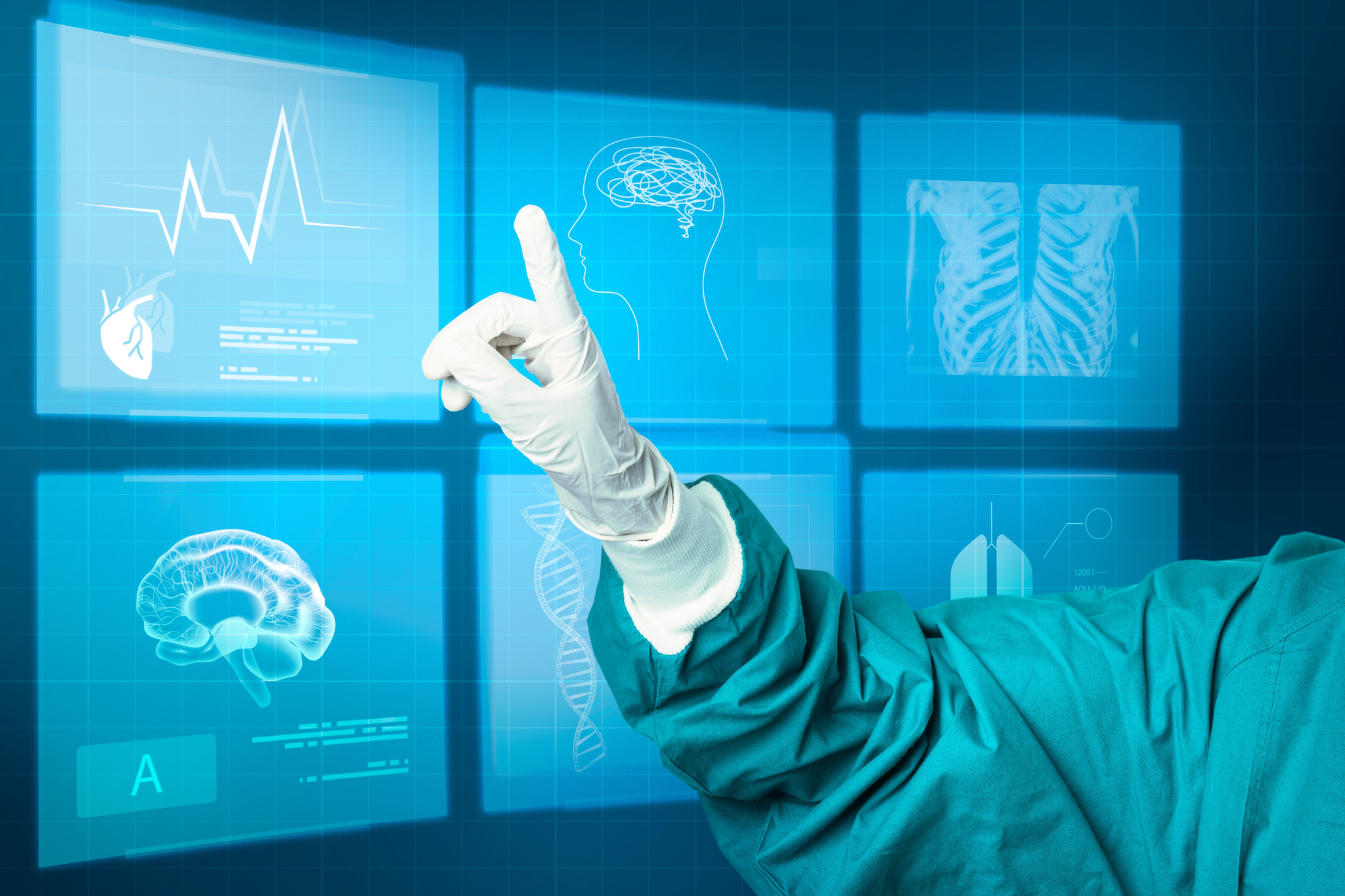
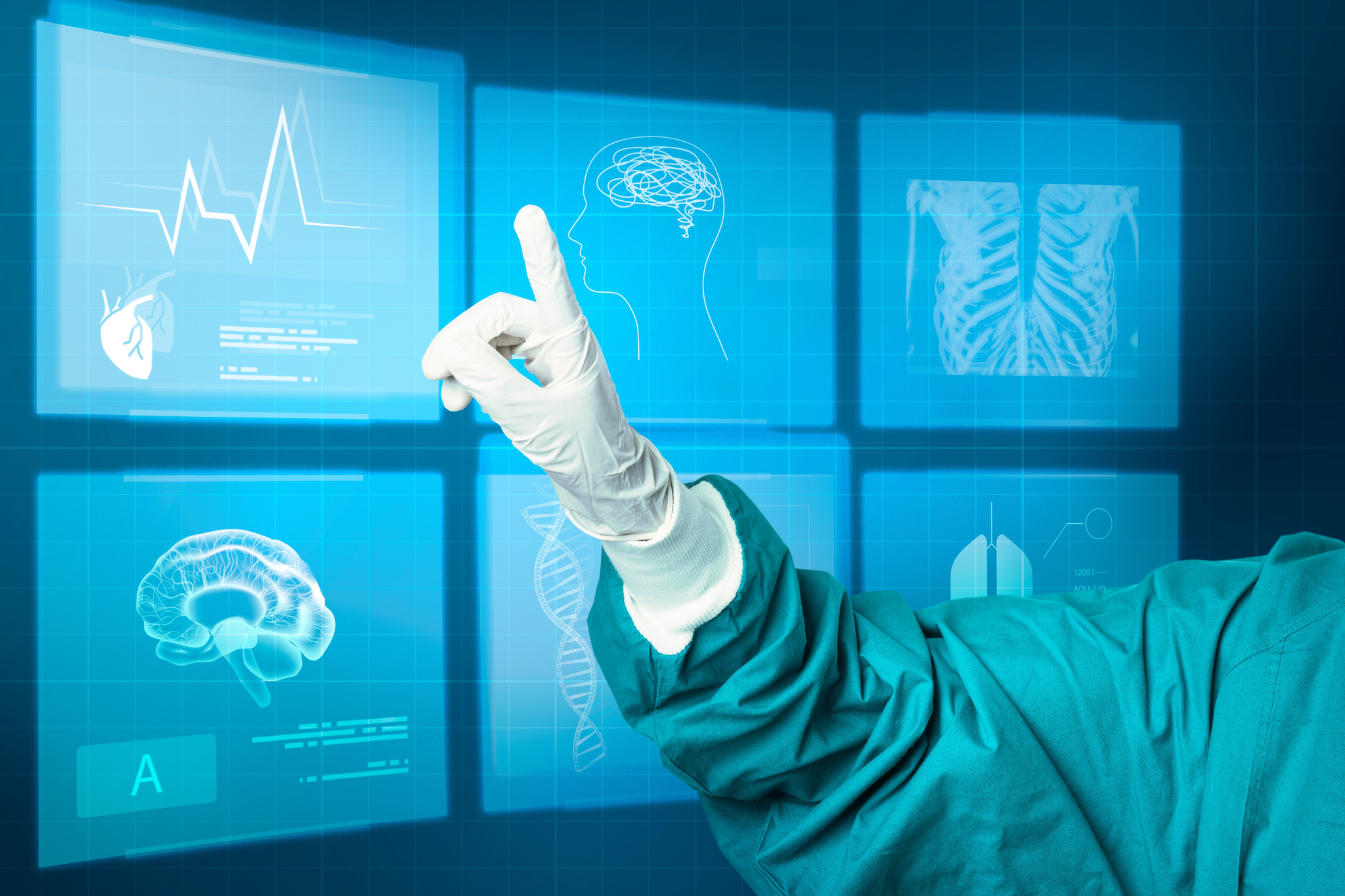
In a recent study, CHARTwatch—a machine learning-based model that forecasts patient decline—was clinically assessed by academics and published in the Canadian Medical Association Journal (CMAJ).
Context
Increasing patient safety depends on recognizing, anticipating, and responding to clinical deterioration in hospitalized patients. The main reason for needless admissions to the intensive care unit (ICU), which leads to longer stays and a higher death rate, is unidentified clinical deterioration. Prediction tools are widely used, although the data supporting their value is not always clear-cut.
