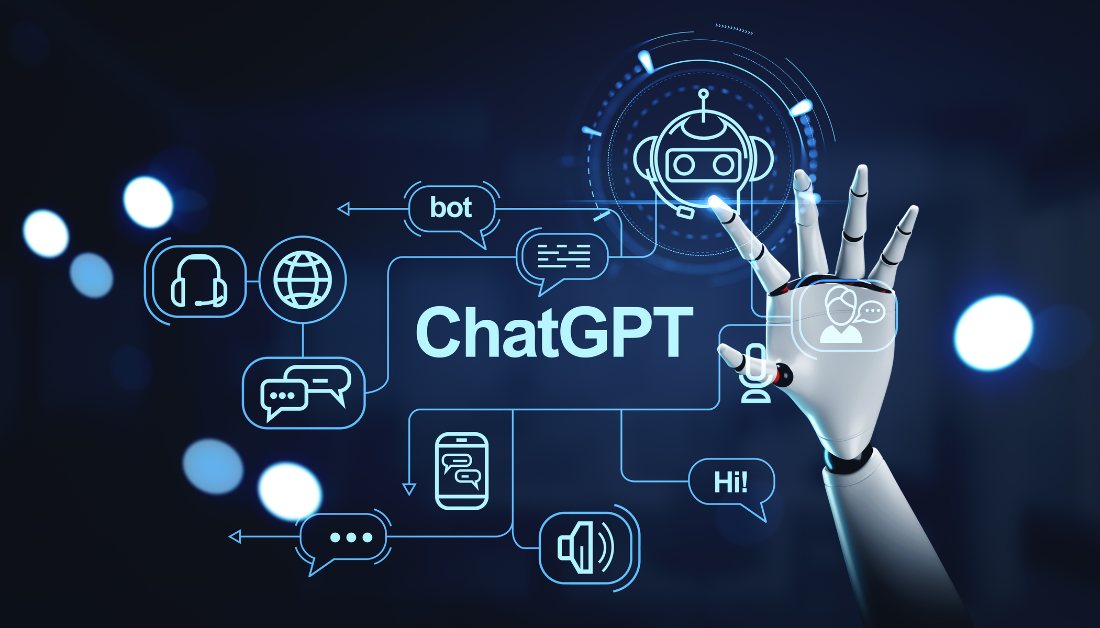
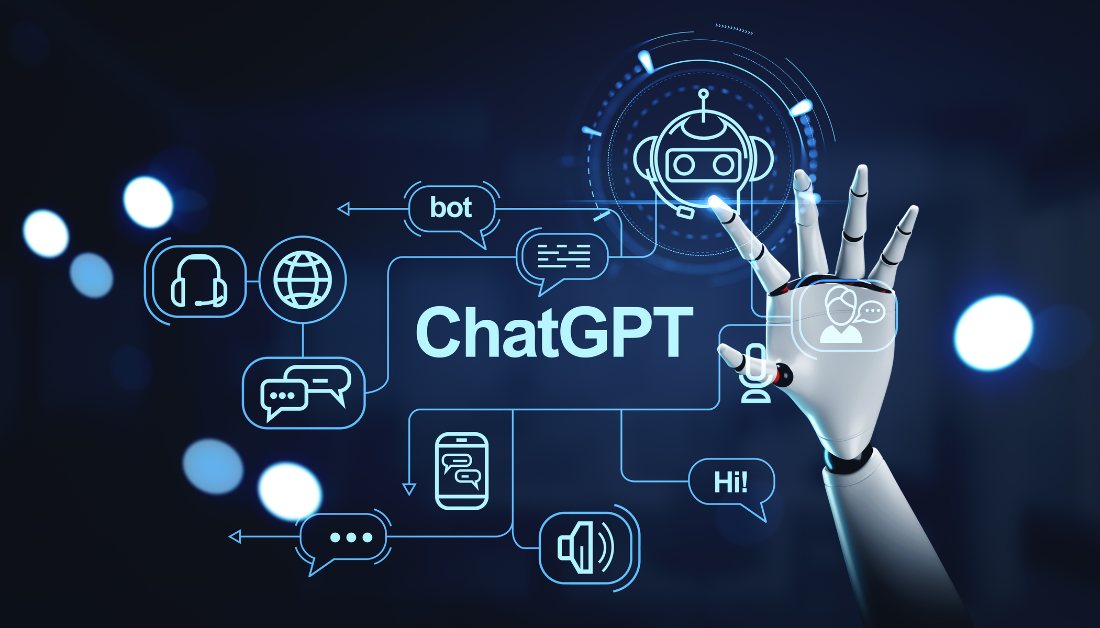
After asking the LLM to assess 100 random case studies, a trio of doctors from Cohen Children’s Medical Center in New York discovered ChatGPT pediatric diagnostic skills to be very poor. Joseph Barile, Alex Margolis, and Grace Cason tested ChatGPT’s diagnostic abilities in their study, which was published in the journal JAMA Pediatrics.
The researchers emphasize that pediatric diagnosis is particularly difficult since, in addition to taking into account all of the symptoms identified in a specific child, age must also be considered. They observed in this new effort that LLMs have been advocated as a viable new diagnostic tool by some in the medical profession. The researchers compiled 100 random pediatric case studies and requested ChatGPT to diagnose them to determine their efficacy.
To keep things simple, the researchers queried the LLM using a same approach for all of the case studies. They pasted in the case study material first, followed by the instruction “List a differential diagnosis and a final diagnosis.”
A differential diagnosis is a method of generating a preliminary diagnosis (or several of them) based on a patient’s history and physical examination. As the name implies, the final diagnosis is the suspected source of the symptoms. Answers provided by the LLM were rated by two non-study colleagues—there were three possible scores: “correct,” “incorrect,” and “did not fully capture diagnosis.”
The researchers discovered that ChatGPT gave the right scores just 17 times, 11 of which were clinically related to the correct diagnosis but were nonetheless incorrect.
The researchers acknowledge that ChatGPT is not yet suitable for use as a diagnostic tool, but they also indicate that more targeted training could improve results. They go on to say that, in the meanwhile, LLMs like ChatGPT could be beneficial as an administrative tool, or to help write research publications or generate instruction sheets for patients to use in aftercare applications.
For more information: Joseph Barile et al, Diagnostic Accuracy of a Large Language Model in Pediatric Case Studies, JAMA Pediatrics (2024). DOI: 10.1001/jamapediatrics.2023.5750
more recommended stories
Caffeine and SIDS: A New Prevention Theory
For the first time in decades,.
Microbial Metabolites Reveal Health Insights
The human body is not just.
Reelin and Cocaine Addiction: A Breakthrough Study
A groundbreaking study from the University.
Preeclampsia and Stroke Risk: Long-Term Effects
Preeclampsia (PE) – a hypertensive disorder.
Statins and Depression: No Added Benefit
What Are Statins Used For? Statins.
Azithromycin Resistance Rises After Mass Treatment
Mass drug administration (MDA) of azithromycin.
Generative AI in Health Campaigns: A Game-Changer
Mass media campaigns have long been.
Molecular Stress in Aging Neurons Explained
As the population ages, scientists are.
Higher BMI and Hypothyroidism Risk Study
A major longitudinal study from Canada.
Therapeutic Plasma Exchange Reduces Biological Age
Therapeutic plasma exchange (TPE), especially when.
Leave a Comment