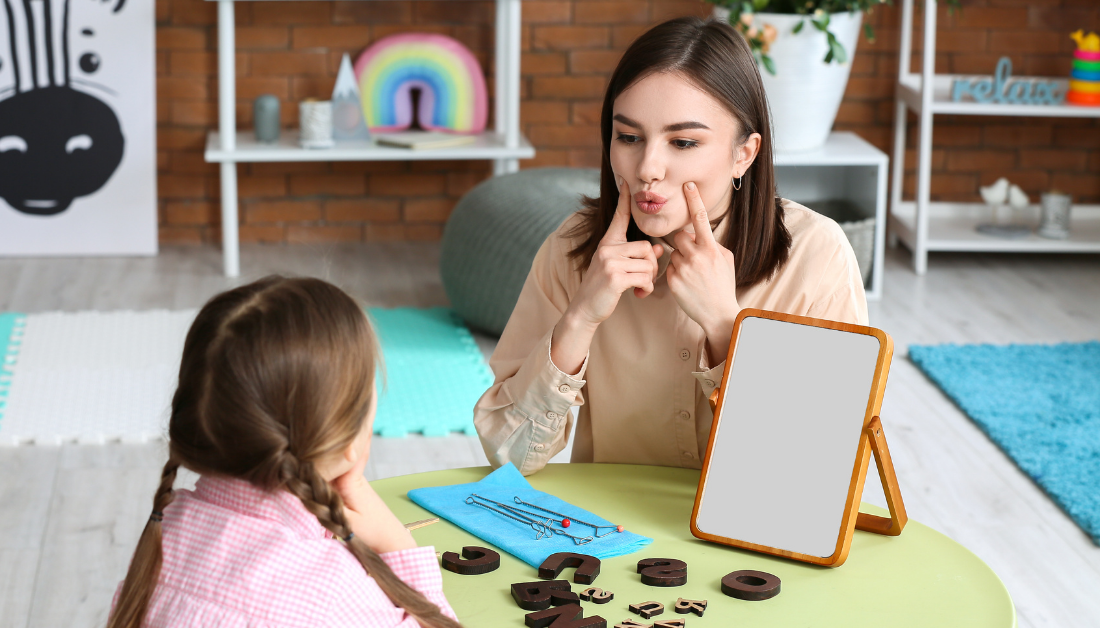
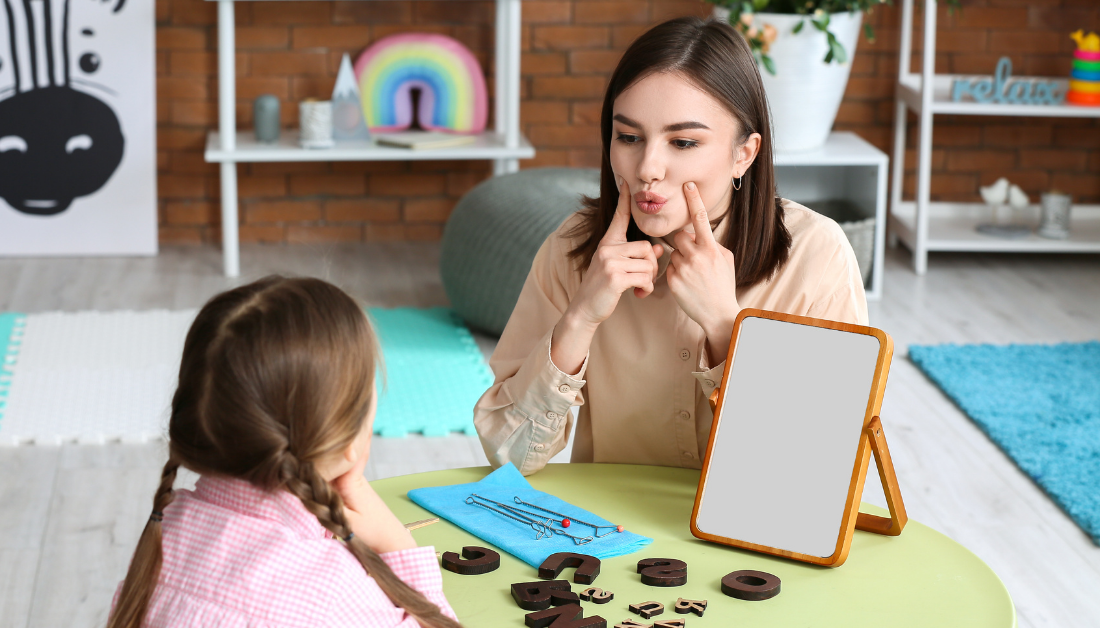
Voice pathology is an issue caused by aberrant abnormalities that create abnormal vibrations in the vocal cords (or vocal folds), such as dysphonia, paralysis, cysts, and even malignancy. In this regard, voice pathology detection (VPD) has attracted a lot of attention as a non-invasive approach to detect vocal issues automatically.
