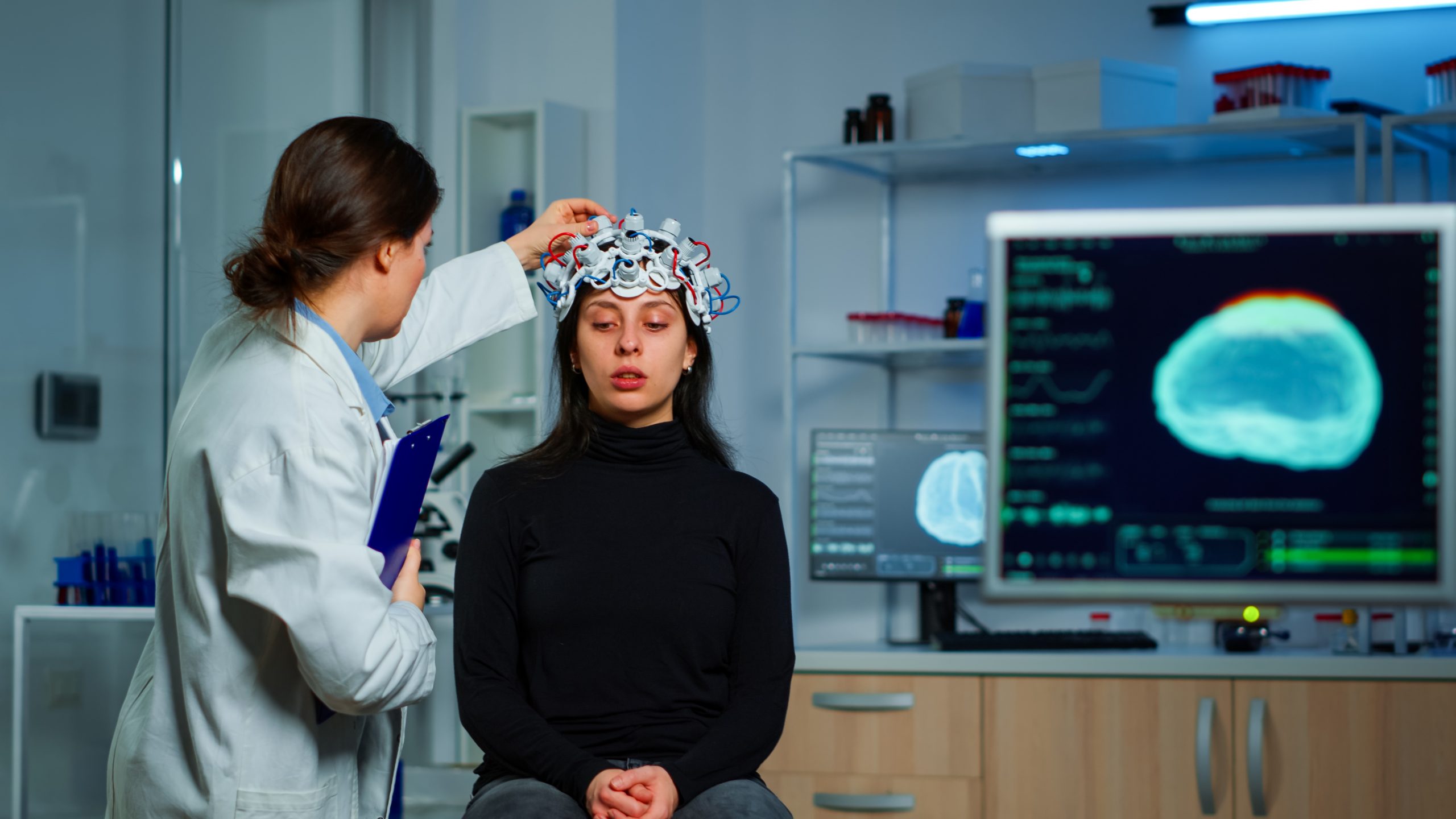
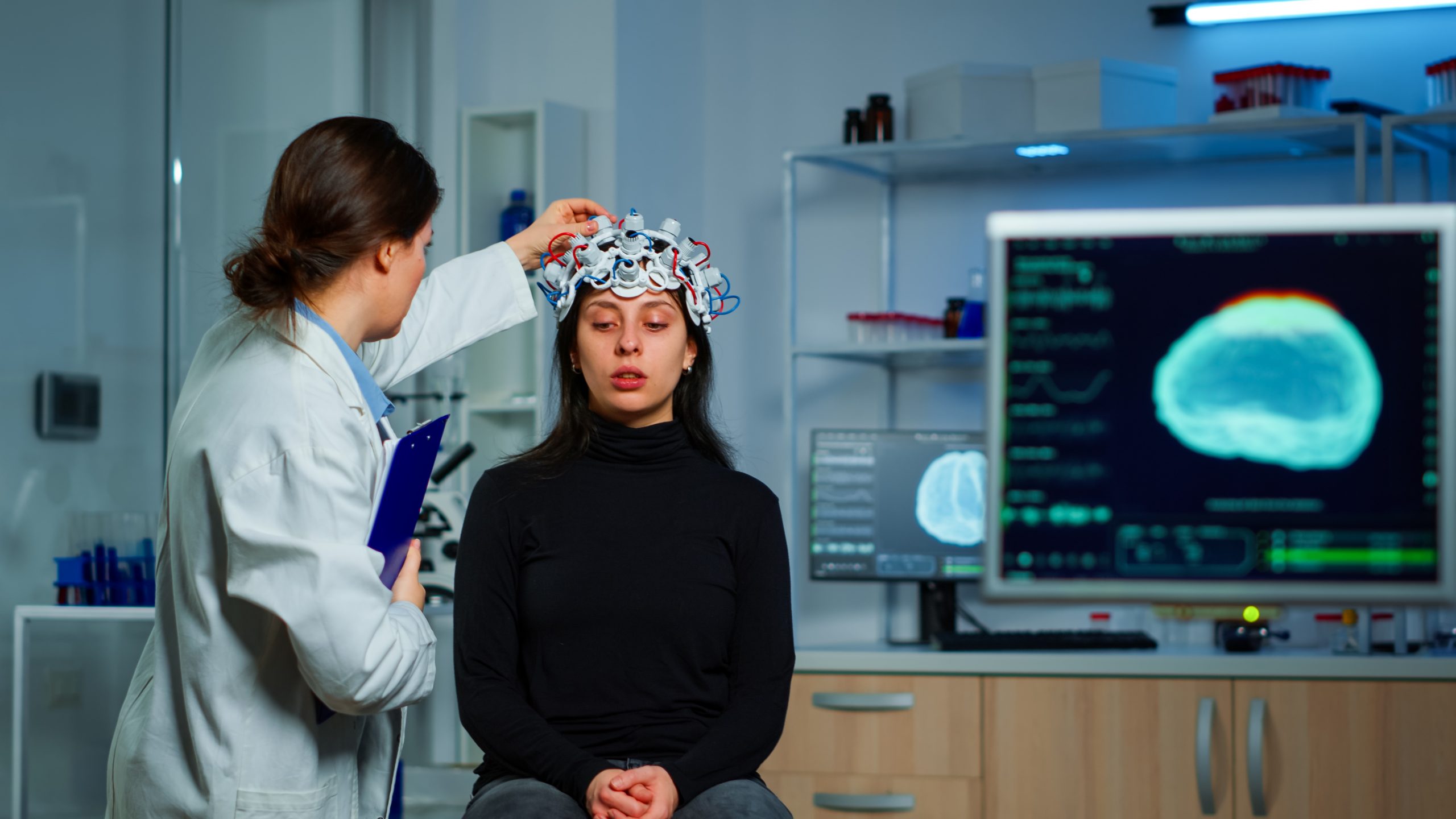
In recent years, research on traumatic brain injury has proliferated, from the football field to the battlefield. Modeling the mechanical forces that compress, strain, and twist the brain tissue and cause harm that can be temporary or fatal is essential to understanding brain injury.
Stanford University researchers claim to have used artificial intelligence to create a model of how brain deformations translate into stresses. They believe their method may help explain why and when concussions sometimes result in permanent brain damage while other times they do not.