An automated risk prediction model combined with remote nurse monitoring and on-the-ground interventions by quick reaction teams reduced 30-day mortality by 16%, according to a Kaiser Permanente study of 19 hospitals in Northern California. Advanced early warning systems may improve clinical results, although it is unclear what technological and clinical features they have.
About the Study
The current study looked on whether CHARTwatch could enhance clinical outcomes related to patient deterioration.
Utilizing real-time information from electronic medical records, the algorithm forecasts patient decline. Time-series summaries, variations in risk ratings from prior evaluations, and risk score projections from earlier interactions were all taken into account by the time-aware multivariate adaptive regression spline (MARS) technique.
The model contained a clinical route for the high-risk patient category, including increased vital sign monitoring, notifications for palliative care consultations, and physician evaluation within an hour. It was shared with nurses and doctors via texts and email.
The intervention was administered to patients admitted to the general internal medicine (GIM) unit of St. Michael’s Hospital between November 1, 2020, and June 1, 2022. The time frame prior to the intervention was from November 1, 2016, until June 1, 2020.
Intervention beneficiaries were compared to patients admitted prior to the intervention using weighting based on propensity scores. The general internal medicine unit’s intervention recipients and non-recipients from the respiratory, nephrology, and cardiology units were compared using difference-indifferences assessment.
Within-hospital mortality from non-palliative care, or deaths that did not stem from a documented palliative care therapy, was the main outcome measure.
The following were secondary endpoints: length of hospital stay, ICU transfer, a composite measure of transfer to ICUs or mortality, palliative deaths, total deaths, and transfers (a composite measure of deaths among palliative care recipients or shifts to inpatient palliative care units).
Patient diagnosis was determined using the tenth revision of the International Classification of Diseases, Canadian version (ICD-10-CA). For patients in the control group, researchers computed model predictions in retrospect.
Only throughout the GIM unit patients’ interventional phase did clinicians receive alerts. Those with influenza or COVID-19, as well as those with preadmission palliative care comorbidities, were not included in the study. Propensity scores for the GIM and subspecialty cohorts were computed using logistic regressions.
The relative risk (RR) was computed by the researchers after accounting for study variables. Linear models compared continuous outcomes, while Poisson regressions compared binary outcomes.
Age, gender, comorbidities, hospitalizations within the previous six months, hospitalization month, vital signs, homelessness, racial and new demographic groups in the area, material resources available to the neighborhood, and admission to the ICU prior to transfer to subspecialty wards or GIM units were among the study covariates.
In summary
According to the study, using CHARTwatch for GIM admissions was associated with a lower risk of non-palliative care-related death as compared to the preintervention era.
The findings indicate that machine learning-based early warning systems are promising tools for enhancing healthcare outcomes.
However, because of the possibility of unmeasured confounding, one should interpret results with caution. Subsequent research endeavors will evaluate the intervention’s equity-related components as well as the clinical team members’ qualitative viewpoints.
For more information: Clinical evaluation of a machine learning-based early warning system for patient deterioration, CMAJ, DOI: https://doi.org/10.1503/cmaj.240132
more recommended stories
Two Surgeries Equally Effective for Blinding Eyelid Condition
The results of a big comparison.
Immunotherapy Boosts Survival for High-Risk Bladder Cancer Patients
According to the findings of a.
New Study Extends Ovarian Health Span
The ovaries of a woman function.
Coffee and Tea May Lower Dementia Risk in Hypertensives
Researchers used data from 453,913 participants.
Machine Learning Links Sleep, Anxiety to Depression Risk
Researchers utilized machine learning alongside brain.
Unique Gut Bacteria Found in Singapore Seniors
Researchers used deep shotgun metagenomic sequences.
Disrupted Glucose Transport Linked to Myelin Thinning and Aging
Researchers looked at how oligodendroglial lipid.
Study Links Nighttime Light Exposure to Increased Alzheimer’s Risk
Researchers looked at the connection between.
Cruciferous Vegetables Lower Blood Pressure in Adults
Researchers conducted a crossover-design randomized controlled.
Machine Learning: Enhancing Flu Vaccine Development
A machine learning algorithm that can.
Leave a Comment