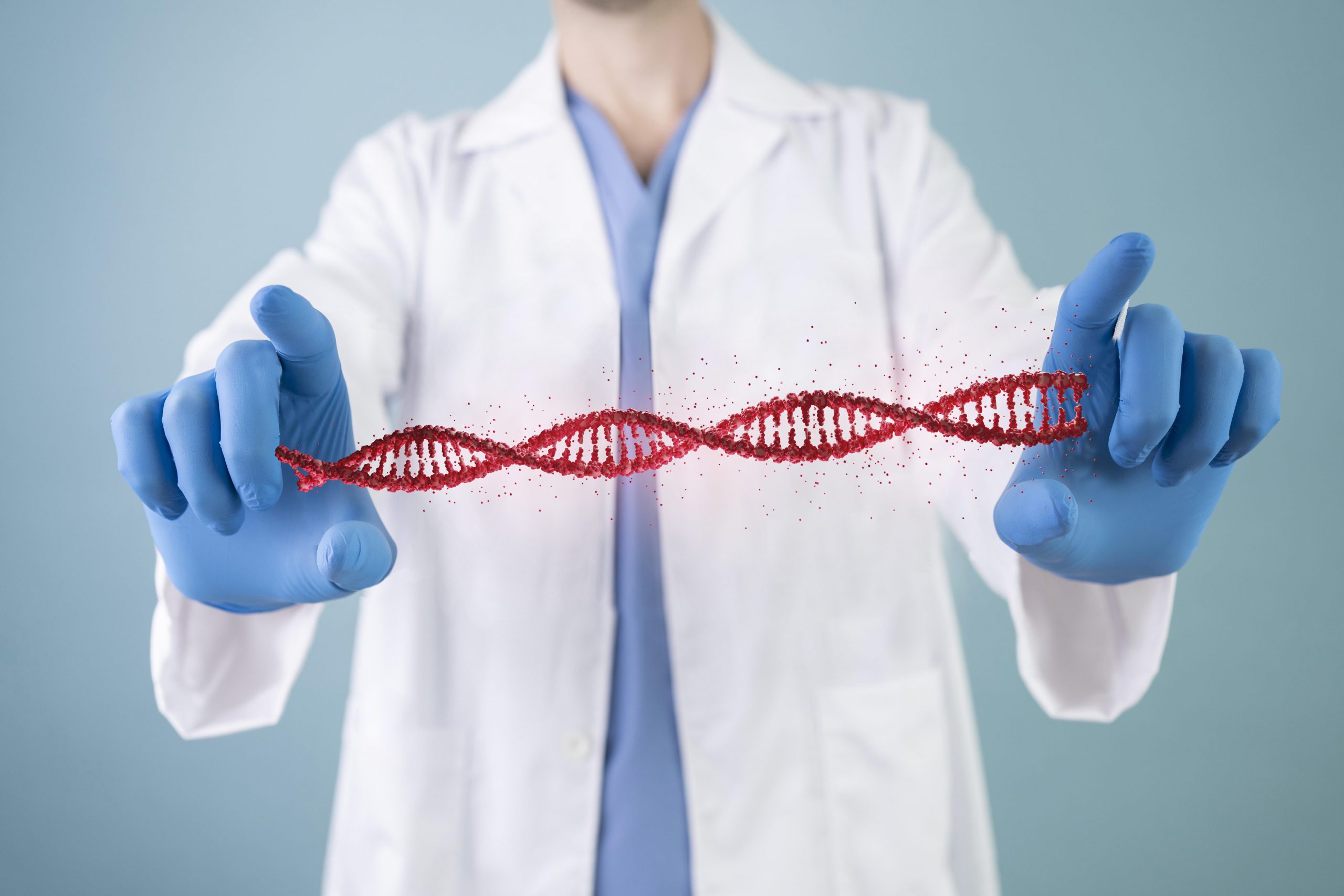
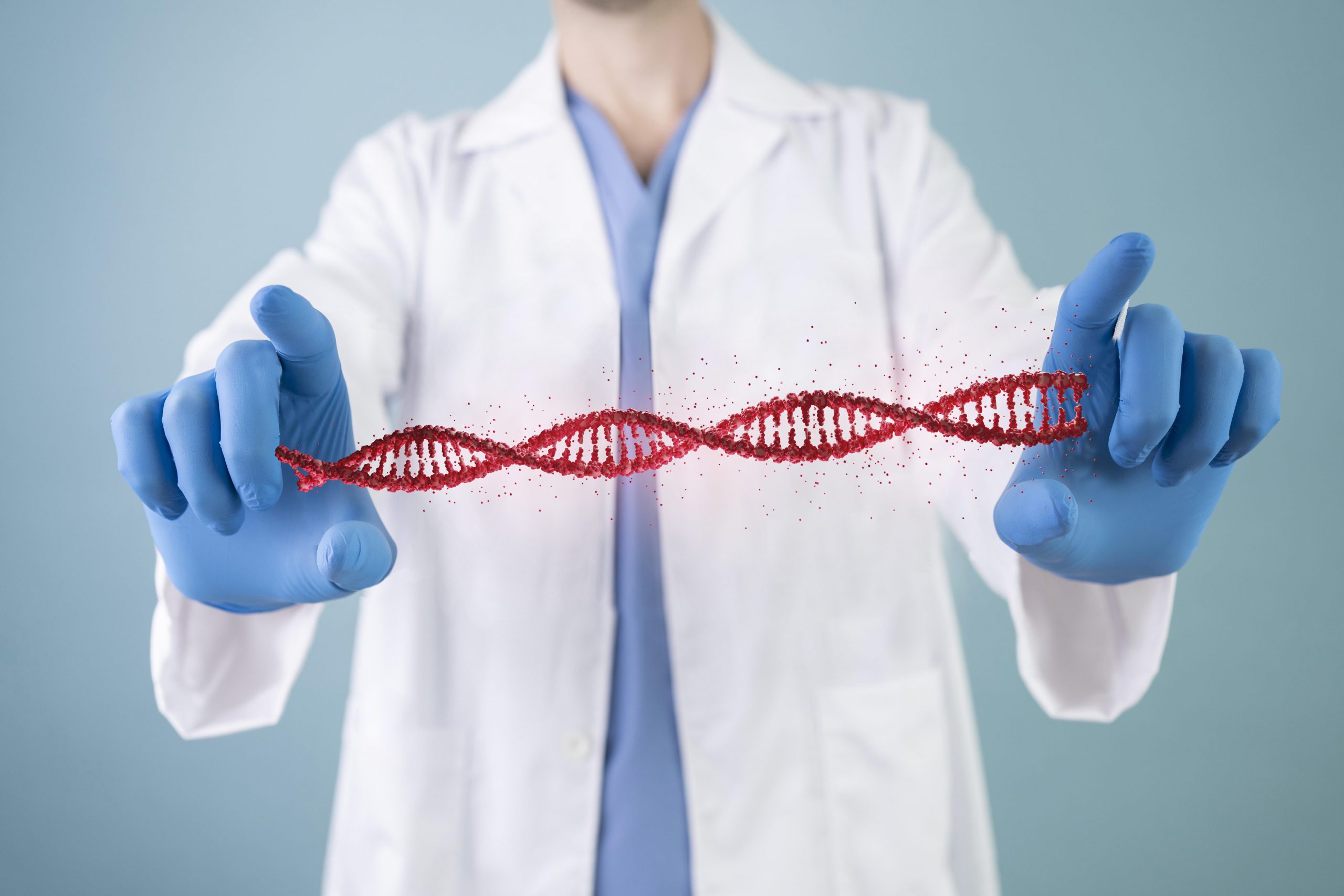
Artificial intelligence (AI) has been used by researchers at Gladstone Institutes, the Broad Institute of MIT and Harvard, and the Dana-Farber Cancer Institute to help them understand how large networks of interconnected human genes control the function of cells, and how disruptions in those networks cause disease.
Large language models, also known as foundation models, are AI systems that learn fundamental knowledge from huge volumes of general data and then use that knowledge to perform new tasks, a process known as transfer learning. These days, AI tools have been used in many surgical diagnosis interventions. With the publication of ChatGPT, a chatbot based on an OpenAI model, these systems have lately attracted mainstream notice.
Gladstone Assistant Investigator Christina Theodoris, MD, Ph.D., built a fundamental model for understanding how genes interact in the current study, which was published in the journal Nature. The new Geneformer model learns from vast quantities of data on gene interactions from a wide range of human tissues and applies this information to forecast how things can go wrong in disease.
Theodoris and her colleagues used Geneformer to investigate how cardiac cells malfunction in heart disease. This approach, on the other hand, is applicable to a wide range of other cell types and disorders.
“Geneformer has vast applications across many areas of biology, including discovering possible drug targets for disease,” says Theodoris, who is also an assistant professor in the Department of Pediatrics at UC San Francisco. “This approach will greatly advance our ability to design network-correcting therapies in diseases where progress has been obstructed by limited data.”
X. Shirley Liu, Ph.D., former director of the Center for Functional Cancer Epigenetics at Dana-Farber Cancer Institute, and Patrick Ellinor, MD, Ph.D., director of the Cardiovascular Disease Initiative at the Broad Institute—both authors of the new study—designed Geneformer during a postdoctoral fellowship.
A Network Diagram
Many genes, when activated, initiate molecular cascades that cause other genes to increase or decrease their activity. Some of those genes, in turn, influence other genes—or loop back and inhibit the first gene. As a result, when a scientist draws the links between a few dozen related genes, the resulting network map frequently resembles a tangled spiderweb.
If mapping out a handful of genes in this manner is difficult, trying to identify links between all 20,000 genes in the human genome is a daunting task. However, such a large network map would provide researchers with insight into how entire networks of genes change in disease, as well as how to reverse those changes.
