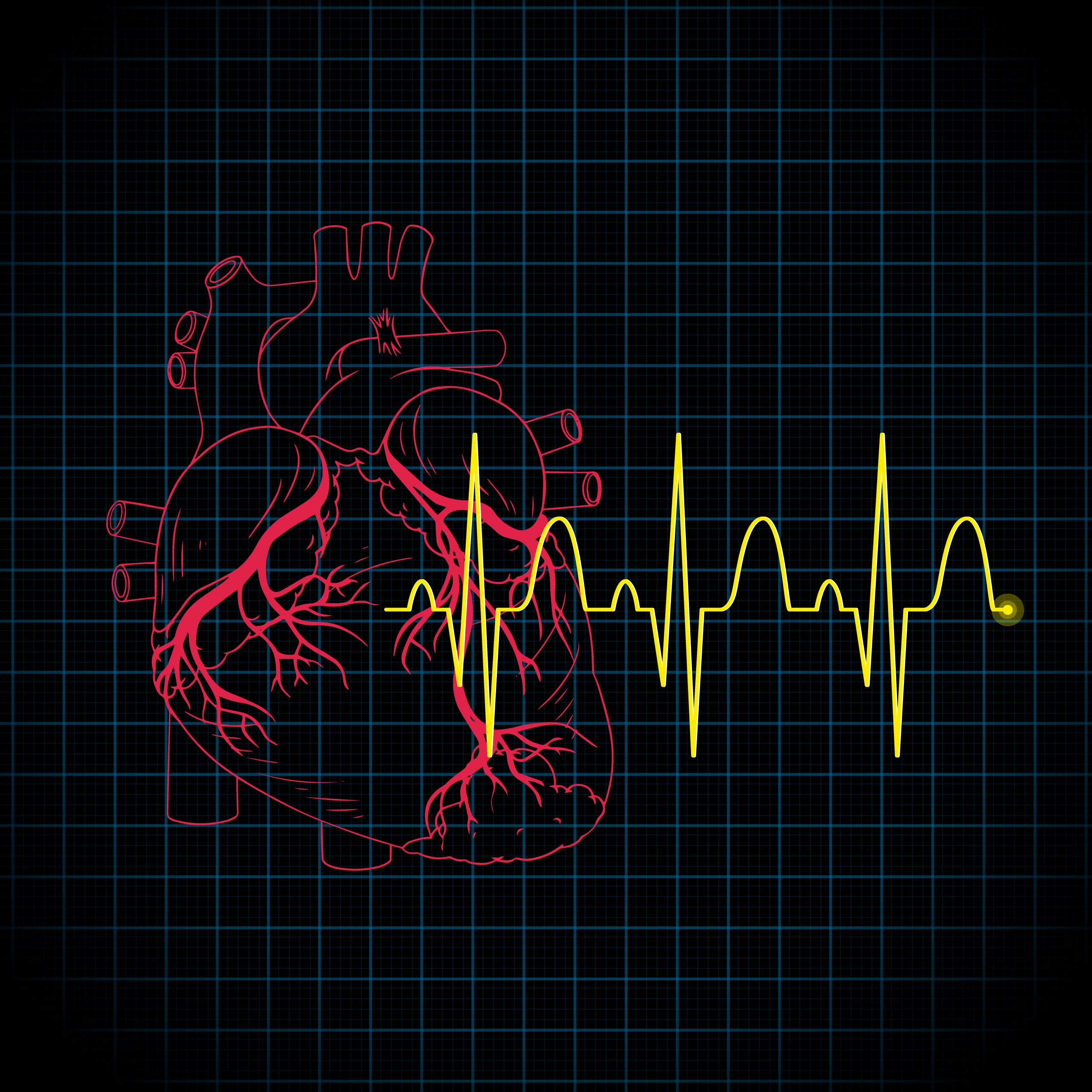
According to a study headed by University of Pittsburgh researchers and published in Nature Medicine, a novel machine learning model uses electrocardiogram (ECG) measurements to detect and classify heart attack faster and more precisely than current approaches.
