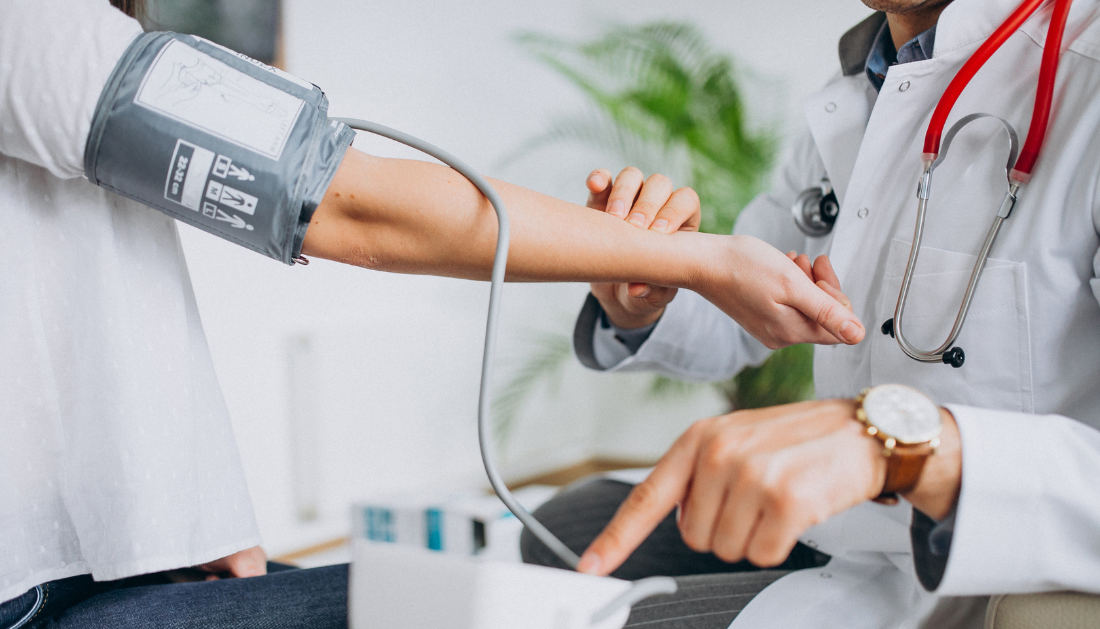
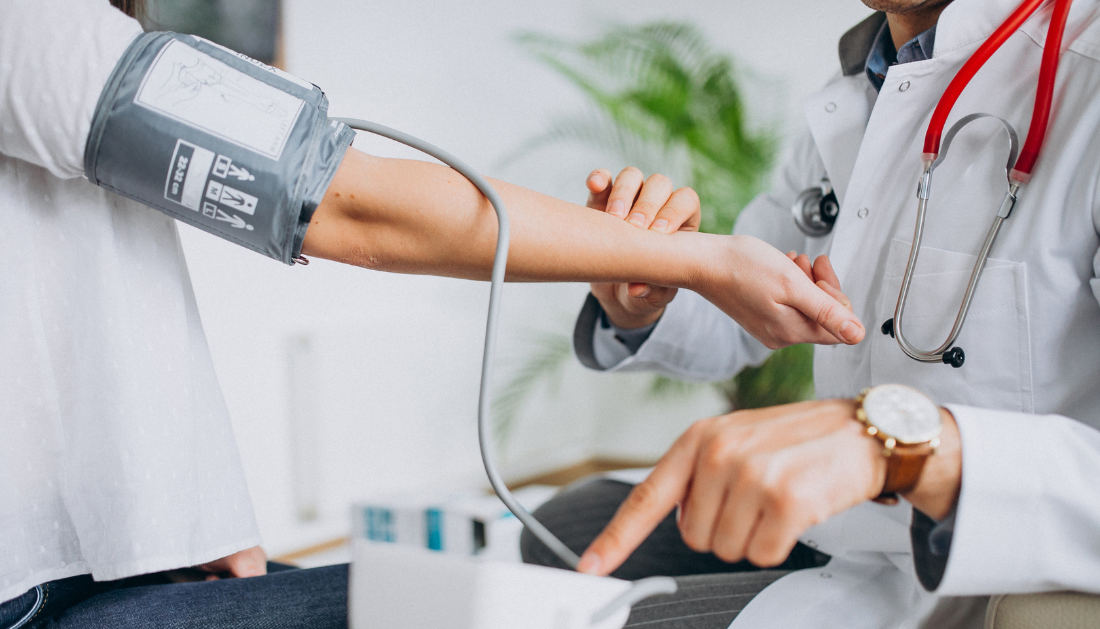
Researchers analyzed current breakthroughs in artificial intelligence (AI) technologies for treating hypertension (HTN) and associated comorbidities in a recent article published in Hypertension Research.
