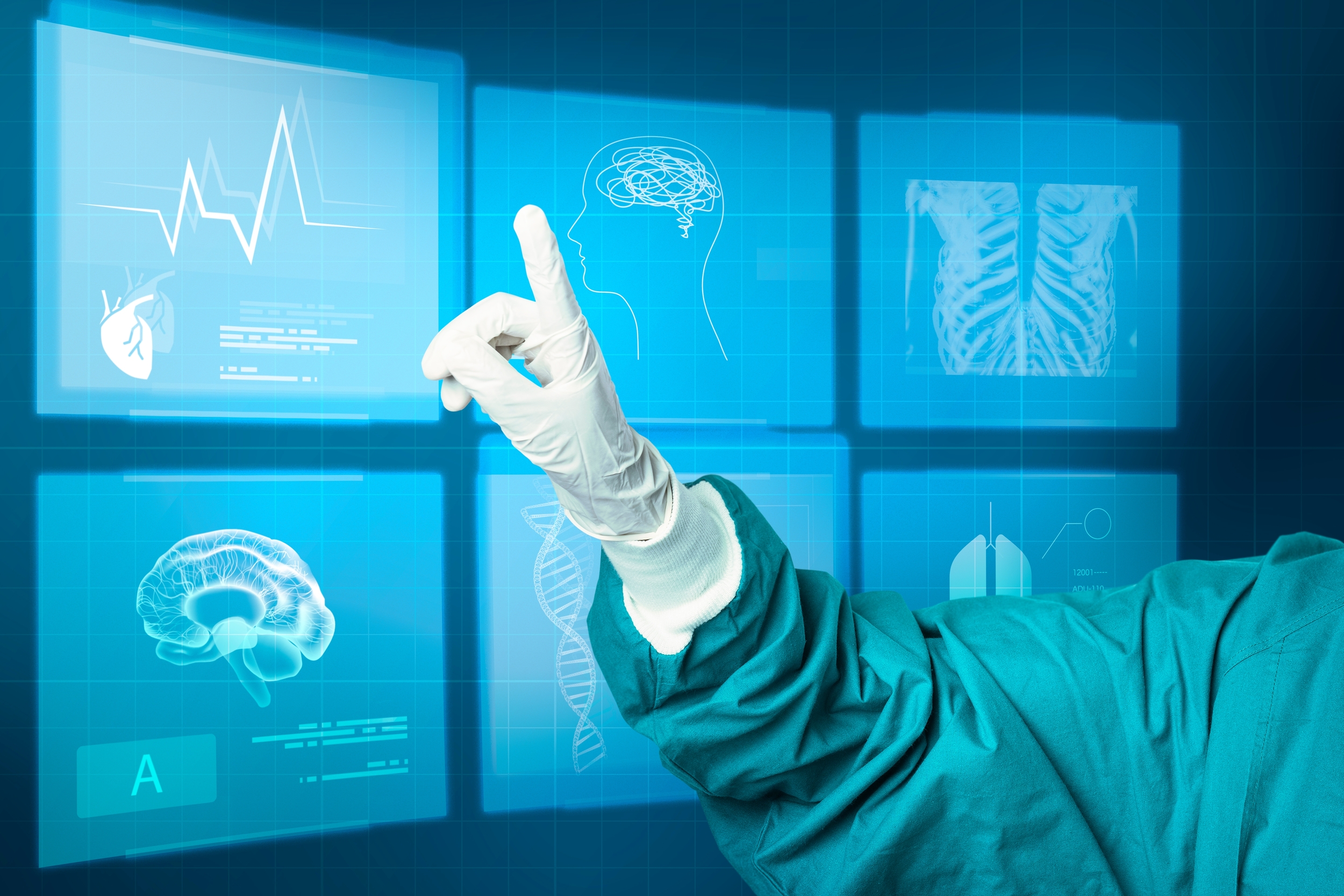
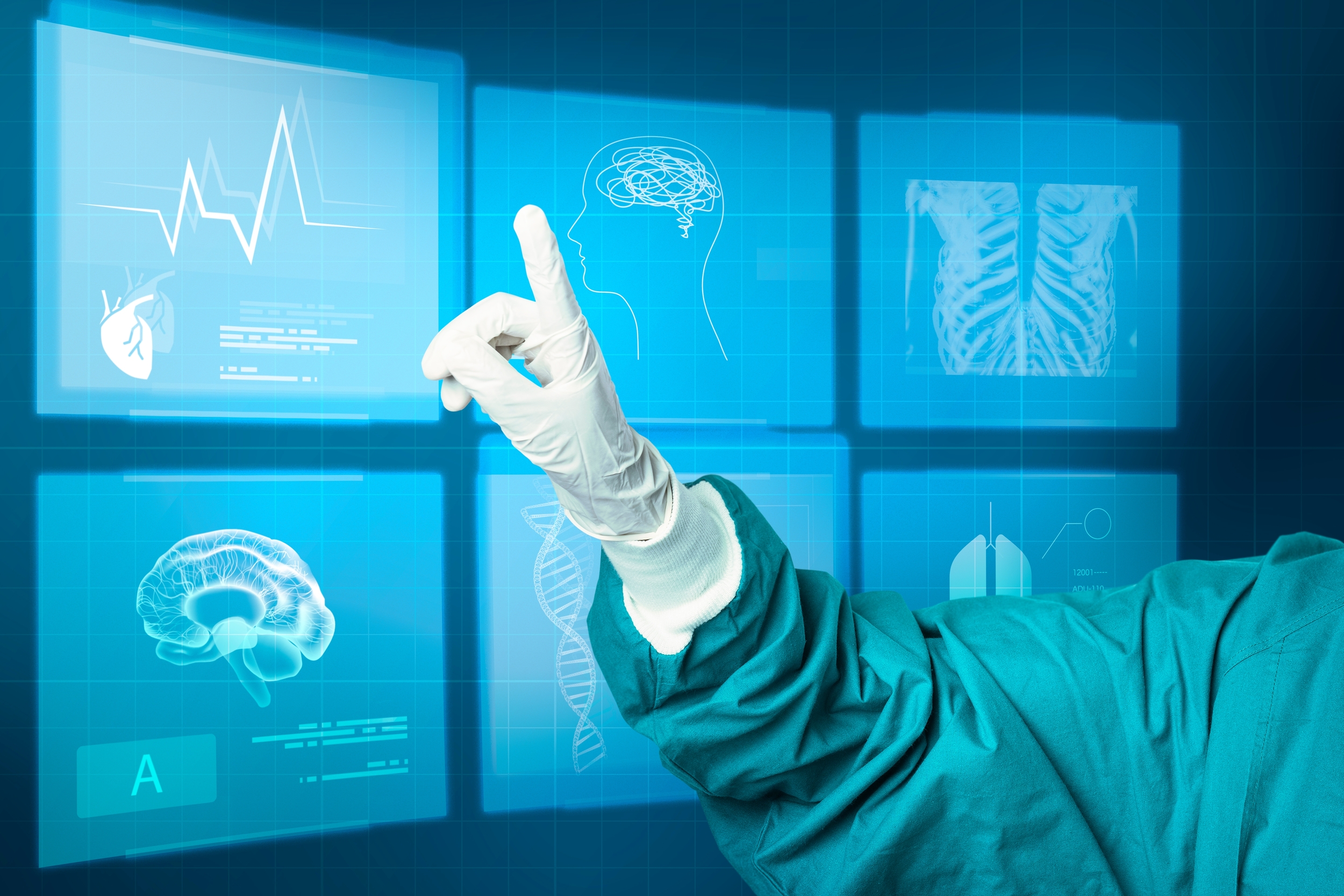
Medical imaging is critical in the diagnosis and treatment of a wide range of illnesses. From X-rays to determine if a bone is broken or a cavity in a tooth to SPECT scans to detect heart problems, clinicians utilize medical imaging to look inside the body, discover disease, and treat it. But what happens when the pictures are hazy?
Recent breakthroughs in artificial intelligence have paved the way for the use of AI-based approaches for denoising, or image cleaning. However, before these tools can be used in clinical settings to provide real-world patient care, they must be rigorously evaluated, according to Abhinav Jha, assistant professor of biomedical engineering in the McKelvey School of Engineering and radiology at the Mallinckrodt Institute of Radiology (MIR) in the School of Medicine at Washington University in St. Louis.
Jha and colleagues at MIR examined a commonly used AI-based solution to denoise cardiac SPECT pictures in a research published in Medical Physics. The approach’s performance was evaluated in two ways: how aesthetically comparable denoised images were to normal images, and how well the denoised image performed in the clinically relevant task of detecting heart abnormalities.
“Rather alarmingly, while the visual-similarity-based metrics suggested that the AI-based denoising technique improved performance, it was actually having no significant impact, and in some cases, it was even degrading performance on clinical tasks,” Jha said. “This emphasizes the important need for performing evaluation of AI algorithms on clinical tasks and not just relying on visual similarity as a measure of performance.”
