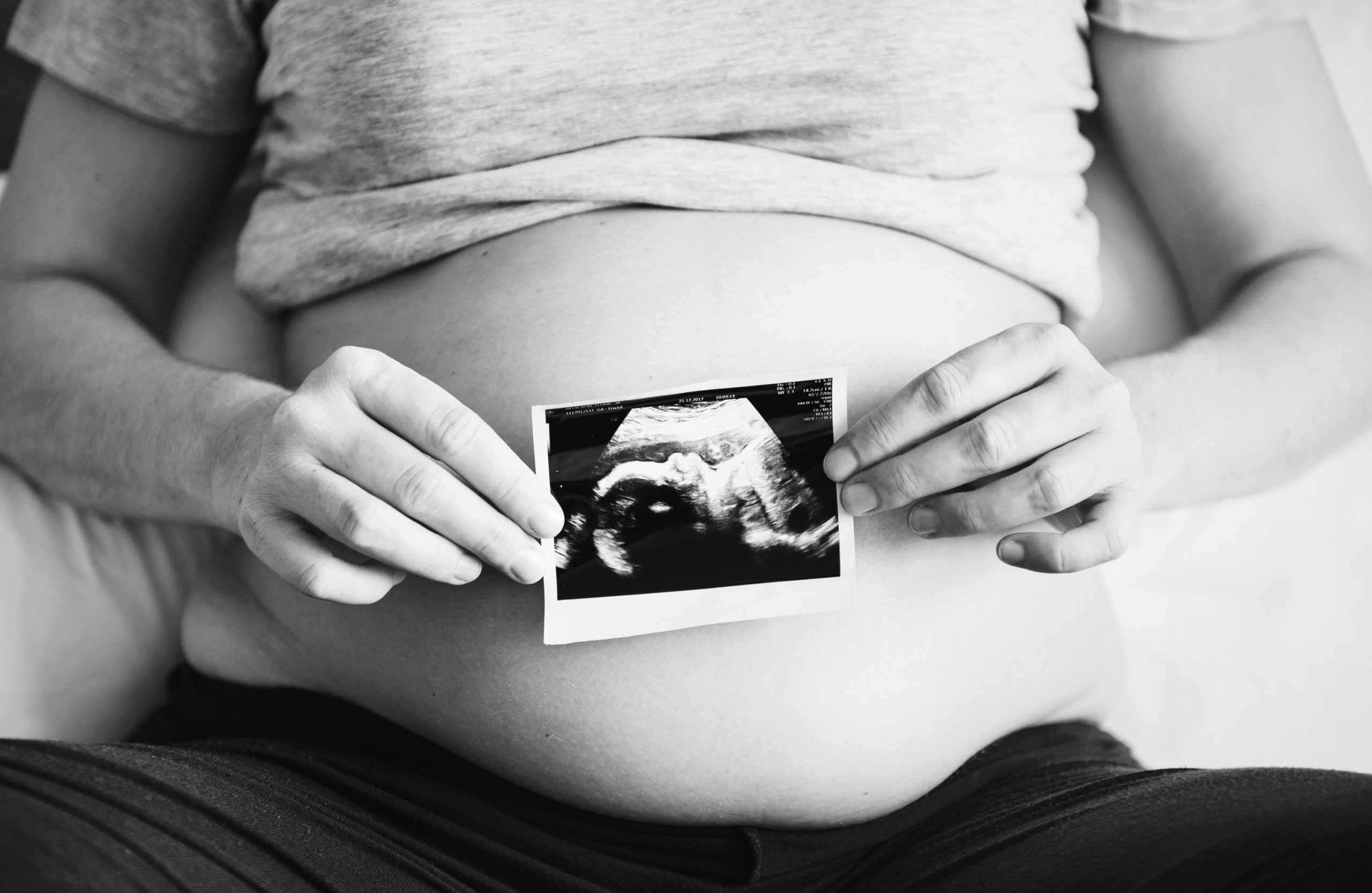
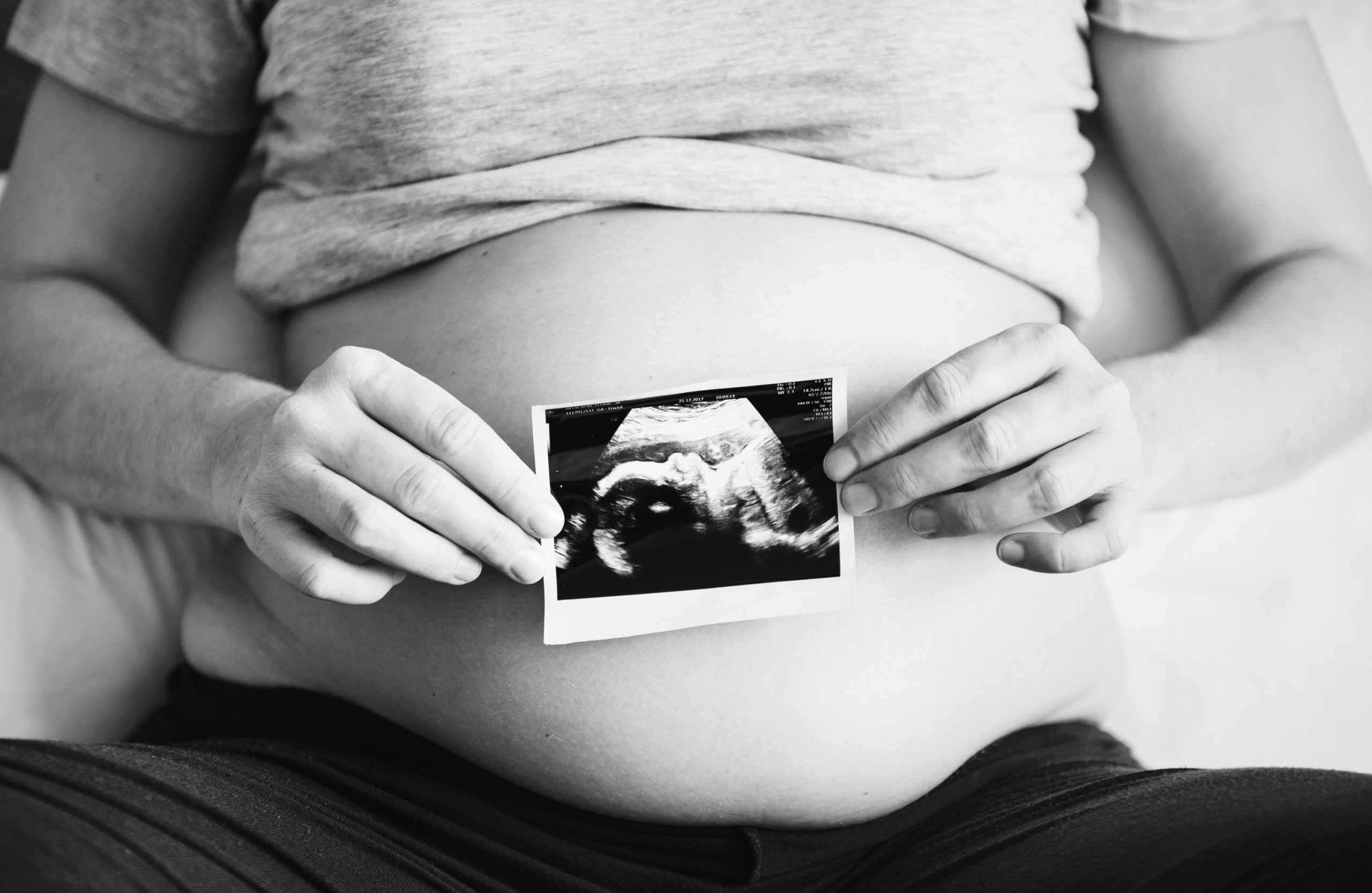
Preterm birth, defined as a baby being born before 37 weeks of gestation, affects almost 10% of pregnancies globally, and rates are rising. Researchers at Washington University in St. Louis’ McKelvey School of Engineering are developing improved methods to predict preterm birth by studying electrical activity during pregnancy.
