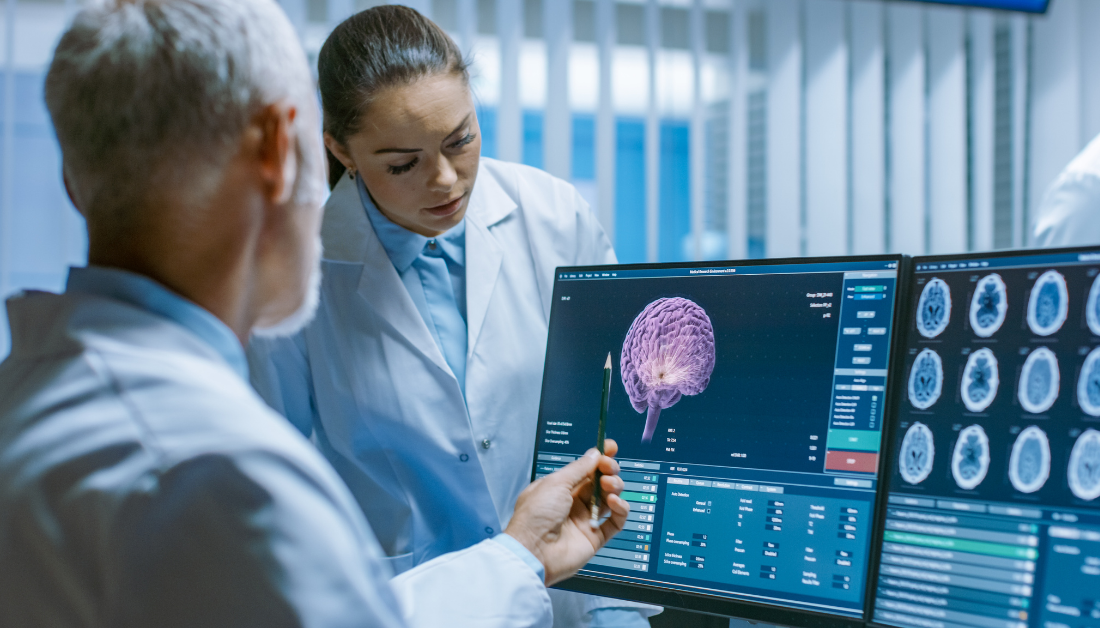
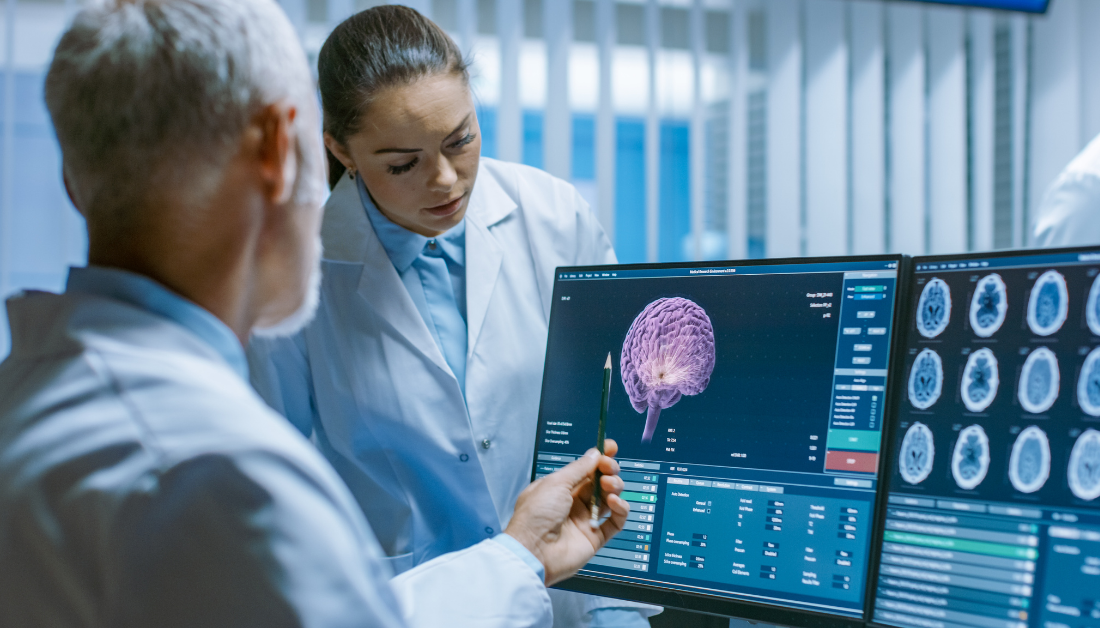
Sturgeon, a patient-agnostic transfer-learned neural network, was built in a recent study published in Nature to enable molecular subclassification of central nervous system (CNS) tumors based on sparsity profiles.
Background
CNS tumors require surgery and must be resected carefully. Preoperative imaging and intraoperative histology analyses, for example, could be erroneous.
Despite the fact that fast nanopore sequencing can help provide a sparse methylation profile during surgery, categorisation remains problematic due to a lack of data and reference samples.
About the Study
Researchers created the Sturgeon machine learning classifier for pediatric and adult CNS tumor categorization in this work, which could be used to improve surgical decision-making.
