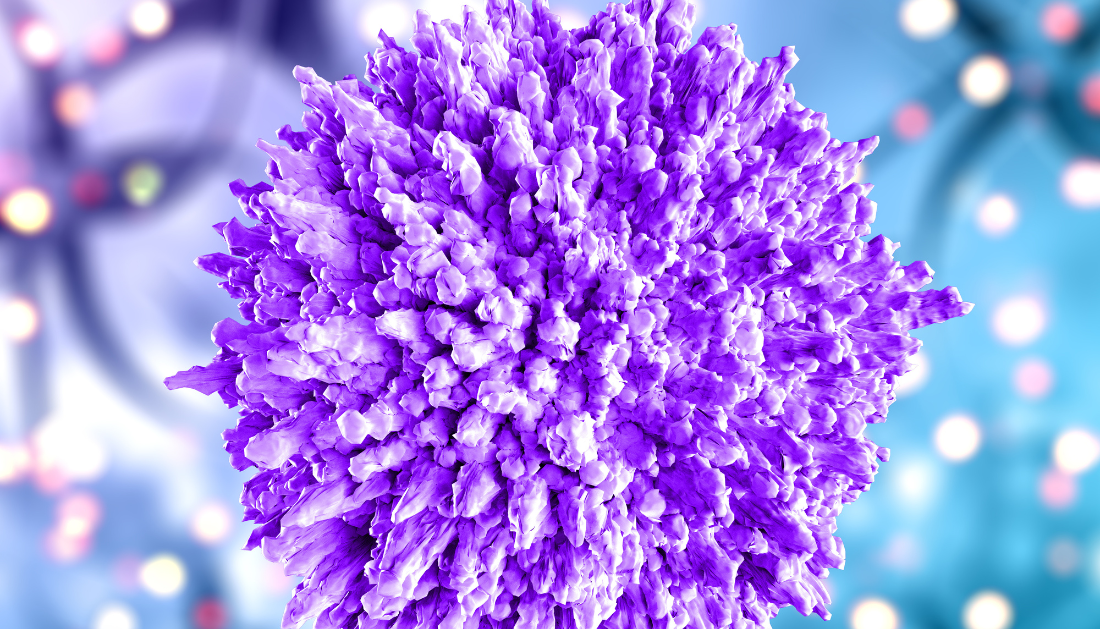
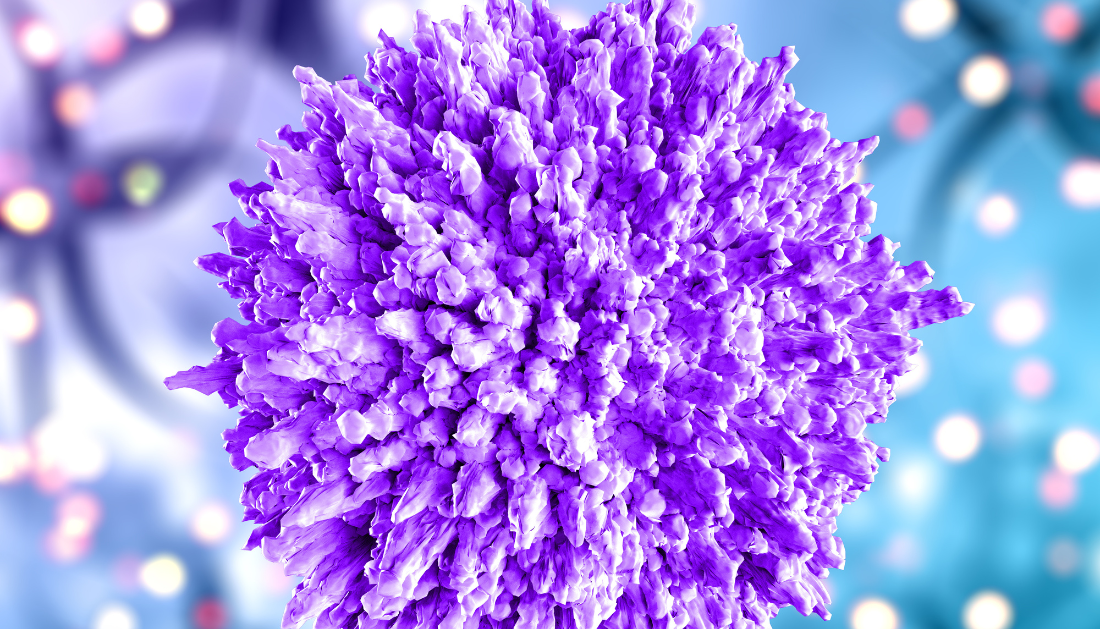
Study: PERCEPTION: Predicting patient treatment response and resistance via single-cell transcriptomics of their tumors
more recommended stories
Children’s Health in the United States is Declining!
Summary: A comprehensive analysis of U.S..
Autoimmune Disorders: ADA2 as a Therapeutic Target
Adenosine deaminase 2 (ADA2) has emerged.
Is Prediabetes Reversible through Exercise?
150 Minutes of Weekly Exercise May.
New Blood Cancer Model Unveils Drug Resistance
New Lab Model Reveals Gene Mutation.
Healthy Habits Slash Diverticulitis Risk in Half: Clinical Insights
Healthy Habits Slash Diverticulitis Risk in.
Caffeine and SIDS: A New Prevention Theory
For the first time in decades,.
Microbial Metabolites Reveal Health Insights
The human body is not just.
Reelin and Cocaine Addiction: A Breakthrough Study
A groundbreaking study from the University.
Preeclampsia and Stroke Risk: Long-Term Effects
Preeclampsia (PE) – a hypertensive disorder.
Statins and Depression: No Added Benefit
What Are Statins Used For? Statins.
Leave a Comment