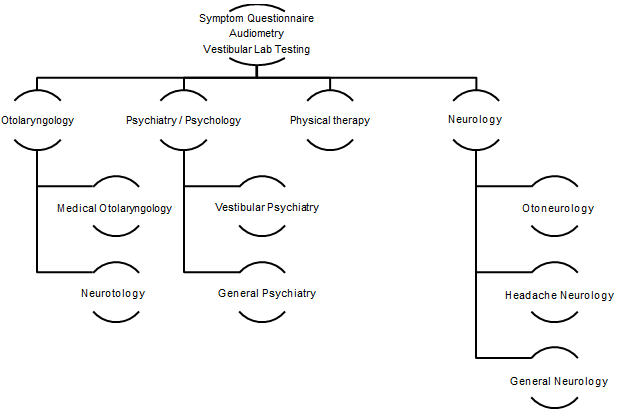
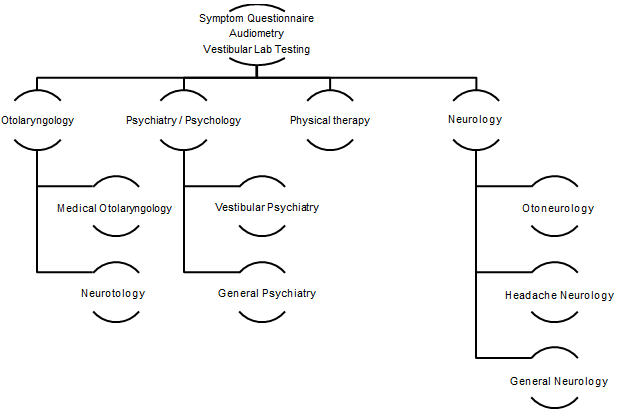
In this edition of “Striking the Right Balance,” Adam Goulson, AuD, and Devin McCaslin, Ph.D. write about how their clinic is using artificial intelligence to help triage and manage their chronic dizzy patients.
Michael Vekasi, AuD, R.Aud, Aud(C), FAAA, and Erica Zaia, MSc, RAUD are coordinating the “Striking the Right Balance,” feature which will cover the latest information on ‘all things vestibular.’
If you would like to be more involved in all things vestibular, please sign-up for the Vestibular Special Interest Group. Sign-up by simply emailing ericazaia@hotmail.com to let us know you want to be a part of. Also, check out our Facebook page for a free list of online vestibular resources at the CAA National Vestibular Special Interest Group page.
It has been reported that dizziness is the third most common complaint about outpatient primary care visits. Dizziness can mean many different things to those experiencing symptoms. For example, their dizziness could be a false sensation of motion either externally or internally. For others, dizziness may mean lightheadedness, unsteadiness, anxiety, or a combination of the above. Nevertheless, the sudden onset and unsettling nature of their symptoms may lead the patient to their local emergency department or primary care provider’s office. The resolution of the presenting patient’s symptoms and overall experience greatly depends on the sophistication that the treating clinician has in dizziness and imbalance.
Health care providers are tasked with identifying the cause of the dizziness and recommending treatment; however, there is a dearth of professionals with expertise in dizziness compared to the number of patients seeking treatment for the disorder. Symptoms that patients qualify as dizziness are often non-specific and multifactorial (e.g., vestibular neuritis and migraines). Dizziness has a multitude of different etiologies each of which can generate similar symptoms. To correctly diagnose the origin of a patient’s dizziness, the appropriate laboratory tests and treatment strategies must be identified.
One of the fundamental factors in identifying the source(s) of a patient’s dizziness is collecting a thorough and accurate case history. During these evaluations, patients often describe their symptoms using non-specific descriptors. The lack of specific descriptions can lead to several etiologies that may present similarly. This typically leads to patients attending multiple physician appointments and undergoing testing which may be inappropriate and unnecessary to find a unifying diagnosis. The search for answers often results in no effective treatment plan, with up to 86% of patients receiving the diagnosis of “unspecified dizziness.” Additionally, the search for answers can be costly to both the patient and physician, especially given that a formal diagnosis or treatment may not be obtained. This leads to high financial costs for the patient and the health care system. The annual cost of dizziness evaluations now exceeds $4 billion a year to the United States emergency departments alone. One way to reduce the overall financial burden would be to create an effective and automatic triage system to reduce unnecessary physician visits and produce cost savings for patients and medical centers nationwide.
