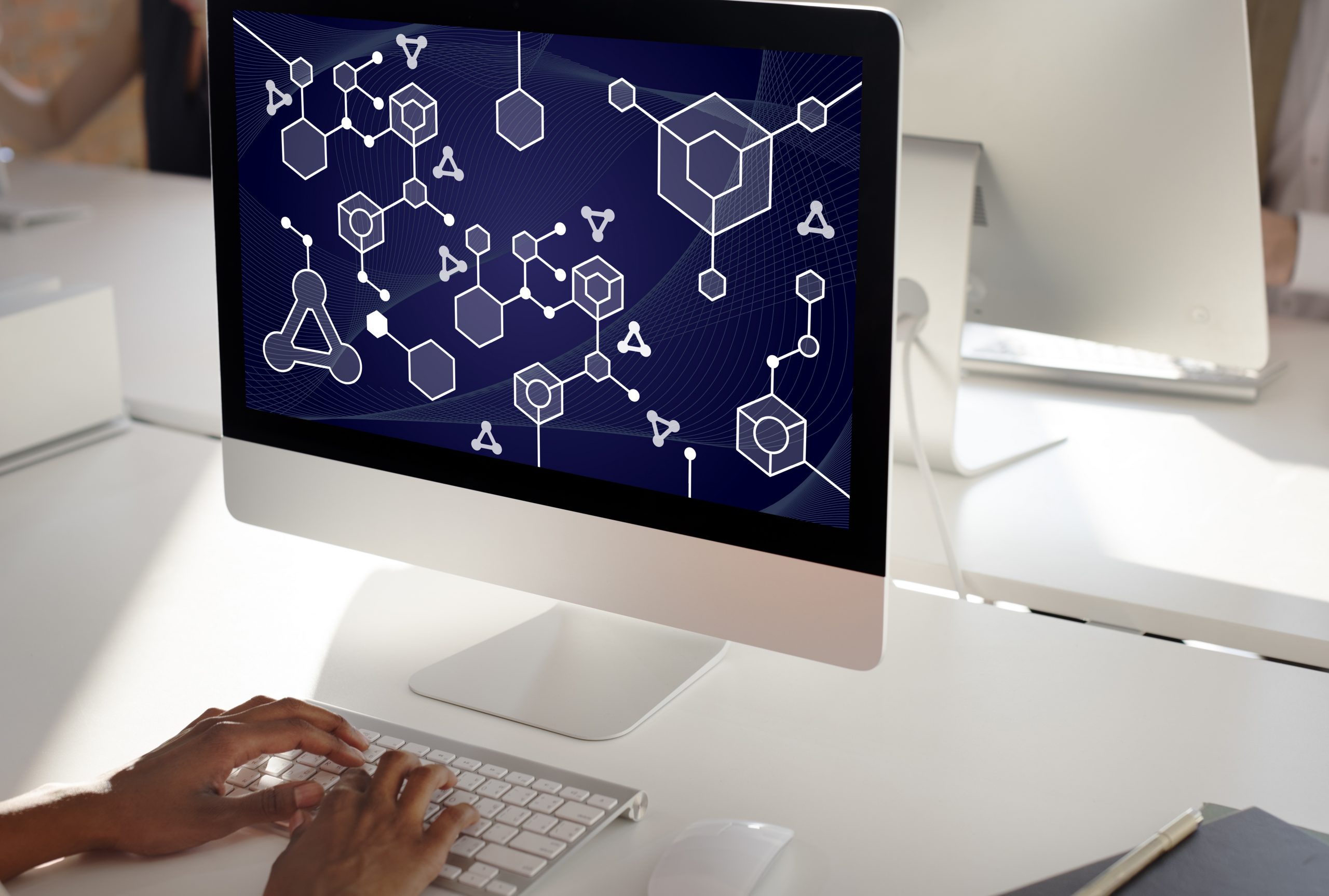
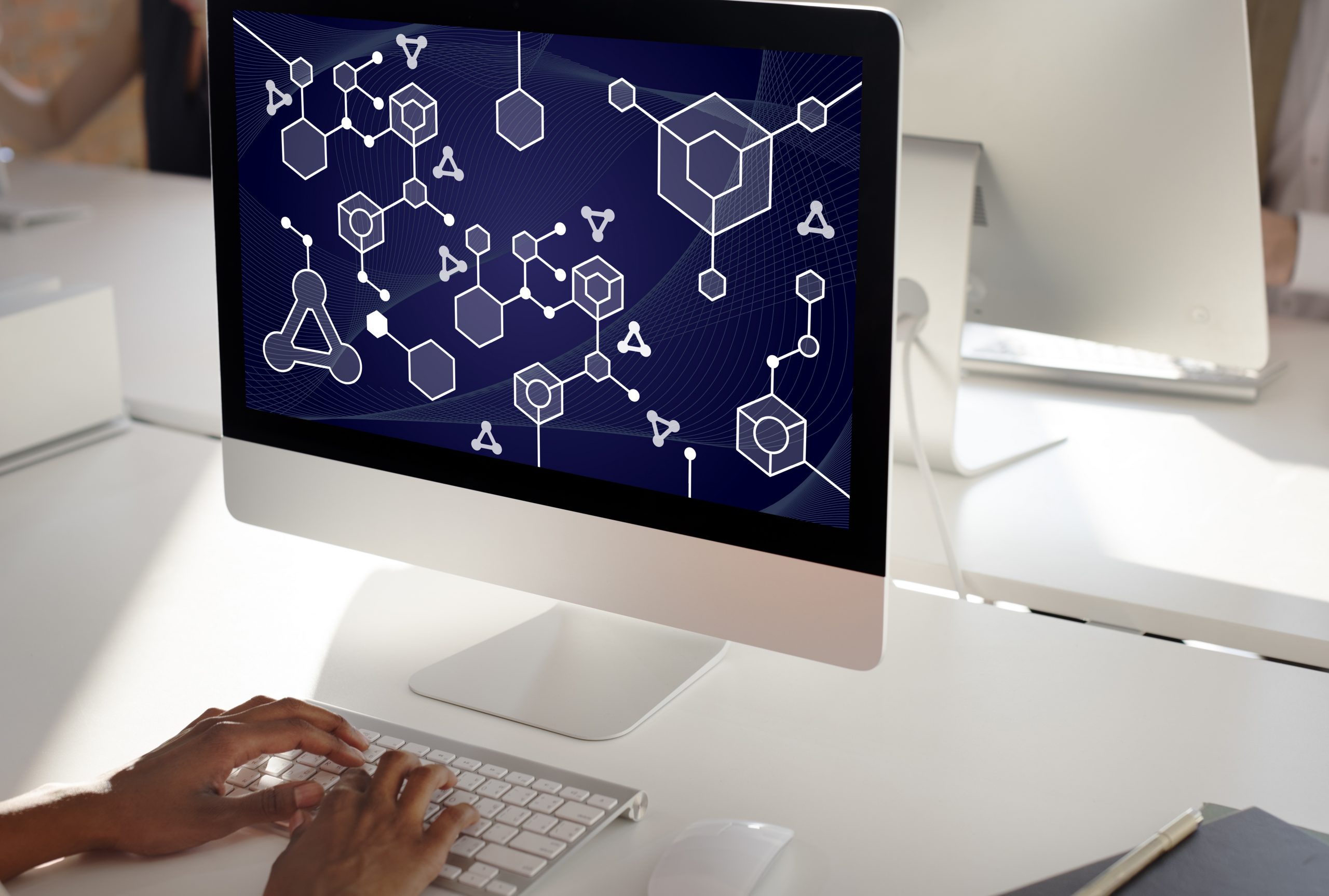
Because race is a social construct, and the molecular mechanisms by which race influences clinical outcomes are frequently unknown, health practitioners are increasingly worried that adding race in predictive clinical algorithms for decision-making may exacerbate injustices.
For example, to determine the estimated glomerular filtration rate, or eGFR, health care clinicians utilize an algorithm based on age, biological sex, race (Black or non-Black), and serum creatinine, a waste product released by the kidneys into the blood. Higher eGFR values indicate improved renal health. In the United States, these eGFR estimations are used to arrange kidney transplants.
A Black patient would be assigned a greater eGFR than a non-Black patient of the same age, gender, and serum creatinine level based on this algorithm, which was trained using actual GFR values from patients. This suggests that some Black individuals are thought to have healthier kidneys than otherwise comparable non-Black patients and are therefore less likely to be given a kidney transplant.
