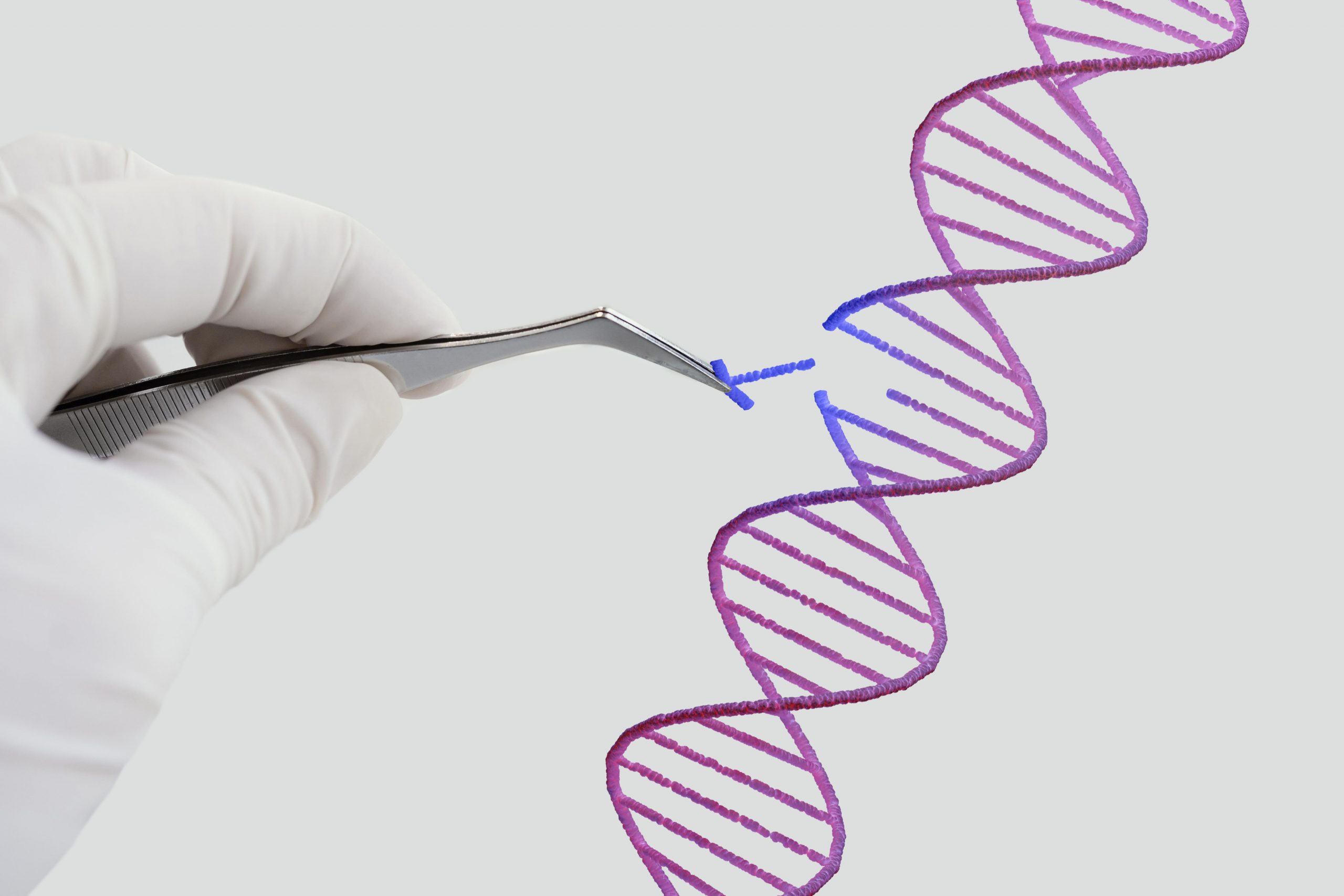
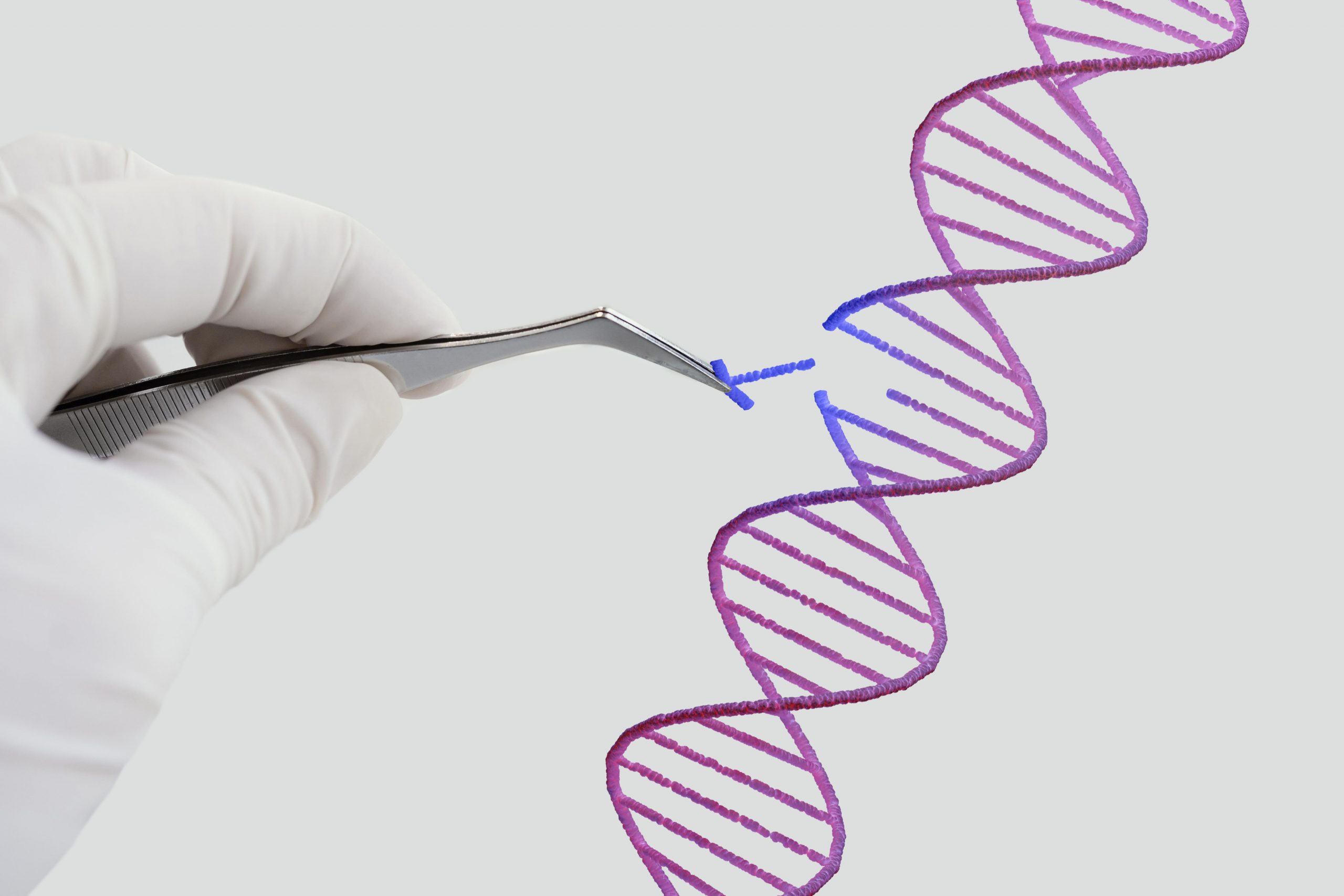
Human cell culture innovations could assist accelerate the development of cellular therapy, a field of regenerative medicine that addresses diseases that are now incurable.
Julie Audet, a professor in the Institute of Biomedical Engineering in the Faculty of Applied Science & Engineering at the University of Toronto, is working to address some of the most critical obstacles associated with producing therapeutic cells.
Her cell and tissue engineering study aims to improve the therapeutic characteristics of lab-grown human cells so that they are ready for clinical use.
Audet and her colleagues are creating complex computational algorithms to help university and industrial researchers optimize laboratory experiments. These instruments will enable researchers to provide the ideal circumstances for therapeutic cell cultivation.
Cellular therapy treats a number of diseases and ailments by transferring improved human cells into the body to replace or repair damaged tissue and cells – a strategy known as a living medication.