Background
They analyze more than 35 research and conclude that recent developments in home blood pressure (BP) monitoring, as well as improved integration of varied data sources, may lead to the creation of individualized machine-learning solutions for HTN risk assessment, diagnosis, and therapy.
They go on to discuss the existing problems in the sector and propose that expanded multidisciplinary cooperation between AI experts, healthcare providers, and clinicians be implemented as the way ahead for AI’s increased applicability in HTN treatment.
Why do we need AI for Hypertension care?
High blood pressure (>140/90 mmHg) is a common symptom of hypertension (HTN). Patients’ hearts must work much harder than usual throughout the condition to guarantee appropriate blood supply to various somatic aspects.
While HTN is rarely fatal on its own, it is linked to a slew of cardiovascular complications, including heart disease and stroke.
Unfortunately, HTN is typically a silent disease, with few outwardly evident signs. Although over half of adults suffer from the disorder, 30% or more are unaware of it, making diagnosis and management difficult.
In recent years, advances in computational power and the influx of artificial intelligence (AI) into medical research have stifled significant growth in the field, with AI proponents claiming that machine learning (ML) models can alleviate the burden on the limited availability of cardiovascular experts by taking the lead in HTN risk assessment and diagnosis.
Several critical advancements have paved the way for the influx of ML models in HTN research, most notably the extensive recent integration of previously distinct data sources – electronic health records, environmental data, and personalized health records, which were previously analyzed separately – have provided ML models with the raw materials needed to reveal population- and individual-specific patterns in HTN pathology.
Notable is the growing popularity of smartwatches and other wearable data loggers, which, while not as precise as clinical instruments, provide high-density and continuous data on lifetime and other important indicators such as heart rate, physical activity levels, and blood pressure (BP).
Finally, deep-learning algorithms, which represent a considerable advancement above typical AI models, have allowed researchers to attain exceptional accuracy in HTN and BP diagnosis by recognizing detailed patterns in multidisciplinary data.
This has led in enhanced personalization of these treatments, which may assist patients by adapting clinical interventions to their unique needs rather than using a ‘one-size-fits-all’ approach.
About the study
In the current study, researchers reviewed over 35 recent articles on the use of AI in HTN risk assessment, diagnosis, and treatment. The study focuses on modeling methodologies that leverage high-density home blood pressure data, as well as research stressing explainability for individualized treatment.
“Home BP is strongly associated with the development of cardiovascular diseases and is the most important index for HTN management”
Big data – advances in BP measurements and their role in AI development
Hypertension patients used to record two or more blood pressure readings (typically in the morning and evening), which were documented in a ‘BP diary’ for subsequent examination by a clinician.
The shrinking size of BP measurement equipment, as well as the growing popularity of wearables (e.g., smart watches) with integrated BP measurement, have resulted in the rise of data digitization and Big Data.
Many recent studies have emphasized the benefits of wearables and smartphone applications for blood pressure treatment support.
However, these techniques have a critical challenge: accuracy validation. Fleischhauer et al. and Tan et al. have developed novel measurement procedures, including as photoplethysmography (PPG) and deep learning, to enhance wearable accuracy (average error rates <7 mmHg). However, these methodologies lack clinical validation.
The European Society of Hypertension (ESH) has gone so far as to validate cuffless blood pressure monitors independently of their conventional cuff equivalents.