Arye Nehorai, the Eugene & Martha Lohman Professor of Electrical Engineering in the Preston M. Green Department of Electrical & Systems Engineering, and Uri Goldsztejn, who will graduate with master’s and doctorate degrees in biomedical engineering from Washington University in 2020 and 2022, respectively, created a deep learning model to predict preterm births as early as 31 weeks of pregnancy. The study’s findings were published in the journal PLoS One on May 11.
“Our method predicts preterm births using electrohysterogram measurements and clinical information acquired around the 31st week of gestation with a performance comparable to the clinical standards used to detect imminent labor in women with symptoms of preterm labor,” Nehorai said.
Nehorai and Goldsztejn used electrohysterograms (EHG), a noninvasive technique that detects uterine electrical activity through electrodes placed on the abdomen, as well as clinical data from two public databases, such as age, gestational age, weight, and bleeding in the first or second trimester, to design their method.
They constructed a deep learning model on data from 30-minute EHGs done on 159 pregnant women aged at least 26 weeks. Some recordings were taken during routine check-ups, whereas others were received from moms who were hospitalized with preterm labor signs. Nearly 19% of all mothers delivered prematurely.
“We predicted the pregnancies’ outcomes from EHG recordings using a deep neural network, because neural networks automatically learn the most informative features from the data,” Goldsztejn said. “The deep learning algorithm achieved a better performance than other methods and provided a good way to combine EHG data with clinical information.”
The researchers trained their deep recurrent neural network on data samples that showed their pregnancy result in order to discover aspects from the data that predicted such outcomes.
The study builds on prior work from Nehorai’s team that was published in PLoS One and is the first to predict preterm deliveries as early as 31 weeks using EHG readings. In a previous work, Nehorai and his colleagues used magnetomyography to estimate electrical current in the uterus during contractions. Magnetomyography is a noninvasive technique that maps muscle activity by monitoring the abdominal magnetic fields produced by electrical currents in muscles.
It also builds on new research by Nehorai and Goldsztejn, which was recently published in Biomedical Signal Processing and Control, and describes a statistical signal processing method for distinguishing uterine electrical activity from baseline electrical activity, such as the woman’s heart, in multidimensional EHG measurements in order to more precisely identify uterine contractions.
Nehorai and Goldsztejn discovered that several components of EHG data contributed to the model’s predictions in their study. Higher frequency components of the EHG readings predicted preterm births better. They also discovered that their model was excellent at predicting with shorter EHG recordings, which could make the model easier to use, more cost-effective in a clinical context, and potentially useable at home.
“Preterm birth is an abnormal physiological condition, not just a pregnancy that happened to end early,” Nehorai said. “Therefore, we can expect that physiological measurements, such as EHG recordings, may show a stronger dichotomy between pregnancies that end with either preterm or term deliveries than is shown in continuous characteristics correlated with gestational age at delivery.”
Nehorai and Goldsztejn intend to design a device to record EHG readings and collect data from a bigger cohort of pregnant women in order to enhance their procedure and validate results in the future.
more recommended stories
lncRNAs in Tumor Microenvironment Drive Cancer Progression
The tumor microenvironment (TME) is a.
Lyme Disease Symptoms: Why They Linger After Treatment
Lyme disease symptoms that persist after.
Body Image in Young Women: Cultural Influences Matter
A new international study led by.
Early Alzheimer’s Found Through Blood Biomarkers
A new study published in Molecular.
Label-Free Motion Analysis Detects Cancer Cells
Label-free motion analysis is emerging as.
Harmful Chemicals in Children’s Mattresses
A recent study has brought to.
TMS for Post-Stroke Aphasia Shows Remarkable Gains
A new clinical study led by.
CT Scan Overuse May Cause 1 in 20 New U.S. Cancers
A recent study published in JAMA.
Lactation Metabolism: Brain-Hormone Link Uncovered
Understanding how the body adjusts to.
Quantum Technology in Cancer Surgery: New Probe Aims to Improve Outcomes
A groundbreaking project from the University.
Leave a Comment