“But before cells can be transplanted into a patient, we want to ensure that the cells are not contaminated with compounds that can trigger an adverse reaction,” Audet says.
“We also need to enhance the therapeutic properties of the cells in a culture process to effect a positive medical outcome.”
When developing cell-culture experiments, there are numerous things to consider. There are numerous pricey reagents, for example, to select and optimize at various concentrations – these chemicals are employed to assess chemical reactions carried out by the cell. There are also major technical and biological differences to consider: cells from different donors do not always behave similarly in culture.
“Our algorithms become necessary when experiments are very costly to execute and are extremely labour-intensive,” Audet says.
“They are especially useful when the results are impossible to predict because of the complexity of the biological systems under study. In that case, it would not be feasible or possible for researchers to use conventional approaches to design and execute their experiments.”
Audet and her team may use advanced computer techniques based on machine learning to design tests that are not only feasible and have a higher chance of success, but are also less expensive, requiring less resources to execute.
Audet’s lab used an earlier prototype of similar algorithms to create a serum-free T-cell medium to treat blood diseases. Craig Simmons, a professor in the Institute of Biomedical Engineering and the department of mechanical and industrial engineering in the Faculty of Applied Science & Engineering, and Neal Callaghan, a PhD graduate from the institute and a former researcher in Simmons’ lab, used the algorithm to develop culture media for cardiomyocytes (cardiac muscle cells), which is now being commercialized.
“Cardiac tissue engineering is an important application for our tools because when it comes to heart failure and heart disease, there are many conditions that can’t currently be cured,” Audet says.
“Cellular therapy offers a promising approach to treat heart failure and other cardiac ailments.”
Audet is collaborating with Sowmya Viswanathan, an assistant professor in the Institute of Biomedical Engineering and the Temerty Faculty of Medicine, and a researcher at the University Health Network’s Krembil Research Institute.
Viswanathan is working on cellular therapeutics for osteoarthritis, a chronic inflammatory condition, that use mesenchymal stromal cells.
“We have seen that different mesenchymal stromal cell donors prefer different growth conditions,” Viswanathan says. “This algorithm helps us identify optimal conditions for different donors without doing a full set of detailed experiments.”
Her cooperation with Audet aims to create categories of illnesses that can be linked to different groups of donors based on genetic characteristics.
Oreoluwa Kolade, a PhD candidate at the Institute of Biomedical Engineering and a fellow at the Data Sciences Institute – one of several U of T institutional strategic initiatives – whose work combines tissue engineering with data science, is co-supervised by Audet and Viswanathan.
“My research involves the statistical design of experiments, looking at the numerous factors that can impact the cell expansion process, such as oxygen levels, cell density and medium composition,” Kolade says.
“If a researcher wants to see which factors maximize the therapeutic quality of their cells, it isn’t cost-effective to test all possible combinations. So we are trying to design experiments in such a way that researchers can get the highest impact when they see their results.”
This methodology would enable researchers to narrow down the many elements and run combinations through a simulation model to determine which experiments would be required to obtain the best cells.
“We are currently working on the commercialization of the latest version of one of the algorithms that includes these machine-learning modules to help design experiments,” Audet says.
“We hope this tool will help make cellular therapies both widely available and more accessible by accelerating the development of these therapies while increasing the effectiveness of the enhanced cells.”
more recommended stories
Caffeine and SIDS: A New Prevention Theory
For the first time in decades,.
Microbial Metabolites Reveal Health Insights
The human body is not just.
Reelin and Cocaine Addiction: A Breakthrough Study
A groundbreaking study from the University.
Preeclampsia and Stroke Risk: Long-Term Effects
Preeclampsia (PE) – a hypertensive disorder.
Statins and Depression: No Added Benefit
What Are Statins Used For? Statins.
Azithromycin Resistance Rises After Mass Treatment
Mass drug administration (MDA) of azithromycin.
Generative AI in Health Campaigns: A Game-Changer
Mass media campaigns have long been.
Molecular Stress in Aging Neurons Explained
As the population ages, scientists are.
Higher BMI and Hypothyroidism Risk Study
A major longitudinal study from Canada.
Therapeutic Plasma Exchange Reduces Biological Age
Therapeutic plasma exchange (TPE), especially when.
Leave a Comment