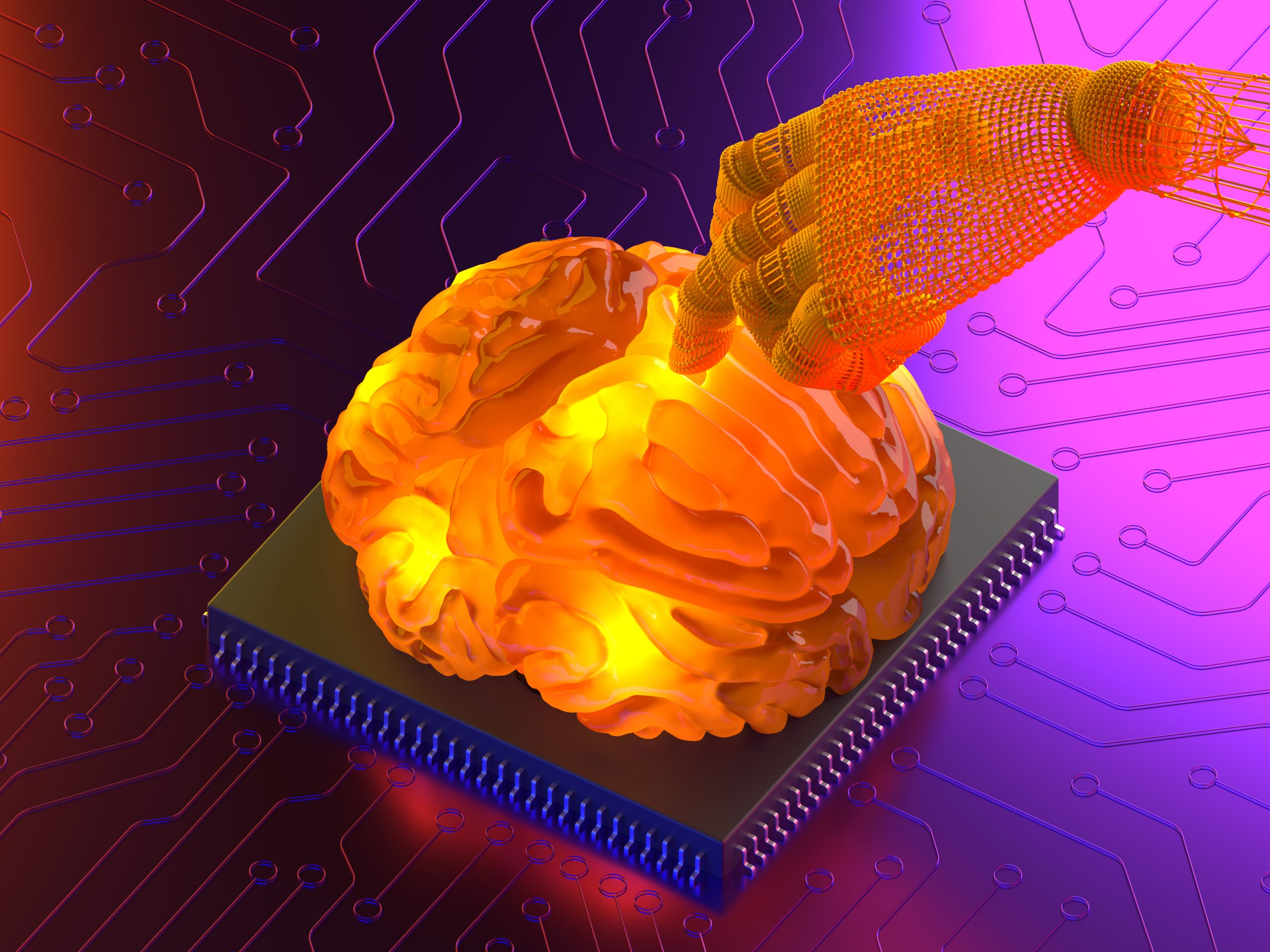
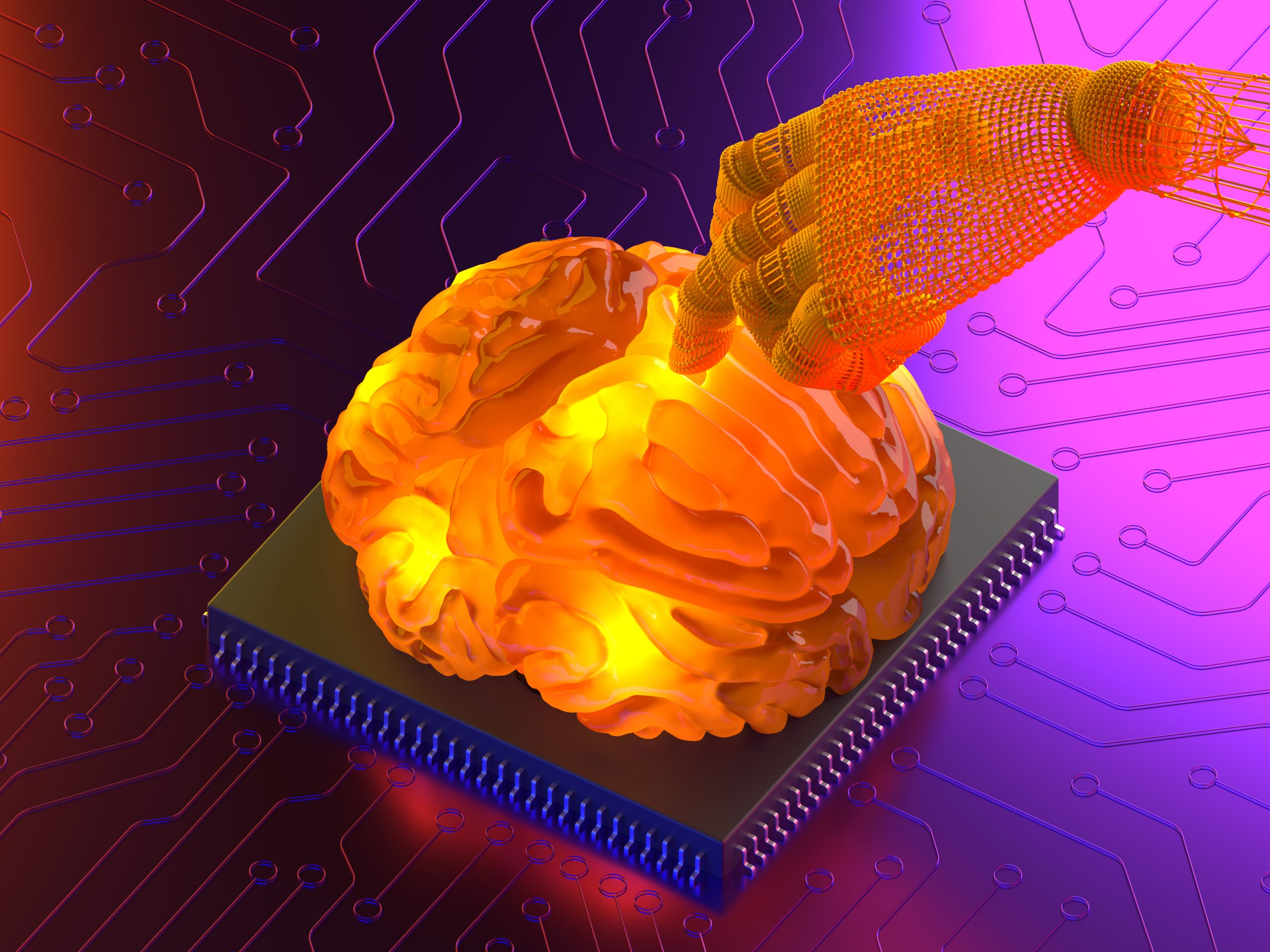
An algorithm developed by researchers at Stanford Medicine may aid doctors in better understanding and treating challenging tumors like Glioblastoma Brain Cancer.
Glioblastoma, a rapid and aggressive form of brain cancer, typically leads to an average survival duration of approximately one year following diagnosis. Its complex treatment is further complicated by the significant variations in the cellular composition of each tumor across individuals.
“Because of the heterogeneity of this disease, scientists haven’t found good ways of tackling it,” said Olivier Gevaert, PhD, associate professor of biomedical informatics and of data science.
Because it can be challenging to determine which malignant cells are responsible for each patient’s glioblastoma, medical professionals and researchers also struggle with prognosis.
However, researchers from Stanford Medicine and their associates have recently created an artificial intelligence model that analyzes stained images of glioblastoma tissue in order to forecast a patient’s tumor’s aggressiveness, identify the genetic make-up of the tumor cells, and assess whether significant cancerous cells are still present after surgery.
In a new study, their team described how the model could aid physicians in identifying individuals who had cellular markers indicative of more aggressive tumors and flagging them for expedited follow-up.
Novel Perspectives on Glioblastoma
Some cancer cells virtually invariably persist even after glioblastoma patients get surgery, radiotherapy, and chemotherapy. Most GBM patients relapse, however, some do so more quickly than others.
