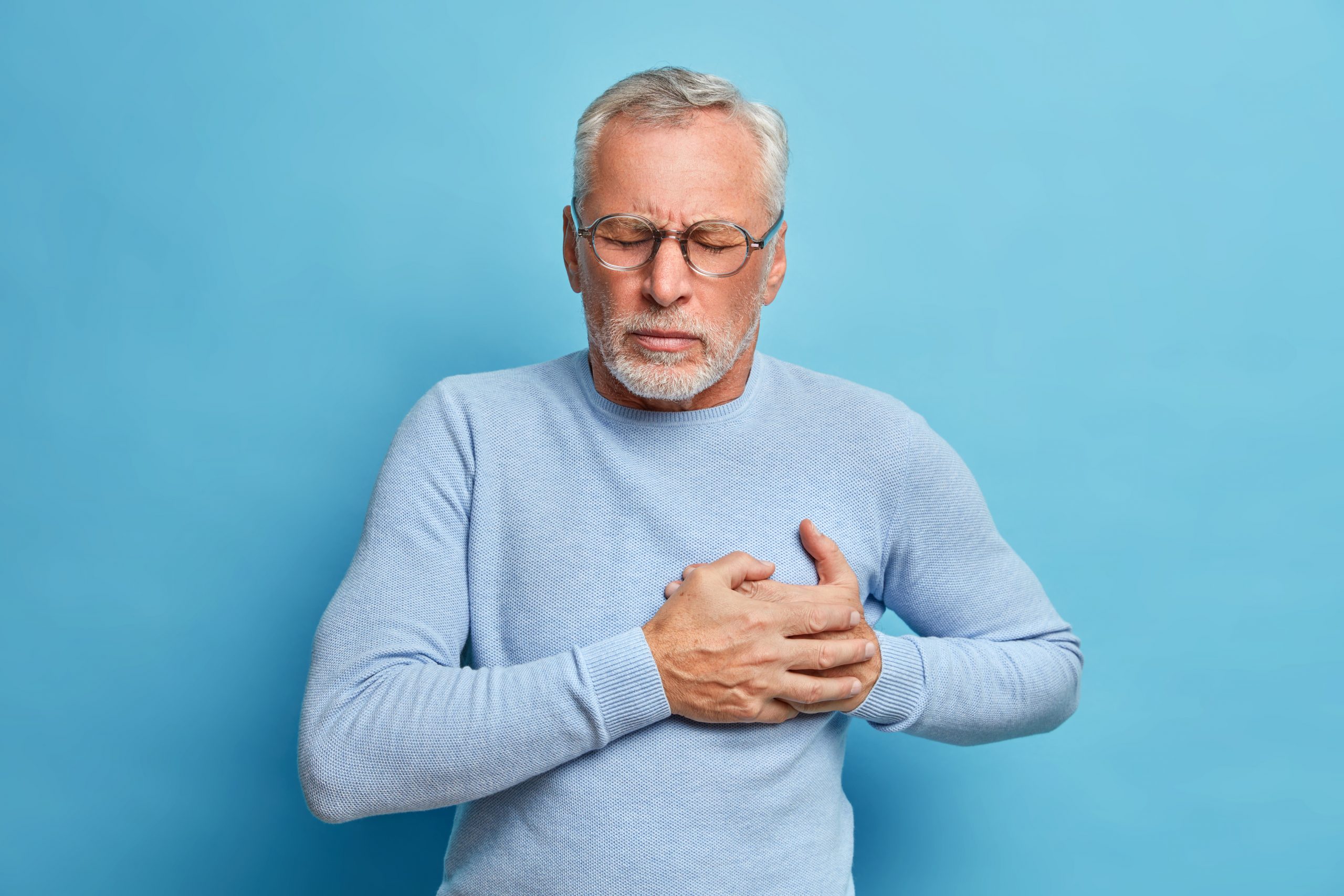
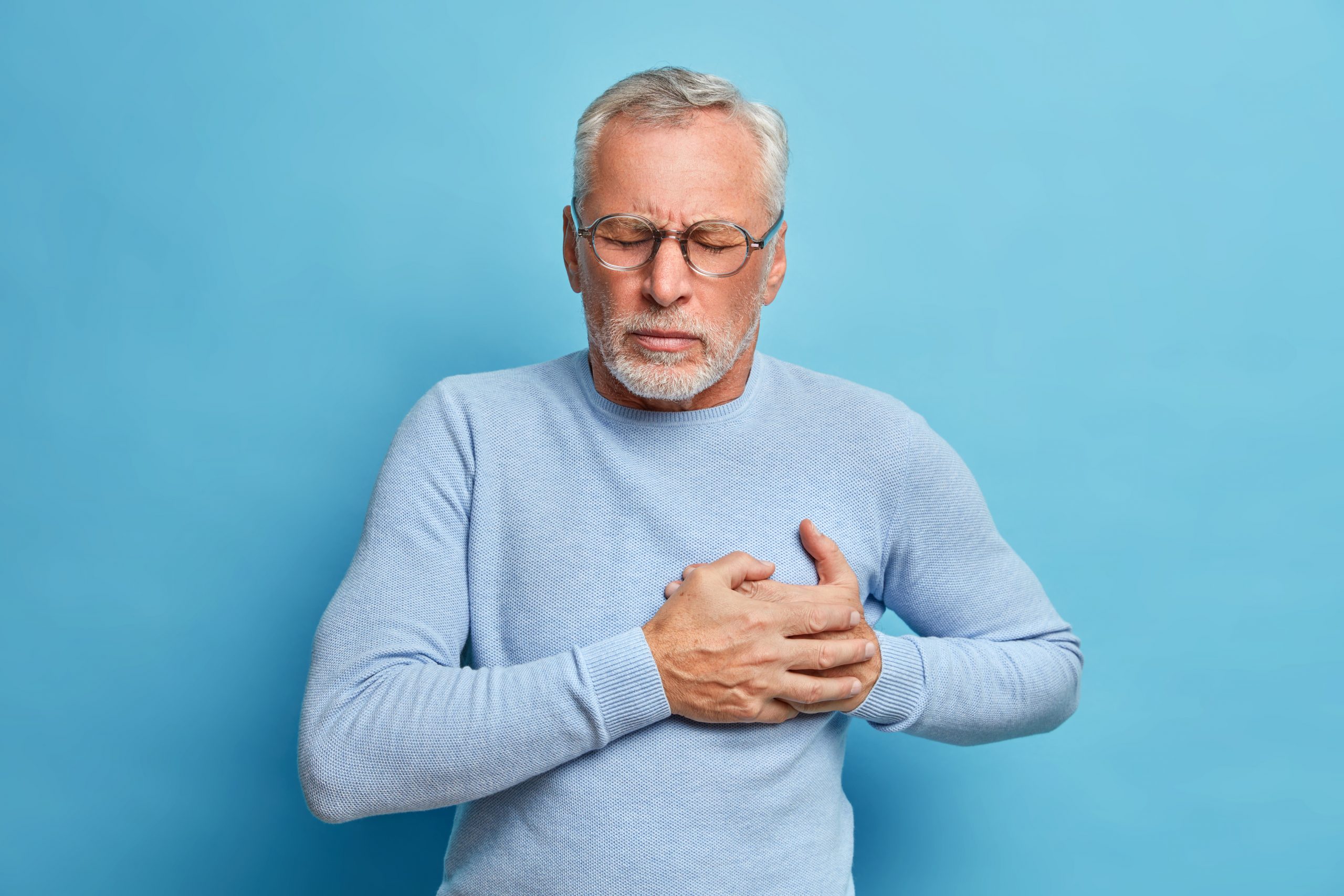
A deep learning artificial intelligence model has been created by researchers from Brigham and Women’s Hospital and Keio University in Japan to check the electrocardiogram (ECG) for indications of atrial septal abnormalities (ASD). Due to the lack of symptoms prior to the development of irreversible consequences, this condition—which can induce heart failure—is underreported. Their findings have been made public in eClinicalMedicine.
