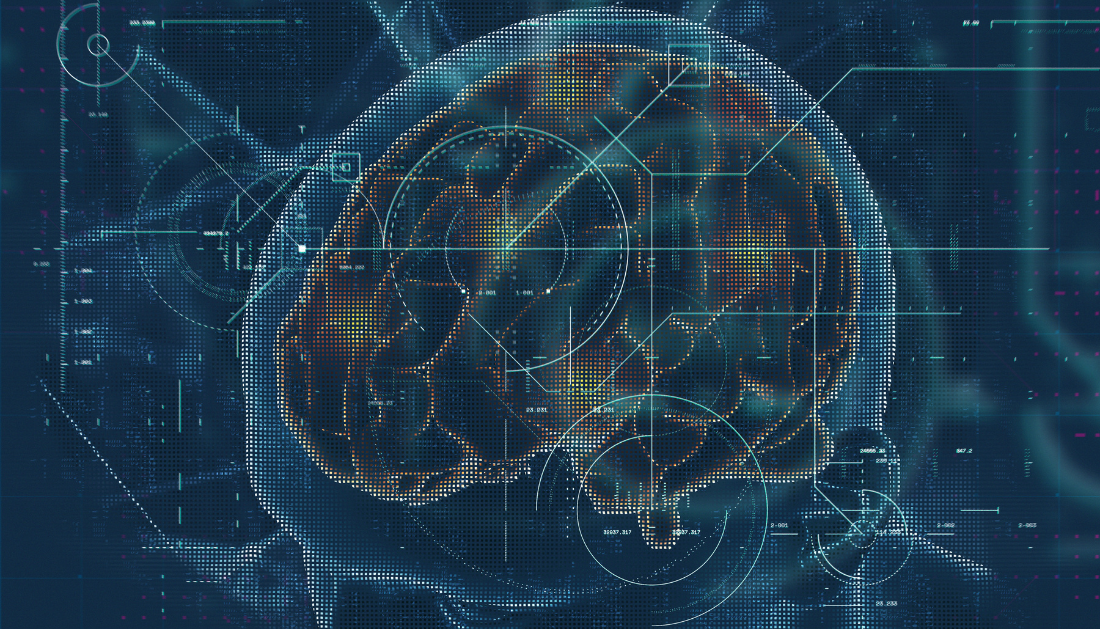
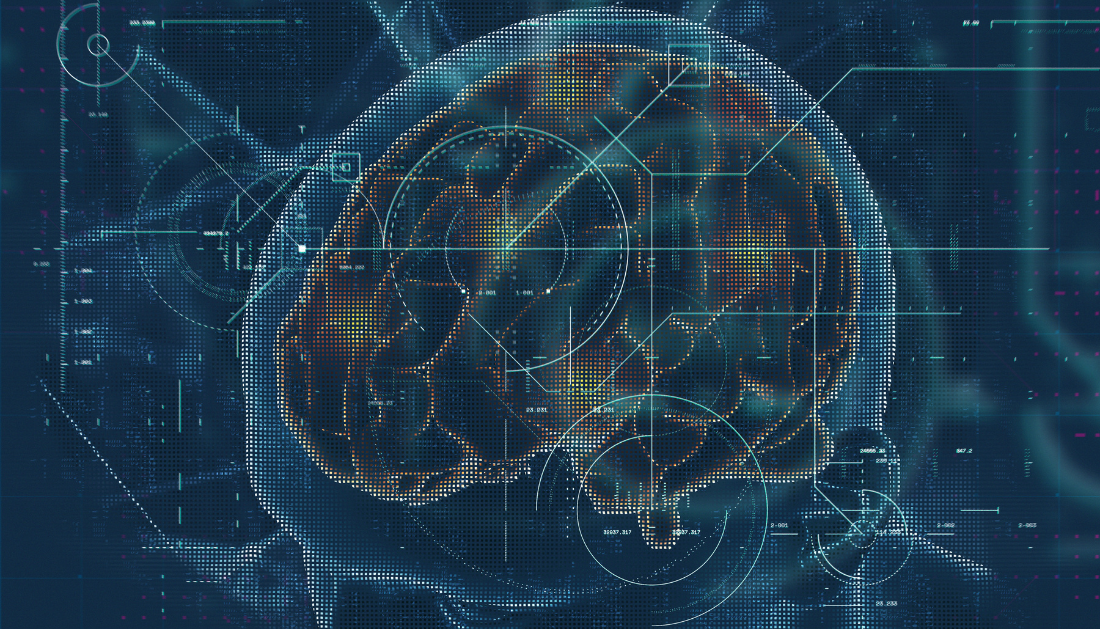
Researchers created and verified an Artificial Intelligence (AI) model that employs multimodal data to reliably distinguish between different dementia (significant cognitive decline) etiologies for better early and individualized management in a recent study that was published in the journal Nature Medicine.