“When a patient comes into the hospital with chest pain, the first question we ask is whether the patient is having a heart attack or not. It seems like that should be straightforward, but when it’s not clear from the ECG, it can take up to 24 hours to complete additional tests,” said lead author Salah Al-Zaiti, Ph.D., R.N., associate professor in the Pitt School of Nursing and of emergency medicine and cardiology in the School of Medicine. “Our model helps address this major challenge by improving risk assessment so that patients can get appropriate care without delay.”
Clinicians can clearly identify a unique pattern within the peaks and valleys of an ECG that suggests the worst type of heart attack known as STEMI. These acute episodes are caused by a complete blockage of a coronary artery and necessitate rapid treatment to restore blood flow.
The issue is that nearly two-thirds of heart attacks are caused by significant blockage but lack the recognizable ECG signal. The new technique detects small signs in the ECG that physicians may miss and improves classification of patients with chest discomfort.
The model was developed with ECGs from 4,026 patients with chest pain at three Pittsburgh hospitals by co-author Ervin Sejdi, Ph.D., associate professor at The Edward S. Rogers Department of Electrical and Computer Engineering at the University of Toronto and the Research Chair in Artificial Intelligence for Health Outcomes at North York General Hospital in Toronto. The model was then verified externally on 3,287 patients from a separate hospital system.
The researchers compared their model to three gold standards for assessing cardiac events: experienced clinician interpretation of ECG, commercial ECG algorithms, and the HEART score, which takes into account the patient’s history (including pain and other symptoms), ECG interpretation, age, risk factors (such as smoking, diabetes, and high cholesterol), and blood levels of a protein called troponin.
The algorithm outperformed the other two, correctly categorizing one out of every three individuals with chest discomfort as low, middle, or high risk.
In our wildest dreams, we hoped to match the accuracy of HEART, but we were surprised to find that our machine learning model based solely on ECG exceeded this score,” said Al-Zaiti.
The algorithm will help EMS personnel and emergency department providers identify people having a heart attack and those with reduced blood flow to the heart in a much more robust way than traditional ECG analysis, according to co-author Christian Martin-Gill, M.D., M.P.H., chief of the Emergency Medical Services (EMS) division at UPMC.
“This information can help guide EMS medical decisions such as initiating certain treatments in the field or alerting hospitals that a high-risk patient is incoming,” Martin-Gill added. “On the flip side, it’s also exciting that it can help identify low-risk patients who don’t need to go to a hospital with a specialized cardiac facility, which could improve prehospital triage.”
The team is currently optimizing how the model will be deployed in collaboration with the City of Pittsburgh Bureau of Emergency Medical Services in the next phase of this project. According to Al-Zaiti, they are working on a cloud-based system that will interact with hospital command centers that get ECG readings from EMS. The model will analyze the patient’s ECG and report back a risk rating, directing medical decisions in real-time.
more recommended stories
Efficient AI-Driven Custom Protein Design Method
Protein design seeks to develop personalized.
Human Cell Atlas: Mapping Biology for Precision Medicine
In a recent perspective article published.
Preterm Birth Linked to Higher Mortality Risk
A new study from Wake Forest.
Heart Failure Risk Related to Obesity reduced by Tirzepatide
Tirzepatide, a weight-loss and diabetes medicine,.
Antibiotic Activity Altered by Nanoplastics
Antibiotic adsorption on micro- and nano-plastics.
Cocoa Flavonols: Combat Stress & Boost Vascular Health
Cocoa Flavonols on combatting Stress: Stress.
AI Predicts Triple-Negative Breast Cancer Prognosis
Researchers at Sweden’s Karolinska Institutet explored.
Music Therapy: A Breakthrough in Dementia Care?
‘Severe’ or ‘advanced’ dementia is a.
FasL Inhibitor Asunercept Speeds COVID-19 Recovery
A new clinical trial demonstrates that.
Gut Health and Disease is related to microbial load
When it comes to Gut Health,.
Leave a Comment