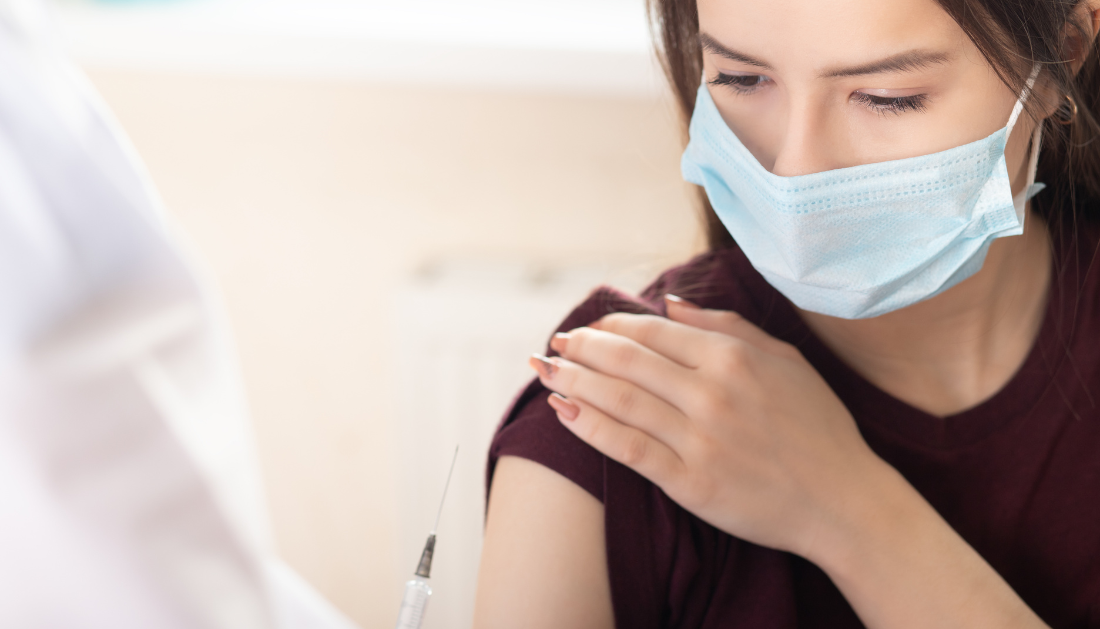
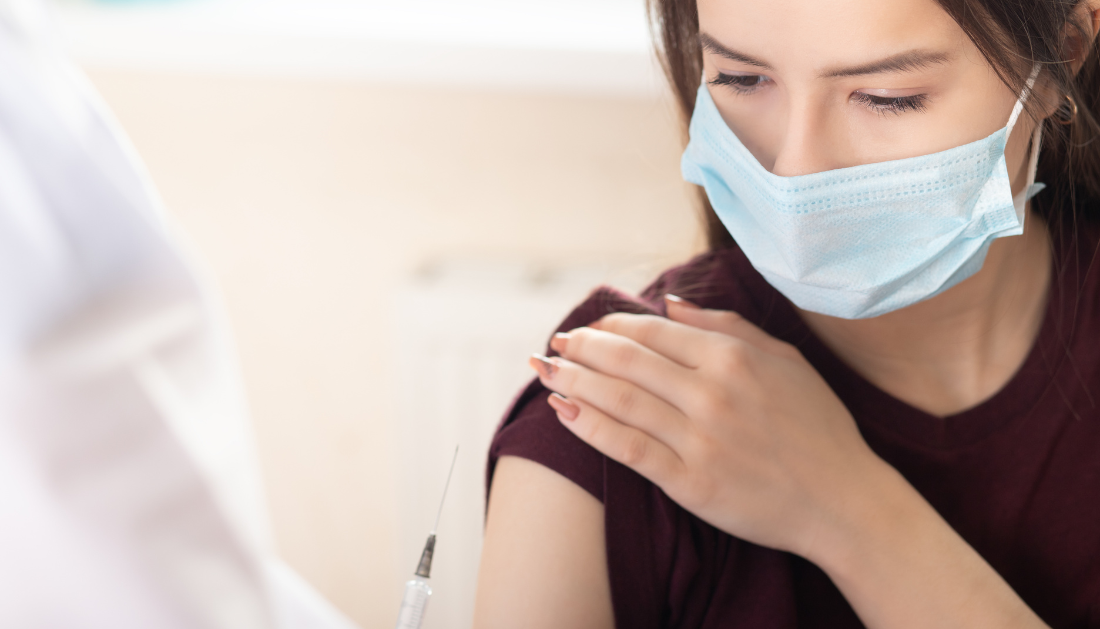
A machine learning algorithm that can precisely forecast how a seasonal flu virus is anticipated to evolve has been created by a group of researchers from the Odum School of Ecology at the University of Georgia. With the use of this data, seasonal flu vaccinations might be updated more promptly, resulting in fewer infections and fatalities.
