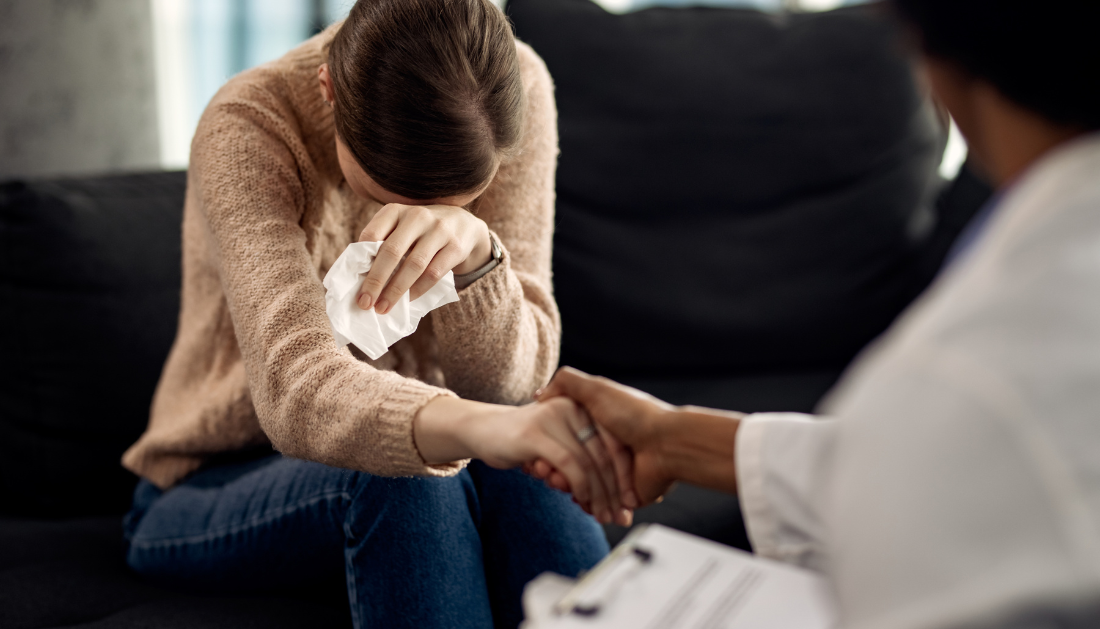
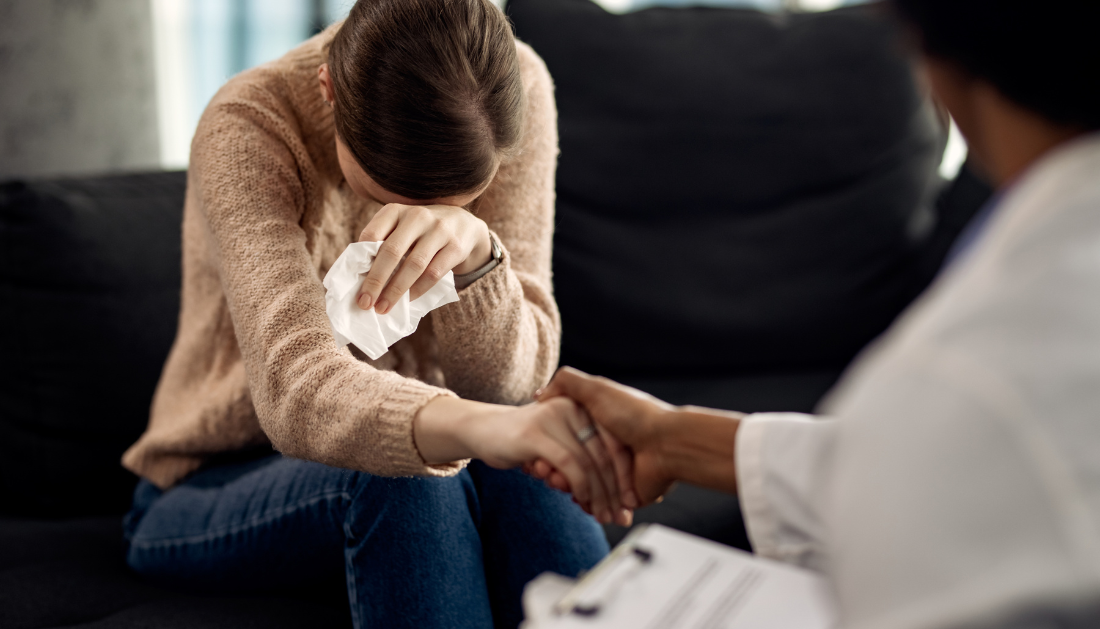
Researchers utilized machine learning alongside brain structural measurements, anxiety, and sleep quality assessments to predict depressive symptom severity (DSS), which refers to the degree or intensity of depressive symptoms an individual is experiencing. The predictive model’s results were tested for generalizability across three independent datasets. This groundbreaking study was recently published in the eBioMedicine journal, showcasing the potential of machine learning in advancing mental health research.