The researchers created a model for predicting CNS tumor resection using nanopore sequencing data. The dataset, which included 2,801 reference labeled methylation profiles from CNS tumor and normal tissue samples, was separated into four folds for submodel training, validation, and score calibration.
A curriculum learning method was used to train the Sturgeon neural network, starting with easy simulations and proceeding to more difficult ones.
The network was trained using sparsity simulations ranging from 0.6% to 14% and fine-tuned using sparsity simulations ranging from 0.6% to 6.3%. The classifier was fine-tuned at a rate of 104 for 3,000 epochs and verified on 50 batches (containing 12,800 samples) for every 2,000 training batches by computing sensitivity and mean loss.
During inference, samples were classified using four submodels, and the scores from the submodel with the greatest confidence level were used for the final classification. To ensure an equitable distribution of simulated sequencing times, the researchers altered the sequencing sparsity ranges.
After each epoch, the class balance was altered by increasing class upsampling and/or simulation periods for which the machine learning model performed badly. The temperature scaling method was used to calibrate the model.
The researchers created 500 simulated samples from the reference dataset with sparsities ranging from 0.6% to 14%. Extra-realistic nanopore sequencing data was obtained by randomizing the order of the sequenced reads to better examine Sturgeon’s resistance.
To assess its robustness to impure materials, the model was tested using 94 pediatric methylation profiles from persons who had CNS tumor excision surgery at the Princess Máxima Center for Pediatric Oncology.
Results
Sturgeon correctly diagnosed 45 of 50 retrospectively sequenced samples within 40 minutes of starting the sequencing. During 25 procedures, it was effective in real-time, with a diagnostic turnaround time of less than 90 minutes. Of these, 18 (72%) were correct, while seven did not satisfy the appropriate degree of confidence.
Sturgeon’s performance is directly related to sequencing depth, with 0.6% to 4% of the 450K CpG regions covered in the first 50 minutes of simulated sequencing. Temperature scaling reduced the test set’s overall expected calibration error (ECE) from 0.025 to 0.002.
In 25 minutes of sequencing simulations, sturgeon was correctly detected (at the cut-off of 0.8) in 95% of cases with a confirmed diagnosis (32,412 of 34,000 sample simulations).
In 25 minutes of sequencing simulations, sturgeon was correctly detected (at the cut-off of 0.8) in 95% of cases with a confirmed diagnosis (32,412 of 34,000 sample simulations).
Using the 0.95 criterion, 86% of simulated samples were correctly identified (29,316 out of 34,000). After 50 minutes of sequencing simulations, 97% (n=33,020) of model simulations achieved an accurate diagnosis with a confidence level of 0.80 and 91% with a 0.95 score.
Sturgeon was the first to move the computationally complex process of model training, validation, and calibration outside of the surgical time frame, resulting in well-tested, highly accurate one-size-fits-all classification models.
The classifier can be utilized in federated learning settings, with typical turnaround times of 1.5 hours, which corresponds to the surgical timescale. Sturgeon could only perform well in samples with a sufficiently large representation in the training data.
A higher proportion of admixed control readings decreases performance by increasing the number of instances in which the classifier fails to generate a confident classification.
Conclusion
According to the findings of the study, machine-learned diagnosis based on low-cost intraoperative sequencing could assist neurosurgeons in making judgments, perhaps minimizing neurological comorbidity and preventing future treatments.
Sturgeon, a deep learning system trained on simulated nanopore sequencing data, could detect tumor types in the majority of pediatric cases within 25 to 50 minutes of sequencing simulations. It correctly classified 72% of tumors (18 out of 25) at the subclass level after only 45 minutes of sequencing.
Other applications for ultra-fast methylation sequencing include routine post-operative diagnostics, shorter turnaround times, and utilization in remote and low-income institutions. However, the required amount of tissue may be a limitation.
Sturgeon may be used in conjunction with histological assessment by a trained pathologist to provide a more accurate intraoperative diagnosis by merging histological and molecular findings. The model could help guide decision-making in tough situations where the histological diagnosis is ambiguous.
For more information: Ultra-fast deep-learned CNS tumor classification during surgery, Nature (2023)
https://doi.org/10.1038/s41586-023-06615-2
more recommended stories
lncRNAs in Tumor Microenvironment Drive Cancer Progression
The tumor microenvironment (TME) is a.
Lyme Disease Symptoms: Why They Linger After Treatment
Lyme disease symptoms that persist after.
Body Image in Young Women: Cultural Influences Matter
A new international study led by.
Early Alzheimer’s Found Through Blood Biomarkers
A new study published in Molecular.
Label-Free Motion Analysis Detects Cancer Cells
Label-free motion analysis is emerging as.
Harmful Chemicals in Children’s Mattresses
A recent study has brought to.
TMS for Post-Stroke Aphasia Shows Remarkable Gains
A new clinical study led by.
CT Scan Overuse May Cause 1 in 20 New U.S. Cancers
A recent study published in JAMA.
Lactation Metabolism: Brain-Hormone Link Uncovered
Understanding how the body adjusts to.
Quantum Technology in Cancer Surgery: New Probe Aims to Improve Outcomes
A groundbreaking project from the University.
Leave a Comment