“If a drug targets a gene that is peripheral within the network, it might have a small impact on how a cell functions or only manage the symptoms of a disease,” says Theodoris. “But by restoring the normal levels of genes that play a central role in the network, you can treat the underlying disease process and have a much larger impact.”
‘Transfer learning’ in artificial intelligence
Typically, researchers use large datasets with many comparable cells to map gene networks. They employ a subset of AI systems known as machine learning platforms to identify patterns in data. A machine learning system, for example, may be trained on a large number of samples from individuals with and without heart disease, and then discover the gene network patterns that distinguish diseased samples from healthy ones.
However, standard machine learning models in biology are only taught to do one task. The models must be retrained from beginning on new data in order to perform a particular task. So, if the first example’s researchers wanted to distinguish damaged kidney, lung, or brain cells from their healthy counterparts, they’d have to start from scratch and train a new algorithm with data from those tissues.
The problem is that there isn’t enough existing data for some diseases to train these machine learning algorithms.
Theodoris, Ellinor, and their colleagues addressed this issue in the new study by using a machine learning technique known as “transfer learning” to train Geneformer as a fundamental model whose essential information can be transferred to other tasks.
First, they “pre-trained” Geneformer to understand how genes interact by feeding it data on gene activity levels in around 30 million cells from a variety of human tissues.
To show that the transfer learning approach was effective, the scientists fine-tuned Geneformer to make predictions about gene relationships or if lowering the amounts of specific genes will cause disease. Because of the underlying knowledge it obtained during the pretraining process, Geneformer was able to generate these predictions with far greater accuracy than competing methodologies.
Furthermore, even when only presented a small number of examples of relevant data, Geneformer was able to produce appropriate predictions.
“This means Geneformer could be applied to make predictions in diseases where research progress has been slow because we don’t have access to sufficiently large datasets, such as rare diseases and those affecting tissues that are difficult to sample in the clinic,” says Theodoris.
Heart disease lessons
Theodoris’ team then set out to apply transfer learning to advance heart disease research. They initially asked Geneformer to forecast which genes might be harmful to the formation of cardiomyocytes, or heart muscle cells.
Many of the top genes found by the model had previously been linked to heart disease.
“The fact that the model predicted genes that we already knew were really important for heart disease gave us additional confidence that it was able to make accurate predictions,” says Theodoris.
Other potentially relevant genes found by Geneformer, such as TEAD4, had not previously been linked to heart disease. TEAD4 was eliminated from cardiomyocytes in the lab, and the cells were no longer able to beat as vigorously as healthy ones.
As a result, Geneformer employed transfer learning to reach a novel conclusion: despite not being supplied any knowledge on cells lacking TEAD4, it accurately anticipated TEAD4’s importance in cardiomyocyte function.
Finally, the researchers requested Geneformer to identify which genes should be targeted at the gene network level to make damaged cardiomyocytes resemble healthy cells. When the researchers tested two of the proposed targets in cells damaged by cardiomyopathy (a condition of the heart muscle), they discovered that utilizing CRISPR gene editing technology to remove the predicted genes restored the beating ability of diseased cardiomyocytes.
“In the course of learning what a normal gene network looks like and what a diseased gene network look like, Geneformer was able to figure out what features can be targeted to switch between the healthy and diseased states,” says Theodoris. The transfer learning approach allowed us to overcome the challenge of limited patient data to efficiently identify possible proteins to target with drugs in diseased cells.
A benefit of using Geneformer was the ability to predict which genes could help to switch cells between healthy and disease states,” says Ellinor. “We were able to validate these predictions in cardiomyocytes in our laboratory at the Broad Institute.”
The researchers intend to increase the quantity and types of cells studied by Geneformer in order to improve its ability to evaluate gene networks. They’ve also made the model publicly available so that other scientists can utilize it.
“With standard approaches, you have to retrain a model from scratch for every new application,” says Theodoris. “The really exciting thing about our approach is that Geneformer’s fundamental knowledge about gene networks can now be transferred to answer many biological questions, and we’re looking forward to seeing what other people do with it.”
more recommended stories
T-bet and the Genetic Control of Memory B Cell Differentiation
In a major advancement in immunology,.
Ultra-Processed Foods May Harm Brain Health in Children
Ultra-Processed Foods Linked to Cognitive and.
Parkinson’s Disease Care Advances with Weekly Injectable
A new weekly injectable formulation of.
Brain’s Biological Age Emerges as Key Health Risk Indicator
Clinical Significance of Brain Age in.
Children’s Health in the United States is Declining!
Summary: A comprehensive analysis of U.S..
Autoimmune Disorders: ADA2 as a Therapeutic Target
Adenosine deaminase 2 (ADA2) has emerged.
Is Prediabetes Reversible through Exercise?
150 Minutes of Weekly Exercise May.
New Blood Cancer Model Unveils Drug Resistance
New Lab Model Reveals Gene Mutation.
Healthy Habits Slash Diverticulitis Risk in Half: Clinical Insights
Healthy Habits Slash Diverticulitis Risk in.
Caffeine and SIDS: A New Prevention Theory
For the first time in decades,.
Leave a Comment