The research describes a machine-learning method called Novel Influenza Virus A Detector (NIAViD) that predicts changes in the seasonal flu virus with about 73% accuracy. It was published in the journal Proceedings of the Royal Society B: Biological Sciences. NIAViD was developed by Alpha Forna, a postdoctoral research associate in the Odum School. It leverages flu virus sequence data to determine how new viruses are expected to evade a population’s existing immunity.
One billion individuals worldwide are infected by seasonal flu viruses each year, and small children in developing nations account for the majority of the estimated 500,000 fatalities. Every year, millions of Americans get infected in the US, and thousands of them pass away. In the United States alone, the estimated yearlyized cost of seasonal illnesses is close to $90 billion.
Predicting how a flu virus is likely to evolve is one of the difficulties in reducing seasonal flu infections, according to Forna.
“The hemagglutinin protein found on every influenza virus causes an infection when it binds to cells. We refer to these tiny genetic alterations to the hemagglutinin protein as “antigenic drift,” which happens when influenza viruses start to proliferate during an infection,” he explained.
“Some of these changes may make an influenza virus unrecognizable to our immune system. This means that although someone may have had a previous influenza infection or received a vaccine, they might still be infected by an influenza virus that has a slightly evolved hemagglutinin protein.”
In the field of infectious disease modeling research, machine learning—a technique that trains computers to learn on their own—becomes more and more crucial. During the course of two years, Forna built the NIAViD system, gaining access to and interpreting enormous datasets and training models to produce precise forecasts. In order to examine amino acid sequences in a specific area of the hemagglutinin protein’s gene and numerous associated features that measure qualities, such a virus’s electrostatic charge, he and his colleagues devised a unique set of algorithms.
The accuracy rate of the model after it was trained on a subset of data was over 73%, matching or bettering the results of previous models. NIAViD helps with the timely updating of flu vaccinations by accurately identifying antigenic changes by focusing on important features.
The influenza virus that gave rise to NIAViD initially appeared during the 1968 pandemic that originated in Hong Kong. Following the start of this virus’s global spread, research facilities all over the world started analyzing gathered viruses to see how they responded to antibodies that were thought to shield humans from infection. Clustered groups were subsequently recognized as viruses that were immune to both antibodies and vaccines; this led to early predictive modeling that assisted vaccine manufacturers in concentrating their efforts on emerging influenza lineages.
These early studies, according to co-author and professor in UGA’s College of Veterinary Medicine Justin Bahl, were crucial in the development of vaccines.
“Clustering influenza viruses into specific groups to understand their level of immunity to vaccines was groundbreaking,” he said. “However, this research was based on a virus surveillance methodology that is labor-intensive, time-consuming, and can be unreliable. We believed that a faster and more accurate method of predicting emerging seasonal influenza viruses could be accomplished using an infectious disease machine-learning algorithm.”
John Drake, a professor at Regents University and the head of the Center for the Ecology of Infectious Diseases, says that the success of NIAViD provides many opportunities for future research.
“Its ability to rapidly identify antigenic variants can support the ongoing development of flu vaccines, bolstering public health readiness and responsiveness to seasonal flu,” he said. “Integrating NIAViD into surveillance systems would allow manufacturers to stay ahead of viral evolution, ensuring vaccines target the most current strains.”
For more information: Sequence-based detection of emerging antigenically novel influenza A viruses, Proceedings of the Royal Society B, https://dx.doi.org/10.1098/rspb.2024.0790
more recommended stories
Harmful Chemicals in Children’s Mattresses
A recent study has brought to.
TMS for Post-Stroke Aphasia Shows Remarkable Gains
A new clinical study led by.
CT Scan Overuse May Cause 1 in 20 New U.S. Cancers
A recent study published in JAMA.
Lactation Metabolism: Brain-Hormone Link Uncovered
Understanding how the body adjusts to.
Quantum Technology in Cancer Surgery: New Probe Aims to Improve Outcomes
A groundbreaking project from the University.
Bean-Based Gum Offers New Approach to Combat Influenza and Herpes
In an era where infectious diseases.
Shingles Vaccine May Cut Dementia Risk by 20%
A new study shows that the.
New Study Questions Fluid Restriction in Heart Failure Management
A groundbreaking study presented at the.
Role of Leptin Signaling in the DMH for Metabolic Regulation
A groundbreaking study from the Pennington.
COVID-19 Vaccines May Lower the Risk of Long COVID by 27%
A recent rapid review suggests that.
Leave a Comment