It has two processing modules: a feature extraction module for characterizing normal sounds and a voice detection module for detecting abnormal voices. To obtain good VPD performance, machine learning approaches like as support vector machines (SVM) and convolutional neural networks (CNN) have been effectively used as pathological voice detection modules. A self-supervised, pretrained model can also learn generic and rich speech feature representations rather than explicit speech features, which increases its VPD skills even further. Fine-tuning these models for VPD, on the other hand, results in an overfitting problem due to a domain shift from conversation speech to the VPD job. As a result, the pretrained model becomes overly focused on the training data and fails to generalize well on new data.
To address this issue, a group of researchers led by Prof. Hong Kook Kim from Gwangju Institute of Science and Technology (GIST) in South Korea proposed a groundbreaking contrastive learning method that combines Wave2Vec 2.0-;a self-supervised pretrained model for speech signals-;with a novel approach called adversarial task adaptive pretraining (A-TAPT). They used adversarial regularization during the continuous learning phase in their study.
The researchers conducted several experiments on VPD utilizing the Saarbrucken Voice Database, discovering that the suggested A-TAPT improved the unweighted average recall (UAR) by 12.36% and 15.38% when compared to SVM and CNN ResNet50, respectively. It also had a 2.77% higher UAR than traditional TAPT learning. This demonstrates that A-TAPT is more effective in mitigating the overfitting problem.
Talking about the long-term implications of this work, Mr. Park says who is the first author of this article: “In a span of five to 10 years, our pioneering research in VPD, developed in collaboration with MIT, may fundamentally transform healthcare, technology, and various industries. By enabling early and accurate diagnosis of voice-related disorders, it could lead to more effective treatments, improving the quality of life of countless individuals.”
Their research was published in Volume 30 of the journal IEEE Signal Processing Letters on July 24, 2023. Their research, which was carried out as part of a GIST-funded project called ‘Extending Contrastive Learning to New Data Modalities and Resource-Limited Scenarios’ in collaboration with MIT in Cambridge, MA, USA, sets out on a path that promises to reshape the landscape of VPD and artificial intelligence in medical applications. Hong Kook Kim (EECS, GIST) and Dina Katabi (EECS, MIT) serve as Principal Investigators (PIs), with Jeany Son (AI Graduate School, GIST), Moongu Jeon (EECS, GIST), and Piotr Indyk (EECS, MIT) serving as co-PIs.
Prof. Kim points out: “Our partnership with MIT has been instrumental in this success, facilitating ongoing exploration of contrastive learning. The collaboration is more than a mere partnership; it’s a fusion of minds and technologies that strive to reshape not only medical applications but various domains requiring intelligent, adaptive solutions.”
Furthermore, it holds promise for health monitoring in vocally demanding professions such as call center agents, ensuring robust voice authentication in security systems, improving the responsiveness and adaptability of artificial intelligence voice assistants, and developing tools for voice quality enhancement in the entertainment industry.
Let us hope for more breakthroughs in the fields of self-supervised learning and contrastive learning!
For more information: Adversarial Continual Learning to Transfer Self-Supervised Speech Representations for Voice Pathology Detection. IEEE Signal Processing Letters.
doi.org/10.1109/LSP.2023.3298532.
more recommended stories
Caffeine and SIDS: A New Prevention Theory
For the first time in decades,.
Microbial Metabolites Reveal Health Insights
The human body is not just.
Reelin and Cocaine Addiction: A Breakthrough Study
A groundbreaking study from the University.
Preeclampsia and Stroke Risk: Long-Term Effects
Preeclampsia (PE) – a hypertensive disorder.
Statins and Depression: No Added Benefit
What Are Statins Used For? Statins.
Azithromycin Resistance Rises After Mass Treatment
Mass drug administration (MDA) of azithromycin.
Generative AI in Health Campaigns: A Game-Changer
Mass media campaigns have long been.
Molecular Stress in Aging Neurons Explained
As the population ages, scientists are.
Higher BMI and Hypothyroidism Risk Study
A major longitudinal study from Canada.
Therapeutic Plasma Exchange Reduces Biological Age
Therapeutic plasma exchange (TPE), especially when.
Leave a Comment