“If we can deploy our model on a population-level ECG screening, we would be able to pick up many more of these patients before they have irreversible damage,” said Shinichi Goto, MD, Ph.D., corresponding author on the paper and instructor in the Division of Cardiovascular Medicine at Brigham and Women’s Hospital.
Adult congenital heart disease, or ASD, is rather frequent. A hole in the heart’s septum that allows blood to pass between the left and right atriums is the root cause of this condition. According to Goto, it is diagnosed in 0.1% to 0.2% of the population but is probably underreported. ASD symptoms are frequently nonexistent or just mildly present until later in life. Symptoms include the inability to engage in rigorous activity, changes to the heartbeat’s rhythm or pace, palpitations, and an elevated risk of pneumonia.
ASD can stress the heart and raise the risk of atrial fibrillation, stroke, heart failure, and pulmonary hypertension even when no symptoms are present. Even if the flaw is eventually repaired, the consequences of ASD are already permanent at that point. ASD can be treated with minimally invasive surgery to improve life expectancy and lower complications if discovered early.
ASD can be discovered in a number of ways. The first step is to use a stethoscope to listen to the heart to identify the greatest abnormalities. But this method can only be used to find roughly 30% of patients. Another is an echocardiography, which is not a suitable option for screening because it requires a lot of time and effort. Electrocardiography, or ECG, is another test that can be used as a screening tool because it just takes a minute or so to complete. There is however poor sensitivity for detecting ASD when humans examine an ECG readout for known abnormalities linked with the disorder.
The study team input ECG data from 80,947 patients over 18 who had both ECG and echocardiography to determine ASD to a deep learning model to test if an AI model could more accurately detect ASD from ECG readouts. 857 people in all received an ASD diagnosis. The information was gathered from three hospitals: Saitama Medical Center in Japan, Dokkyo Medical University, and two sizable teaching hospitals: BWH in the US and Keio University in Japan.
Then, utilizing scans from Dokkyo, which has a more diverse population and isn’t specifically screening individuals for ASD, the model was put to the test. The algorithm was more accurate at detecting ASDs than screening for known ECG abnormalities. While known abnormalities indicated ASD 80.6% of the time, the model properly detected ASD 93.7% of the time.
“It picked up much more than what an expert does using known abnormalities to identify cases of ASD,” Goto said. One limitation of the study is that the model was trained used samples from academic institutions, which deal more with rare diseases like ASD. All the patients used to train the model were being screened for ASD and received an echocardiogram, so it is not clear how well the model would work on a general population, which is why they tested it in Dokkyo. “The model’s performance was retained even in the community hospital’s general population, which suggests that the model generalizes well.”
The authors also point out that not all defects will be found even when using an echocardiography to determine ASD. Even though these minor flaws are less likely to need surgical repair, some may evade both routine screening and the AI model. “The problem of machine learning is that it’s a black box—we don’t really know what features it picked up,” Goto said. That implies that the model also cannot teach us what characteristics to search for in ECGs.
The method may be utilized in population-level screening to identify ASD before it causes irreversible heart damage, according to the results. ECG is being used in numerous applications and is quite inexpensive. “Perhaps this screening could be integrated into an annual PCP appointment or used to screen ECGs taken for other reasons,” Goto said.
more recommended stories
Caffeine and SIDS: A New Prevention Theory
For the first time in decades,.
Microbial Metabolites Reveal Health Insights
The human body is not just.
Reelin and Cocaine Addiction: A Breakthrough Study
A groundbreaking study from the University.
Preeclampsia and Stroke Risk: Long-Term Effects
Preeclampsia (PE) – a hypertensive disorder.
Statins and Depression: No Added Benefit
What Are Statins Used For? Statins.
Azithromycin Resistance Rises After Mass Treatment
Mass drug administration (MDA) of azithromycin.
Generative AI in Health Campaigns: A Game-Changer
Mass media campaigns have long been.
Molecular Stress in Aging Neurons Explained
As the population ages, scientists are.
Higher BMI and Hypothyroidism Risk Study
A major longitudinal study from Canada.
Therapeutic Plasma Exchange Reduces Biological Age
Therapeutic plasma exchange (TPE), especially when.
Leave a Comment