Researchers discovered in 2021, however, that omitting race from the initial eGFR formulae could result in bigger differences between estimated and actual GFR values for both Black and non-Black individuals. They also discovered that adding another biomarker called cystatin C can improve predictions. Even with this biomarker, omitting race from the algorithm resulted in higher disparities between races.
Different perspectives on fairness
To explain how society allocates resources, researchers employ many economic models. Utilitarianism and equality of opportunity are two important frameworks.
A purely utilitarian viewpoint aims to determine what characteristics would maximize the benefits of a favorable outcome or minimize the harm caused by a negative one, regardless of who possesses such characteristics. This strategy directs resources to individuals who have the greatest potential to achieve positive results or mitigate unfavorable ones.
Regardless of whether it is fair, a utilitarian approach would always incorporate race and ethnicity to improve the predictive power and accuracy of algorithms. Utilitarian policies, for example, would seek to promote overall survival among those requesting organ transplants. They would give organs to people who would live the longest after transplantation, even if those who would die sooner without the donation due to factors beyond their control would die sooner.
Opportunity equality
Equality of opportunity is founded on two essential ideas.
To begin, disparity in outcomes is immoral if it results from differences in conditions beyond an individual’s control, such as a child’s parents’ poverty, exposure to systemic racism, or living in violent and unsafe environments. This can be solved by rewarding persons with disadvantaged circumstances in such a way that they have the same chance of achieving particular health outcomes as those who are not disadvantaged by their circumstances.
Second, disparities in results for people in similar situations due to differences in individual effort, such as practicing health-promoting activities like diet and exercise, are not unethical, and policymakers can encourage those who achieve better outcomes through such practices. Individual effort inequalities caused by circumstances, such as living in a region with restricted availability to healthful food, are not addressed under equality of opportunity. Keeping all other factors constant, any disparities in effort between persons should be due to personal preferences, free will, and perceived advantages and costs. This is known as accountable effort. As a result, two people in the same situation should be rewarded based on their accountable efforts, and society should tolerate the consequent disparities in outcomes.
If algorithms are to be employed for clinical decision-making, it is vital to understand what causes variation in the predictions they produce.
If variation in predictions is caused by differences in circumstances or biological conditions rather than by individual accountable effort, then the algorithm should be used for compensation, such as allocating kidneys so that everyone has an equal chance of living the same length of life, rather than reward, such as allocating kidneys to those who would live the longest with the kidneys.
In contrast, if variation in forecasts is caused by changes in individual accountable effort but not by their circumstances, then the algorithm should be used for reward rather than compensation.
Evaluating the fairness of clinical algorithms
To hold machine learning and other artificial intelligence algorithms responsible to a standard of equity, I evaluated whether race should be included in clinical algorithms using equality of opportunity principles. I ran simulations under both ideal data settings, in which all information about a person’s circumstances is available, and real data situations, in which some information about a person’s circumstances is lacking.
Better biomarkers may one day be able to predict health outcomes more accurately than race and ethnicity. Until then, incorporating race into specific therapeutic algorithms could aid in the reduction of inequities.
more recommended stories
Screen Addiction in Teens May Fuel Manic Episodes
A new study published in Social.
New Frailty Intervention Reduces ER Visits by 35%
A new study led by researchers.
ADHD and Gut Health: The Role of Chili Peppers
The Gut Health-Brain Axis and ADHD:.
HEALEY Platform Accelerates ALS Therapy Research
A New Era of ALS Clinical.
Can Your Genetics Influence Your Income and Health?
A New Perspective on Health and.
Tracking Immune Cells in Blood Predicts Cancer Survival
A new study from University College.
Dream Recall: The Role of Personality, Sleep, and Cognitive Traits
A recent study from the IMT.
Higher BMI Linked to Stronger Memory in Midlife Adults
Does Obesity Boost Brainpower? Study Links.
Antibiotic-Resistant Bacteria Found in Hospital Drains
Dangerous Bacteria Found in Hospital Sink.
Pancreatic Cancer Immune Map May Guide Future Therapies
New Immune Map Reveals Key Insights.
Leave a Comment