Know more about the Artificial Intelligence and Devices in Dementia at the Global Neurology Summit 2024.
Context
About 10 million people suffer from dementia each year, and it presents serious clinical and financial difficulties. Accurate diagnosis is necessary for successful therapy, but it can be difficult because different types of symptoms often overlap. The need for better tools intensifies as populations age and the necessity for precise diagnostics in drug trials increases. The problem is made worse by the lack of experts, which emphasizes the need for scalable solutions. To assess the AI model’s influence on healthcare outcomes and clinical practice integration, more investigation is required.
About the study
51,269 participants from nine cohorts were included in the current study, and extensive data was gathered on them, including demographics, medical histories, lab results, physical and neurological examinations, medications, neuropsychological testing, functional assessments, and multisequence MRI scans. Written informed consent was given by participants or their informants, and institutional ethical review committees authorized the protocols. The group comprised people with dementia (22,063), mild cognitive impairment (MCI) (slight cognitive decline, 9,357), and normal cognition (NC) (healthy brain function, 19,849).
Alzheimer’s disease (AD) (memory loss dementia; 17,346), Lewy body (hallucinations and motor issues) and Parkinson’s disease (movement disorder with dementia; 2,003), vascular dementia (VD) (cognitive decline from reduced brain blood flow; 2,032), prion disease (PRD) (rapid neurodegenerative disorder; 114), frontotemporal dementia (FTD) (personality and language decline; 3,076), normal pressure hydrocephalus (NPH) (fluid buildup causing dementia-like symptoms; 138), dementia due to systemic and external factors (SEF, 808), psychiatric diseases (PSY, 2,700), traumatic brain injury (TBI, 265), and other causes (ODE, 1,234) were further subclassified.
The research made use of information from the following sources: Australian Imaging, Biomarker and Lifestyle Flagship Study of Ageing (AIBL), 4 Repeat Tauopathy Neuroimaging Initiative (4RTNI), Lewy Body Dementia Center for Excellence at Stanford University (LBDSU), the Framingham Heart Study (FHS), Alzheimer’s Disease Neuroimaging Initiative (ADNI), Frontotemporal Dementia (FTD) Neuroimaging Initiative (NIFD), Parkinson’s Progression Marker Initiative (PPMI), and the Framingham Heart Study (FHS). NC, MCI, or dementia diagnosis was necessary for eligibility, and NACC data served as the baseline. The Uniform Data Set (UDS) dictionary was utilized to normalize data from additional cohorts. By addressing missing features or labels, a novel approach to model training ensured robust data use and maximized sample sizes.
Study findings
Using multimodal data, this study carefully classifies dementia into thirteen diagnostic categories that neurologists have identified and that correspond with therapeutic management paths. Because their care pathways are comparable, dementia from Parkinson’s disease and LBD are placed together under LBD, but cases of VD involve stroke symptoms that are handled by stroke specialists. PSY covers psychiatric disorders such as depression and schizophrenia.