Context
In contemporary countries, depression symptoms affect approximately 25% of the population. These symptoms have become more prevalent over time and can develop into major depressive disorder (MDD) within 15 years. Reducing depression rates requires early detection.
Depression and sleep disorders, especially insomnia, are associated, although the relationship is complicated because of individual variations in stress, anxiety, and heredity.
More study is required to improve the accuracy and generalizability of predictive models across a range of populations, as well as to better understand the relationships between sleep, anxiety, brain anatomy, and depressive symptoms.
About the Study
The Washington University-University of Minnesota (WU-Minn HCP) consortium obtained the Human Connectome Project (HCP)-Young dataset, which consists of young adults in good health between the ages of 22 and 35. Individuals with ongoing medical, mental, or neurological disorders, heart problems, substance misuse, or treatment plans are not included.
Of the 1,206 subjects, 1,101 had full structural magnetic resonance imaging (MRI), sleep quality, anxiety, and depression symptoms data. Those with a history of clinical depression were not included in a subsequent analysis. Although persons from 36 to over 100 were recruited for the HCP-Aging dataset, only those between the ages of 36 and 59 were included because of the DSS questionnaire’s design.
Last but not least, individuals between the ages of 18 and 59 were able to access cross-sectional and longitudinal data from the Enhanced Nathan Kline Institute (eNKI) dataset, a community-representative sample. This made it possible for researchers to evaluate how generalizable machine learning models were and to forecast DSS in the future using baseline measurements of anxiety and sleep.
Each cohort’s ethical clearances are accessible online, and the University Hospital of Heinrich-Heine University Düsseldorf has given permission for analysis.
Advanced neuroimaging and machine learning approaches were used to process the data, with an emphasis on neurobehavioral predictions and gray matter volume. The models were validated by testing predictions on several datasets.
Through mediation studies, the links between anxiety, DSS, and sleep quality were further investigated. This helped to elucidate the ways in which behavioral and neural components contribute to depressive symptoms in various groups.
In conclusion
In summary, the results showed that DSS could be accurately predicted by sleep quality across three different datasets. The model’s predicted accuracy was significantly improved by include anxiety. Measurements of the structural and functional brain, however, did not moderate the link between sleep quality and DSS, nor did they significantly predict DSS.
Similar outcomes were produced by the ML models on several independent datasets, highlighting the approach’s generalizability.
Based on baseline anxiety and sleep quality, the models also reliably predicted future DSS in a longitudinal subsample. The validity of these results was validated by complementary analyses that took into account factors like anxiety’s mediating function, sleep-related confounders, and depression history.
For more information: Prediction of depressive symptoms severity based on sleep quality, anxiety, and gray matter volume: a generalizable machine learning approach across three datasets, eBioMedicine, https://doi.org/10.1016/j.ebiom.2024.105313
more recommended stories
Immunotherapy Boosts Survival for High-Risk Bladder Cancer Patients
According to the findings of a.
New Study Extends Ovarian Health Span
The ovaries of a woman function.
Coffee and Tea May Lower Dementia Risk in Hypertensives
Researchers used data from 453,913 participants.
Unique Gut Bacteria Found in Singapore Seniors
Researchers used deep shotgun metagenomic sequences.
Disrupted Glucose Transport Linked to Myelin Thinning and Aging
Researchers looked at how oligodendroglial lipid.
Study Links Nighttime Light Exposure to Increased Alzheimer’s Risk
Researchers looked at the connection between.
Cruciferous Vegetables Lower Blood Pressure in Adults
Researchers conducted a crossover-design randomized controlled.
Machine Learning: Enhancing Flu Vaccine Development
A machine learning algorithm that can.
Immunotherapy Reduces Death & Recurrence in Lung Cancer
According to a new analysis by.
Hormones and Gender Differences in Immune Response
Scientists have discovered that hormones have.
Leave a Comment