First author Zitong Yu, a PhD student in Jha’s group, discovered that the AI denoising technique tended to smooth down cardiac SPECT pictures, which reduced noise as planned but also diminished the contrast of the heart defect needed by doctors to make correct diagnosis. “This is precisely what we want to prevent from happening in actual medical practice,” Yu said.
The study advocates for task-based evaluation of AI-based denoising methods to assess the usefulness of AI-processed images. “Ensuring AI-based denoising works well for real clinical tasks—not just aesthetically—would mean big benefits for patients by producing high-quality images in less time or with reduced radiation doses,” said collaborator Robert J. Gropler, professor of radiology and senior vice chair and division director of radiological sciences at MIR.
more recommended stories
Osteoarthritis Genetics Study Uncovers New Treatment Hope
Osteoarthritis- the world’s leading cause of.
Antibody Breakthrough in Whooping Cough Vaccine
Whooping cough vaccine development is entering.
Scientists Unveil Next-Gen Eye-Tracking with Unmatched Precision
Eye-tracking technology has long been a.
Men5CV: Hope for Ending Africa’s Meningitis Epidemics
A landmark global health study led.
Stem Cell Therapy Shows 92% Success in Corneal Repair
A groundbreaking stem cell therapy known.
Gene Therapy for Maple Syrup Urine Disease
Researchers at UMass Chan Medical School.
How Fast Are Your Organs Aging? Simple Blood Test May Tell
New research from University College London.
HEALEY Platform Accelerates ALS Therapy Research
A New Era of ALS Clinical.
Low-Oxygen Therapy in a HypoxyStat Pill? Scientists Say It’s Possible
A New Approach to Oxygen Regulation-HypoxyStat.
Early Alzheimer’s Diagnosis with Advanced Tau Test
A New Biomarker Test Promises Early.
Leave a Comment