“Currently, cuffless BP monitors are not intended to replace traditional BP monitors using cuff. Further validation of the device’s performance as a measurement device and its clinical efficacy and effectiveness is needed to clarify its clinical position.”
Despite this obstacle, cuffless BP monitors have supplied a large digital dataset for training and developing AI models. Combining constantly logged blood pressure data with easily available hospital test values, health records, and demographic data has resulted in the development of ‘time-series’ studies capable of predicting HTN risk months, if not years, before the disease starts.
“If AIs can predict future BP values based on past BP trends, doctors will know when BP levels exceed the HTN criteria at an early stage. Li et al. developed an AI that sequentially predicts mean BP values every month for three months using BP, BMI, sex, age, latitude, and longitude”
Personalized Hypertension treatment – the AI advantage
One of the most compelling arguments against utilizing AI in medical research was its lack of both accuracy and explainability.
LIME (2016) and SHAP (2017) addressed these concerns by adding the concept of “local explanation,” which eliminates the need for generalization as a condition for explainability.
This concept, encouragingly, accidentally brought in the age of personalized, AI-guided patient care, in which personal data is used to inform clinical interventions rather than the previous ‘one-size-fits-all’ medical procedures.
“In particular, for personalized medicine aimed at preemptive disease prevention, it is essential to encourage lifestyle modifications in response to early signs of disease. Accordingly, it is expected that other XAI methods and comprehensive systems utilizing machine learning models will be implemented in addition to technologies such as SHAP.”
Individual conditional expectancies (ICE) and counterfactual explanations (CE) are two unique concepts driving personalized predictive healthcare. When a patient’s present body metrics indicate a future risk of HTN or a comparable disease, these principles enable AI to model the variables and behavioral changes needed to reduce or mitigate that risk.
Modification feasibility is a current difficulty with these approaches. Just because a series of alterations can minimize HTN risk does not indicate the patient can readily implement those ‘ideal’ lifestyle changes.
The integration of SHAP technologies with ICEs and other AI techniques in the future may enable the development of guided AI interventions that provide recommendations informed by the patient’s health records and daily routine, allowing for small cumulative modifications with synergistic effects against HTN.
Conclusions
The current paper covers the advancements, problems, and prospects of AI in HTN risk assessment, tailored treatment, and diagnosis.
AI can assist or even replace human medical practitioners in some of these disciplines, but its novelty and lack of clinical validation present significant limitations.
Nonetheless, deep learning models are increasingly being built to improve patient well-being, aided by the ever-increasing digital outputs of smart gadgets and wearables, which log real-time patient data in unprecedented ways.
With targeted studies aimed at overcoming present measurement accuracy constraints, we are closer than ever to a future in which our smartwatches and smartphones recommend lifestyle modifications aimed at enhancing our overall health.
For more information: Kohjitani, H., Koshimizu, H., Nakamura, K. et al. (2024) Recent developments in machine learning modeling methods for hypertension treatment. Hypertens Res. doi: https://doi.org/10.1038/s41440-023-01547-w.
more recommended stories
Early Brain Development: Gene Control Atlas Unveiled
An atlas of the brain’s early.
PLS Riskier in ABO Mismatched Lung Transplants
Passenger lymphocyte syndrome (PLS) is a.
Researcher Develops MRI Scan Error Detection Sensor
The world’s first sensor prototype that.
Lysosomal Function in Parkinson’s Disease Risk
The genetic traits that affect the.
Omega-6 Fatty Acid Role in Bipolar Disorder
Higher concentrations of arachidonic acid, an.
Transplant Drug Shows Promise in Slowing Alzheimer’s in Seizure Patients
Protein imbalances, which heighten the excitability.
Fentanyl Inhalation: Brain Damage Risks
After treating a middle-aged man who.
Enhanced Immune Response in New COVID-19 Vaccines
An improved vaccination offers an enhanced.
Bioresorbable Wireless Sensor for Early Stomach Leakage Detection
In a recent publication in Scientific.
Neurocardiac Connectivity in Depression Treatment
Heart rate deceleration and sadness may.
Leave a Comment