“The problem in brain modeling to date is that the brain is not a homogeneous tissue—it’s not the same in every part of the brain. Yet, trauma is often pervasive,” said Ellen Kuhl, professor of mechanical engineering, director of the Living Matter Lab, and senior author of a new study appearing in the journal Acta Biomaterialia. “The brain is also ultrasoft, much like Jell-O, which makes both testing and modeling physical effects on the brain very challenging.”
Going to the library
Researchers who want to study brain trauma are forced to select from a library of dozens of material models, some dating back almost a century, to help calculate the stresses on the brain.
For decades, scientists have developed these models for soft materials with inscrutable names like the “neo-Hookean Model for Plastics and Rubber,” the “Demiray Model for Soft Tissues,” and the “Ogden Model for Rubber-like Solids.” But a model that works for a certain type of stress—tension, compression, or shear—may not work for another. Similarly, a model that might work for one region of the brain might not work for another.
The new approach takes a model-of-models tack, using artificial intelligence to discover which model, out of more than 4,000 possibilities, best explains the behavior of the brain. In the past, selecting the best model was a hit-or-miss process that depended largely on user experience and personal preference.
“We take user selection out of the equation by allowing machine learning to examine the data and decide which model works best,” adds Sarah St. Pierre, a doctoral scholar in Kuhl’s lab and a co-author of the paper. “Automating this process lowers barriers to model the brain. Now, every Stanford student can do this.” Once machine learning has discovered the best model, it’s easy to relate it back to the models that generations of researchers have proposed.
Transformative insights
The approach, known as a Constitutive Artificial Neural Networks, was developed by Kevin Linka before he joined the Living Matter Lab as a postdoc to apply his method to the brain.
“We provide the network with all existing constitutive models developed over the last century. And the AI does a mix-and-match to find the best option. This is impossible to do by hand,” Linka said. “Now, we’ve effectively discovered a new model that makes us more confident in studying the mechanical stress in the brain.”
Constitutive Artificial Neural Networks, in contrast to traditional off-the-shelf neural networks, offer fresh perspectives on the physics of the brain. The team cites as just one illustration how they have precisely measured physically significant factors like variable shear stiffnesses in the cortex, basal ganglia, corona radiata, and corpus callosum at precisely 1.82, 0.88, 0.94, and 0.54 kilopascals respectively.
The force from a blow to the head, for example, and the ensuing deformation of the brain tissue are related by the shear modulus. By these standards, the corpus callosum, the network of nerves that connects the two hemispheres of the brain, is more than three times as stiff as the cortex, the grey, outer layer of the brain.
Researchers studying brain damage now have better expertise to recreate and comprehend the origins of brain injury. This may serve as motivation for developing additional safeguards or curative procedures. Kuhl’s team has worked with Dassault Systemès Simulia, a leading provider of simulation software, to integrate automated model discovery right into their analysis workflow in order to apply this knowledge to engineering practice.
“What’s really most exciting about this research,” Kuhl said, “is that Constitutive Artificial Neural Networks could induce a paradigm shift in soft tissue modeling, from user-defined model selection to automated model discovery. This could forever change how we simulate materials and structures.”
more recommended stories
Affordable AI Tool Predicts Cancer Immunotherapy Outcomes
Researchers from Memorial Sloan Kettering Cancer.
Inogen’s SIMEOX 200 Gets FDA Clearance for Airway Care
Inogen, Inc., a medical technology company.
AI System Tracks Emerging infectious Disease
Researchers have developed a novel method.
Psilocybin Helps Reduce Anxiety, Depression During Cancer
Groundbreaking research from NYU Langone Health.
New Gene Delivery Methods Offer Hope for CKD Patients
Researchers from Oregon Health & Science.
Laser-Textured Stents: Breakthrough for Vascular Health
Dr. Hojeong Jeon and Dr. Hyung-Seop.
SPLICER: A Breakthrough in Alzheimer’s Gene Therapy
A new gene editing technique –.
New MRI Technique Predicts Heart Disease Risk
Everyone is aware of the health.
Eye Drops for Dry AMD: A Non-Invasive Breakthrough
Age-related macular degeneration (AMD), the leading.
Revolutionary Cancer Therapy: Cells Turned Normal
In a revolutionary breakthrough, scientists at.
Leave a Comment