Histology photos, or photographs of colored disease tissue, are frequently used by medical professionals and researchers to assist them in detecting tumor cells and developing treatment strategies. Although the photos often demonstrate the size and position of cancer cells, they usually do not fully depict the tumor. A far more sophisticated method known as spatial transcriptomics evolved in recent years.
It uses certain chemicals to identify the genetic material in tumor cells and exposes the location and genetic makeup of numerous cell types.
“The spatial transcriptomics data allows us to look at these types of tumors in a way that was not possible previously,” Gevaert said. “But it’s currently an expensive technology. It takes a few thousand dollars to generate data for a single patient.”
Gevaert and Zheng used artificial intelligence (AI) to streamline the procedure. They created a model to use spatial transcriptomics and enhance simple histology pictures to produce a more accurate tumor map.
“The model showed which cells like to be together, which cells don’t want to communicate and how this correlates with patient outcomes,” Gevaert said.
Constructing the Model
The researchers built the model using genetic information from more than 20 individuals with glioblastoma brain cancer and spatial transcriptomics images. The model discovered that specific cell types, cell-cell interactions, and profiles were associated with better (or worse) cancer outcomes based on these in-depth images.
For instance, the model discovered that patients appeared to have more rapid, aggressive malignancies when tumor cells known as astrocytes, which resemble neuron support cells, packed together inappropriately. According to other research, astrocyte clusters transmit biological signals that promote tumor growth.
The model may contribute to the development of more effective glioma treatments by exposing cellular patterns like this obvious clumping, according to Gevaert.
The model was further refined to distinguish various tumor cells in related histology images with an accuracy of 78% or higher using spatial transcriptomics data from the same glioblastoma patients. Essentially, it made use of the shape of the cells to foretell which genes are “on” and “off,” which is information that discloses a cell’s identity.
In addition, Zheng expects that clinicians will be able to determine what proportion of a tumor was effectively removed after surgery and how much is still present inside the brain using this technique. Their simulations revealed that the center of a patient’s tumor frequently contains tumor cells with genetic signs of oxygen deprivation. Worse cancer outcomes were associated with higher proportions of these cells.
After training the model to detect the spatial distribution of distinct cell types using simple images, scientists assessed its effectiveness using a more extensive, distinct dataset of histology images obtained from 410 patients. Through these images, the model started deducing potential cancer outcomes. The team observed the model’s capability to recognize cellular arrangements that correlated with the aggressiveness of cancer.
The underlying concept suggests that in the future, this model could aid doctors in pinpointing patients exhibiting cellular patterns indicative of a more aggressive tumor. This early identification could signal an impending risk, whether from relapse or swift tumor expansion.
What’s on the Horizon?
Zheng expresses enthusiasm for the predictive potential of the model, but underscores the necessity of training it on a more extensive patient cohort prior to its deployment in clinical practice. His intention is to enhance the model’s capabilities, enabling it to generate even more detailed cellular mappings of glioblastoma tumors.
Presently, an initial version of their model, GBM360, serves as a proof-of-concept and is accessible for researchers to upload diagnostic images, thus forecasting outcomes for glioblastoma patients. However, Zheng emphasizes that the model remains in the research phase, cautioning against its direct application in patient care for now.
Looking forward, Zheng envisions the algorithm as a tool capable of foreseeing results for various conditions, such as breast or lung cancers.
“I think these multimodal data integrations can shape improvement of personalized medicine in the future.”
Researchers affiliated with the University of Granada, Ghent University, and the University of Freiburg contributed to the work.
The study was funded by the National Cancer Institute (grant R01CA260271).
more recommended stories
Early Brain Development: Gene Control Atlas Unveiled
An atlas of the brain’s early.
PLS Riskier in ABO Mismatched Lung Transplants
Passenger lymphocyte syndrome (PLS) is a.
Lysosomal Function in Parkinson’s Disease Risk
The genetic traits that affect the.
Omega-6 Fatty Acid Role in Bipolar Disorder
Higher concentrations of arachidonic acid, an.
Transplant Drug Shows Promise in Slowing Alzheimer’s in Seizure Patients
Protein imbalances, which heighten the excitability.
Fentanyl Inhalation: Brain Damage Risks
After treating a middle-aged man who.
Enhanced Immune Response in New COVID-19 Vaccines
An improved vaccination offers an enhanced.
Bioresorbable Wireless Sensor for Early Stomach Leakage Detection
In a recent publication in Scientific.
Neurocardiac Connectivity in Depression Treatment
Heart rate deceleration and sadness may.
Antioxidants: Impact on Quality of Life in Acne Vulgaris
A recent study published in the.
Leave a Comment