With a microaveraged Area Under the Precision-Recall Curve (AUPR) of 0.90 and an Area Under the Receiver Operating Characteristic Curve (AUROC) of 0.94, the model showed impressive performance on test cases of dementia, MCI, and NC. On datasets from the Framingham Heart Study (FHS) and Alzheimer’s Disease Neuroimaging Initiative (ADNI), it performed better than CatBoost, demonstrating its improved diagnostic accuracy.
Key factors influencing diagnosis decisions were identified by Shapley analysis: memory-related features, functional impairment, and T1-weighted MRI for MCI predictions; cognitive status, lower Mini-Mental State Examination (MMSE) scores, and memory task performance for NC predictions; and functional impairment, lower Mini-Mental State Examination (MMSE) scores, and Apolipoprotein E4 (APOE4) alleles for dementia predictions.
The model proved robust against missing features, retaining consistent scores in the face of imperfect data. Validation on external datasets such as ADNI and FHS demonstrated outstanding performance, with weighted-average AUROC and AUPR scores of 0.91 and 0.86 for ADNI and 0.68 and 0.53 for FHS, respectively, despite considerable missing data.
The model’s usefulness in early disease identification was further evidenced by its constant ability to attribute greater odds of prodromal Alzheimer’s disease (AD) to MCI cases associated with AD. The model’s sensitivity to incremental clinical dementia assessments was demonstrated by a substantial correlation found between comparison with Clinical Dementia Ratings (CDR) scores across the NACC, ADNI, and FHS datasets.
The model showed good diagnostic performance with microaveraged AUROC and AUPR values of 0.96 and 0.70, respectively, across ten different dementia etiologies. Despite the fact that differences in AUPR scores suggested difficulties in recognizing less common or complicated dementias, the model demonstrated strong performance across various demographic categories.
The model demonstrated considerable distinction between biomarker-negative and positive groups when aligning model-predicted probabilities with AD, FTD, and LBD biomarkers, demonstrating its efficacy in capturing the pathophysiology of dementia. The model’s ability to match probability scores with neuropathological indicators was further validated by postmortem data.
The model’s potential to improve clinical dementia diagnosis was demonstrated by the notable gains in diagnostic performance that AI-augmented clinician assessments revealed, with enhanced AUROC and AUPR scores across all categories.
In conclusion
The paper presents an AI model that uses multimodal data to diagnose dementia differentially. It differs from earlier models in that it makes a distinction between different dementia etiologies, including AD, VD, and LBD, which is important for individualized treatment plans. The model’s predictions were validated across a variety of cohorts and supported by postmortem and biomarker data. It was shown that combining model predictions with neurologist assessments could improve diagnostic accuracy, as it performed better than neurologist-only evaluations. By offering likelihood scores for each etiology, the model tackles mixed dementias and enhances clinical decision-making.
For more information: AI-based differential diagnosis of dementia etiologies on multimodal data, Nature Medicine, DOI- https://doi.org/10.1038/s41591-024-03118-z
more recommended stories
New Blood Cancer Model Unveils Drug Resistance
New Lab Model Reveals Gene Mutation.
Osteoarthritis Genetics Study Uncovers New Treatment Hope
Osteoarthritis- the world’s leading cause of.
Antibody Breakthrough in Whooping Cough Vaccine
Whooping cough vaccine development is entering.
Scientists Unveil Next-Gen Eye-Tracking with Unmatched Precision
Eye-tracking technology has long been a.
Men5CV: Hope for Ending Africa’s Meningitis Epidemics
A landmark global health study led.
Stem Cell Therapy Shows 92% Success in Corneal Repair
A groundbreaking stem cell therapy known.
Gene Therapy for Maple Syrup Urine Disease
Researchers at UMass Chan Medical School.
How Fast Are Your Organs Aging? Simple Blood Test May Tell
New research from University College London.
HEALEY Platform Accelerates ALS Therapy Research
A New Era of ALS Clinical.
Low-Oxygen Therapy in a HypoxyStat Pill? Scientists Say It’s Possible
A New Approach to Oxygen Regulation-HypoxyStat.
Leave a Comment