Initial Concept
More than 20 years ago, Neil T. Shepard, Ph.D., came to Mayo Clinic in Rochester, Minnesota, as the vestibular and balance program’s section head. Along with his physician colleagues, he created a coordinated multidisciplinary team approach to diagnosing and treating patients with chronic balance disorders. The coordinated team is outlined in Figure 1 and consists of clinicians from otolaryngology, neurology, psychiatry, physical therapy, and audiology.
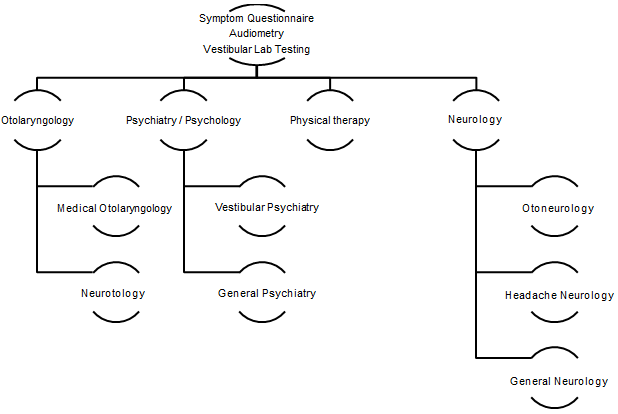
This coordinated team is referred to as the Mayo Integrated Neuro-vestibular Team or MINT. To be accepted into the program, patients with longstanding dizziness had to have a form of dizziness that was able to be treated by the subspecialists available in the MINT program. For example, patients with clearly defined autonomic dysfunction were referred to cardiology rather than the MINT. Subsequently, a formalized triaging system was needed first to determine if a patient was appropriate for the program. Secondly, once a patient was accepted, their condition’s most appropriate appointments needed to be selected based on the questionnaire’s responses. The original manual process is illustrated in Figure 2 and is the foundation for the current electronic automated algorithm-based triaging model being used in Mayo Clinic in Rochester, Minnesota.
As stated above, in order to be accepted, the patient first needed to complete a 162-item paper questionnaire. The questionnaire asked about the patient’s clinical history, in their own words, hearing and otologic history, medical history, including prior surgeries and headache history, along with what may exacerbate their symptoms. Once completed, the questionnaire was mailed back along with their external medical records and laboratory test results. Audiologists with expertise in dizziness were the initial point of contact once those records arrived. The audiology team would complete a thorough review of the questionnaire and external documentation. Following analysis, an optimal appointment set was created, and the patient was contacted and scheduled.
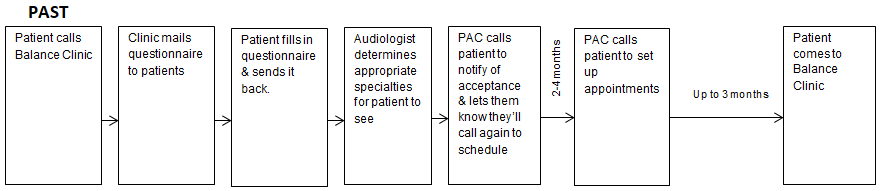
Our research has shown that this process was effective in reducing patient’s symptoms. However, given the manual nature and paper format, this process took an enormous amount of non-billable time for the audiology team to complete the pre-visit triage process. This generated the question: Could we automate the review process using machine learning techniques and have successful patient outcomes.
Development of Artificial Intelligence Triage Process
In 2016, Devin McCaslin, Ph.D., joined the team intending to automate the MINT documentation review process. Due to the MINT program’s success under Dr. Shepard’s leadership, patient volume was exceeding capacity, and there was a need more than ever to develop a solution to make the program more efficient. As the story goes, it was in a Martini Bar in Rochester, Minnesota, where Dr. McCaslin pitched the idea to Dr. Shepard about developing an algorithm model to manage the triage program. After two martinis each, the plan was hatched, and hence, the project’s unofficial title became the “Martini Project.”
As outlined in Figure 3, the formalized idea was to take the current manual review process and teach an algorithm to pick the most appropriate appointments for a patient’s source/s of dizziness automatically and consistently. One of the main goals was to reduce the time and effort expended by the audiology team and improve patient care and access.
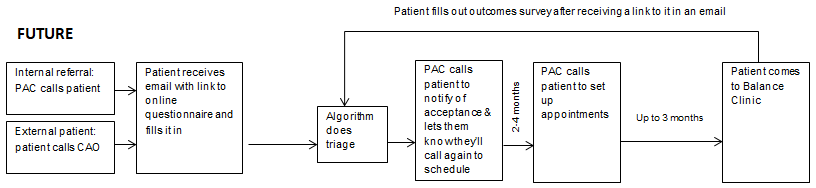
The first step was to train the algorithm with a data set culled from patients evaluated by the MINT. This was accomplished by having the multidisciplinary team members perform a retrospective analysis of the diagnosis and scheduled appointments to create an “ideal” set of consultations with the various specialties. The automated triage algorithm was then created from the responses obtained from the expert panel. This effort was essentially made to teach the algorithm how to pick the most appropriate provider to treat particular disorders. After the training process was completed and validated, the algorithm could be put into clinical use.
The automated triage algorithm process was completed using an existing application program interface (API) within Qualtrics. The triage model’s output is a list of recommended consultations presented to the triaging clinician for review and final approval. The list of appointments typically includes an audiometric evaluation, vestibular diagnostic testing (e.g., otolith and semicircular canal function), and vestibular and balance rehabilitation. The physician(s) that are best suited to address the patient’s symptoms are presented. Consultations will vary from patient-to-patient based on their symptom clusters but may include one or multiple specialists, including but not limited to otorhinolaryngology, neurology, and psychiatry. After approval, the file is designated as complete. Following the clinician cross-check of the model’s appointment selection, the patient’s information is made viewable by the patient appointment coordinator, who proceeds to contact the patient to schedule their appointments.
The development and utilization of a triage algorithm for our dizzy patients with longstanding symptoms at Mayo Clinic in Rochester, Minnesota, has been shown to address clinical optimization issues and improved patient outcomes. It has significantly reduced the amount of clinician time that was previously allocated to reading through paper charts. It has also improved patients’ ability to the most appropriate provider to evaluate and treat their dizziness concerns. For example, an initial finding was an approximately 20% drop in dizziness referrals to otolaryngology, which suggested we had an over-referral of patients to ENT. These patients could instead be seen by the provider that was most appropriate for their specific concerns.
It should be noted that our current model is not yet self-learning. That is, although outcome data is collected on patients, the model must be changed by a human. The team is “lightly supervising” the model’s output to ensure the most appropriate appointments are selected. If it is determined that the algorithm has recommended an inappropriate consultation as decided by the human reviewer, it is manually corrected, and the model registers this change. While the intention is to have the algorithm become self-learning (i.e., modify itself based on outcome data), significantly more data is needed.
Future Application(s)
The development of this model was specific to Mayo Clinic in Rochester, Minnesota. It was designed to maximize the use of the resources and providers available on the Rochester campus. However, it was recognized that thought should be given about how to modify this process to develop a system capable of helping clinicians worldwide treat and manage patients with dizziness. This meant the system needed to be adapted to work effectively in all environments such as private practices and government healthcare facilities, not just large tertiary clinics. To accomplish this goal, a partnership was formed with a Danish healthcare company called inno. health. This company has since developed a product called DizzyGuide to adapt this application to the various healthcare systems existing globally. Additionally, there is ongoing work to figure out how to incorporate vestibular laboratory testing into the model’s predictions. As well as analyze relationships between patient diagnoses with treatments and outcomes.
Lastly, it would be good to address a question when this topic comes up frequently: Will artificial intelligence replace audiologists completing vestibular and balance testing? The answer is no. This model’s development is to act as a decision support tool in terms of recommending appointments and treatment options. Audiologists completing vestibular testing still value their knowledge in the interpretation and recommendations based on the clinical history and results obtained from testing. This is another tool to help get the patient the fastest and most accurate diagnosis possible while lessening the time and money needed to get those answers for the patient and the healthcare system.
more recommended stories
Kaempferol: A Breakthrough in Allergy Management
Kaempferol, a dietary flavonoid found in.
Early Milk Cereal Drinks May Spur Infant Weight Gain
New research published in Acta Paediatrica.
TaVNS: A Breakthrough for Chronic Insomnia Treatment
A recent study conducted by the.
First-of-Its-Kind Gene-Edited Pig Kidney: Towana’s New Life
Surgeons at NYU Langone Health have.
Just-in-Time Training Improves Success & Patient Safety
A study published in The BMJ.
ChatGPT Excels in Medical Summaries, Lacks Field-Specific Relevance
In a recent study published in.
Study finds automated decision minimizes high-risk medicine combinations in ICU patients
A multicenter study coordinated by Amsterdam.
Study Discovers Connection Between Omicron Infection and Brain Structure Changes in Men
A recent study in the JAMA.
Advancing COPD Prognosis: Deep Learning Models
Researchers conducted a meta-analysis in a.
4 in 1 Test for Swine Flu, COVID-19, RSV, and Influenza
In the United Kingdom, the first.
Leave a Comment